Source-free unsupervised multi-source domain adaptation via proxy task for person re-identification
The Visual Computer(2021)
摘要
Most of existing unsupervised domain adaptation methods focus on aligning the feature discrepancy between labeled source and unlabeled target data. However, in practice, the source data may not be accessible due to transfer issue, privacy problem, etc. In such case, transferring knowledge through only the trained source model without the labeled source data remains a challenging problem. In this paper, we propose a novel method for source-free (without accessing any source domain data) multi-source domain adaptation in person re-identification (Re-ID). Two proxy tasks including proxy label learning and domain discriminative learning are designed to transfer knowledge from source models to the target domain with the inspiration of self-supervised learning. In the proxy label learning process, a subset of the unlabeled data in the target domain is randomly selected to trained a proxy label generator for measuring the similarity between each sample and the selected subset. With the proxy label as input, in the domain discriminative learning process, a domain discriminator is learnt to assign weights for measuring similarity between each source domain and the target one. With the combination of these two proxy tasks, knowledge from multiple source models can be properly aggregated and adaptively transferred to the target domain without any source data. Extensive evaluations on benchmark datasets, which are DukeMTMC and Market-1501, demonstrate the superior performance of the proposed method.
更多查看译文
关键词
Multi-source domain adaptation, Person re-identification, Transfer knowledge
AI 理解论文
溯源树
样例
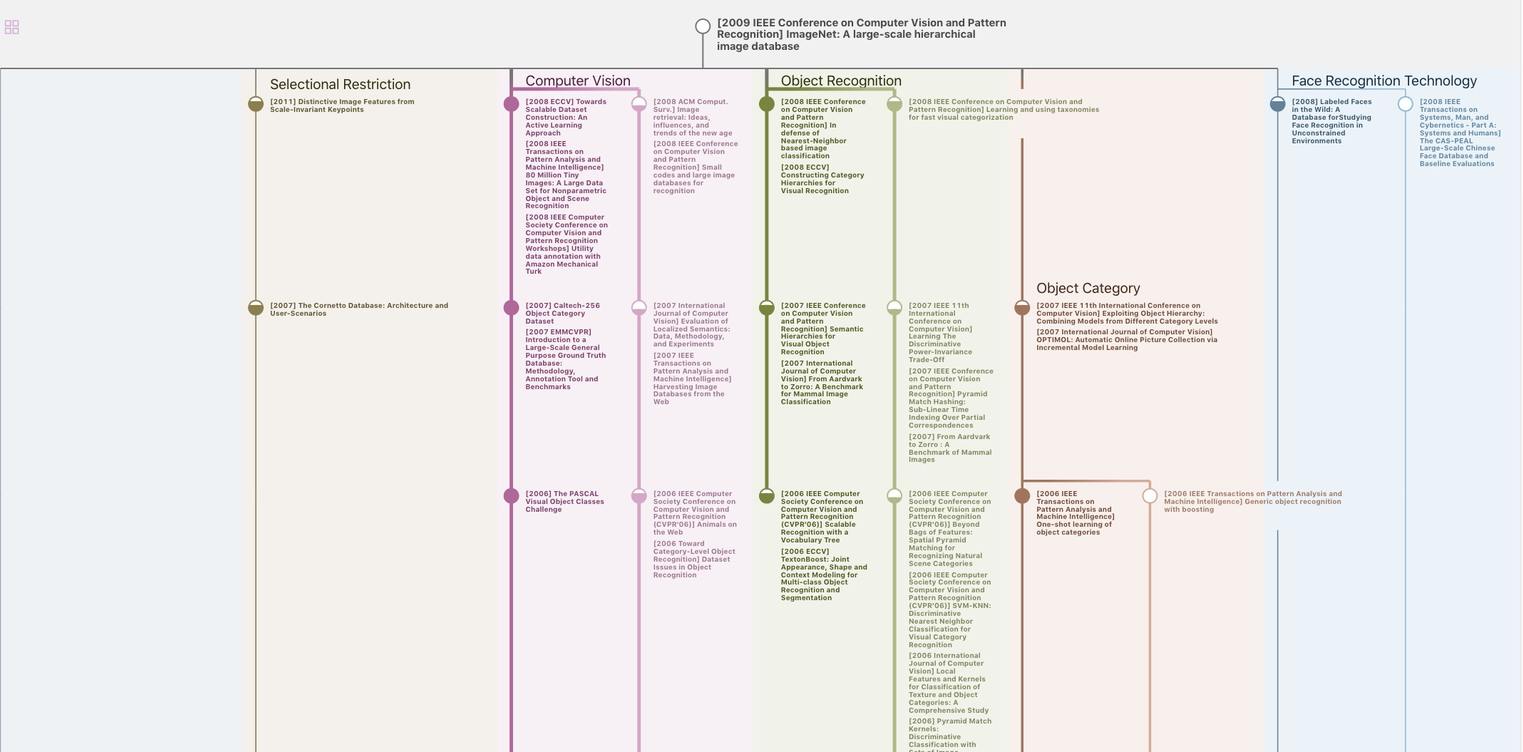
生成溯源树,研究论文发展脉络
Chat Paper
正在生成论文摘要