Tissue Classification Using Landmark And Non-Landmark Gene Sets For Feature Selection
BIG DATA, IOT, AND AI FOR A SMARTER FUTURE(2021)
摘要
The L1000 dataset, containing gene microarray data from 978 landmark genes has been previously shown to accurately predict expression of similar to 81% of the remaining 21,290 target genes. Microarray data was utilized to characterize groups of tissue types within the L1000 dataset to assess whether 978 landmark genes, compared to non-landmark genes, would better differentiate samples into clusters containing distinct tissue types. Landmark genes better differentiated k-means clusters, compared to non-landmark genes. These results suggest that landmark genes better characterize heterogeneous samples in their comprehensive genetic profile. Our previous studies showed that categorical separation of samples based on clinical or biological groups generally improves when studying heterogeneous sample types when using landmark genes as features, compared to non-landmark genes. However, the present work indicates that non-landmark genes may also be utilized to separate samples in clustering when there is a large sample size present for training k-means clustering models. In contrast, when studying a small sample size of the same set of heterogenous samples, landmark genes as features improve clustering. This study has implications for assessing various tissue types as landmark genes may be directly measured to predict categorical sample qualities as well as expression of remaining target genes. (c) 2021 The Authors. Published by Elsevier B.V. This is an open access article under the CC BY-NC-ND license (https://creativecommons.org/licenses/by-nc-nd/4.0) Peer-review under responsibility of the scientific committee of the Complex Adaptive Systems Conference, June 2021.
更多查看译文
关键词
Landmark Genes, L1000, Microarray, K-Means Clustering, Dimensionality Reduction, Feature Selection, Principal Components Analysis, Tissue Classification
AI 理解论文
溯源树
样例
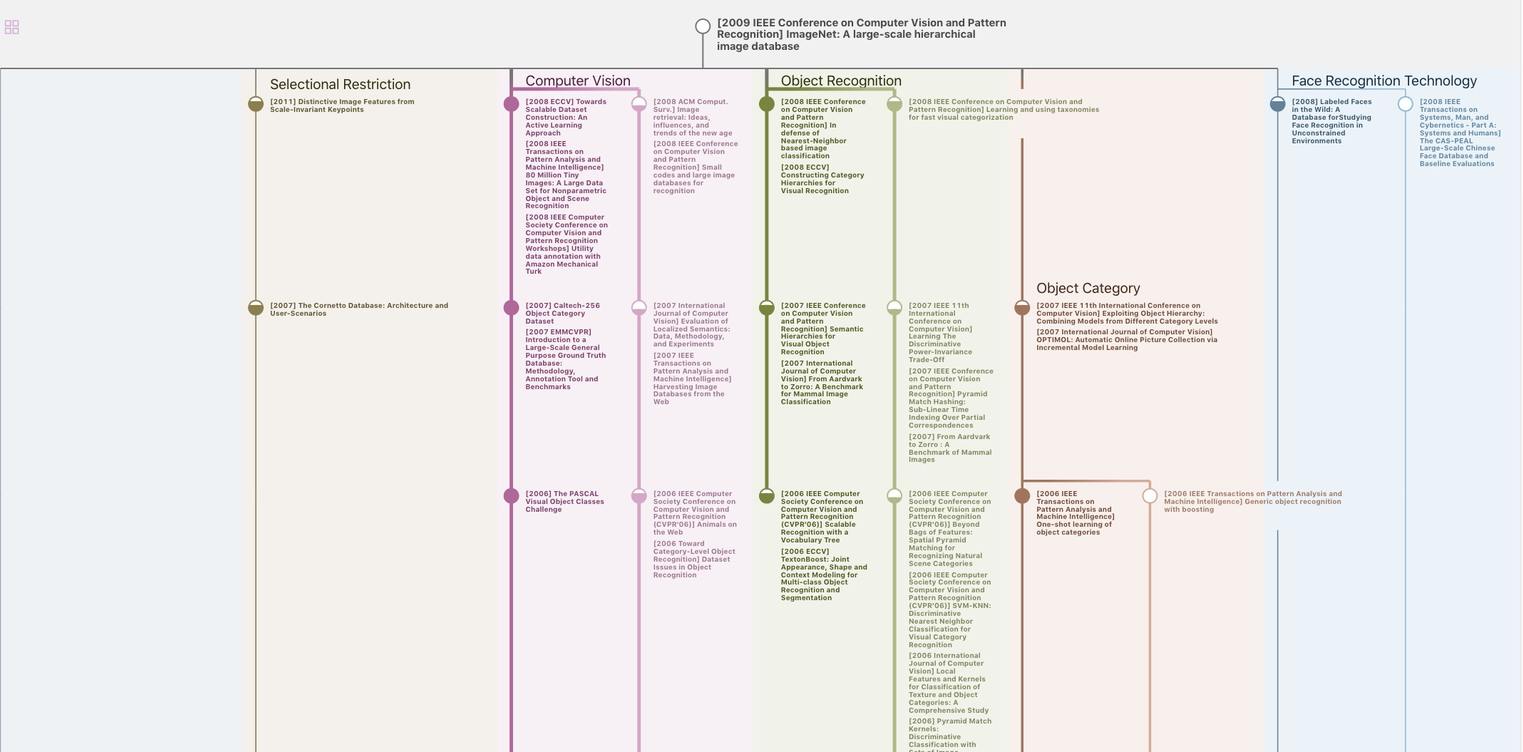
生成溯源树,研究论文发展脉络
Chat Paper
正在生成论文摘要