A machine learning framework for damage mechanism identification from acoustic emissions in unidirectional SiC/SiC composites
NPJ COMPUTATIONAL MATERIALS(2021)
摘要
In this work, we demonstrate that damage mechanism identification from acoustic emission (AE) signals generated in minicomposites with elastically similar constituents is possible. AE waveforms were generated by SiC/SiC ceramic matrix minicomposites (CMCs) loaded under uniaxial tension and recorded by four sensors (two models with each model placed at two ends). Signals were encoded with a modified partial power scheme and subsequently partitioned through spectral clustering. Matrix cracking and fiber failure were identified based on the frequency information contained in the AE event they produced, despite the similar constituent elastic properties of the matrix and fiber. Importantly, the resultant identification of AE events closely followed CMC damage chronology, wherein early matrix cracking is later followed by fiber breaks, even though the approach is fully domain-knowledge agnostic. Additionally, the partitions were highly precise across both the model and location of the sensors, and the partitioning was repeatable. The presented approach is promising for CMCs and other composite systems with elastically similar constituents.
更多查看译文
关键词
Characterization and analytical techniques,Composites,Computational methods,Materials Science,general,Characterization and Evaluation of Materials,Mathematical and Computational Engineering,Theoretical,Mathematical and Computational Physics,Computational Intelligence,Mathematical Modeling and Industrial Mathematics
AI 理解论文
溯源树
样例
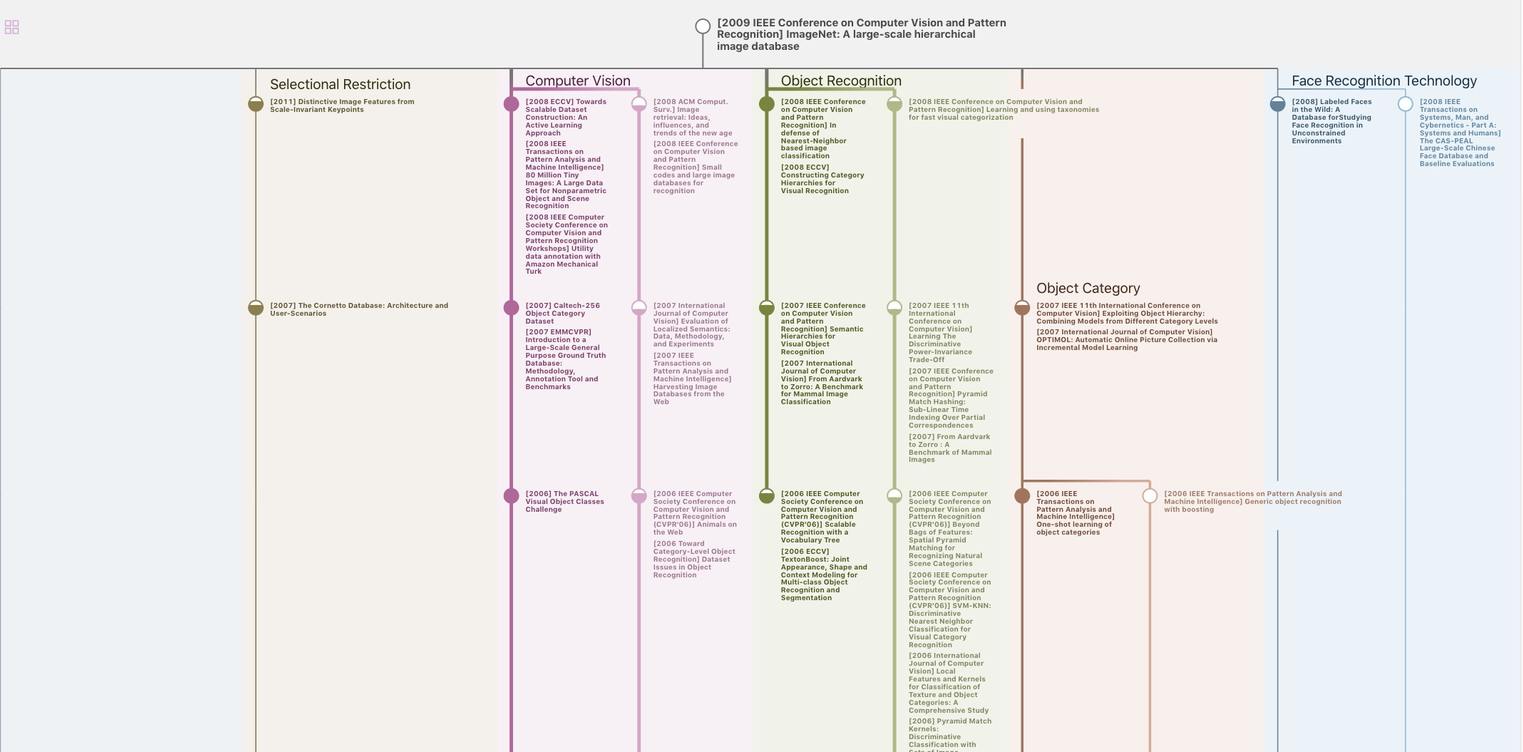
生成溯源树,研究论文发展脉络
Chat Paper
正在生成论文摘要