Generalization Capability Of Convolutional Neural Networks For Progress Variable Variance And Reaction Rate Subgrid-Scale Modeling
ENERGIES(2021)
摘要
Deep learning has recently emerged as a successful approach to produce accurate subgrid-scale (SGS) models for Large Eddy Simulations (LES) in combustion. However, the ability of these models to generalize to configurations far from their training distribution is still mainly unexplored, thus impeding their application to practical configurations. In this work, a convolutional neural network (CNN) model for the progress-variable SGS variance field is trained on a canonical premixed turbulent flame and evaluated a priori on a significantly more complex slot burner jet flame. Despite the extensive differences between the two configurations, the CNN generalizes well and outperforms existing algebraic models. Conditions for this successful generalization are discussed, including the effect of the filter size and flame-turbulence interaction parameters. The CNN is then integrated into an analytical reaction rate closure relying on a single-step chemical source term formulation and a presumed beta PDF (probability density function) approach. The proposed closure is able to accurately recover filtered reaction rate values on both training and generalization flames.
更多查看译文
关键词
large eddy simulation, turbulent combustion, deep learning, convolutional neural network, progress variable variance, generalization
AI 理解论文
溯源树
样例
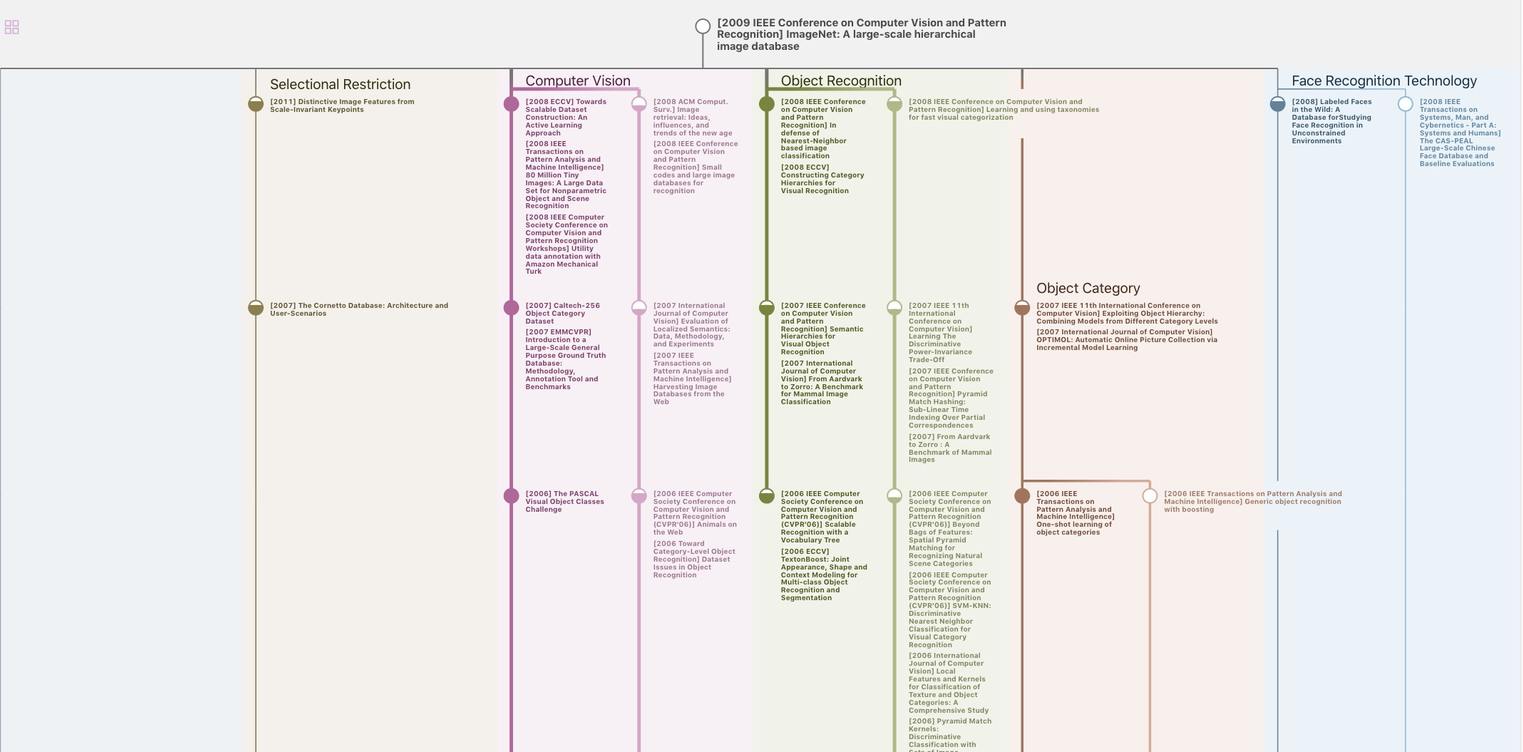
生成溯源树,研究论文发展脉络
Chat Paper
正在生成论文摘要