Gaussian process surrogate model for an effective life assessment of transformer considering model and measurement uncertainties
International Journal of Electrical Power & Energy Systems(2022)
摘要
The challenges encountered because of simplifications, assumptions, and limited information in the transformer thermal modeling affect the entire life assessment process of the transformer. The classical techniques such as the gradient estimator and the Levenberg-Marquardt (LM) method for parameter estimation of the existing thermal models result in incorrect gradient and Hessian calculation due to measurement noise. Such limitations lead to model and measurement uncertainties that fail to achieve effective online monitoring for system diagnosis, determination of overload capability, and ageing rate. A systematic approach relying on the black-box model and Design of experiment (DoE) is proposed to build a surrogate model for Top-oil Temperature (TOT) prediction and parameter estimation. The Hot spot Temperature (HST) and Loss-of-Life (LoL) are evaluated under uncertainties with sequential use of the Gaussian Process (GP) surrogate model and an existing thermal-electrical-based thermal model (white-box model) of a transformer. An in-service transformer data and the virtual data generated in MATLAB are used to authenticate the proposed method's accuracy and effectiveness in the presence of uncertainties.
更多查看译文
关键词
Design of Experiment (DoE),Gaussian Process Regression (GPR),Hot spot Temperature (HST),Loss of Life (LoL),Top-oil Temperature (TOT)
AI 理解论文
溯源树
样例
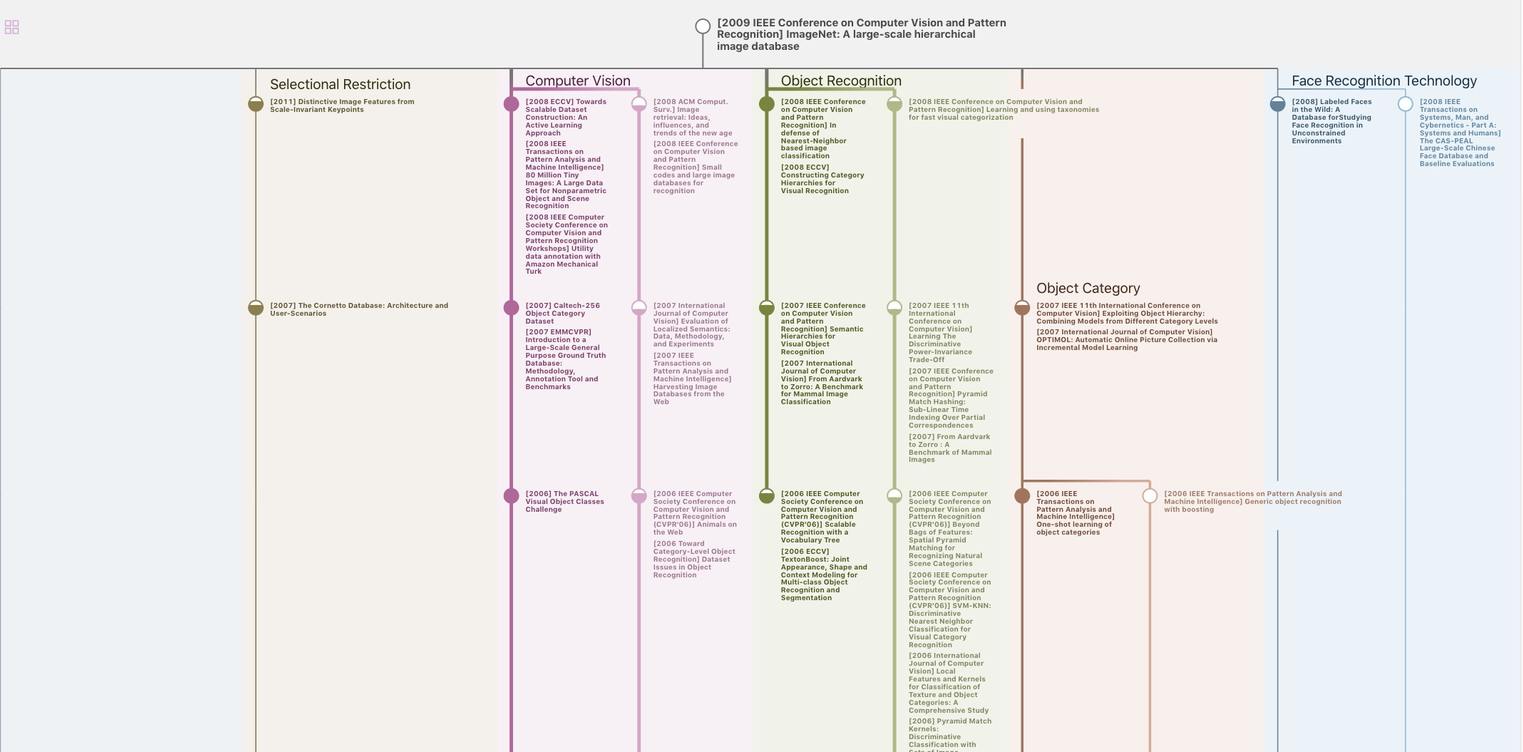
生成溯源树,研究论文发展脉络
Chat Paper
正在生成论文摘要