Federated Learning with Domain Generalization
arxiv(2023)
摘要
Federated Learning (FL) enables a group of clients to jointly train a machine learning model with the help of a centralized server. Clients do not need to submit their local data to the server during training, and hence the local training data of clients is protected. In FL, distributed clients collect their local data independently, so the dataset of each client may naturally form a distinct source domain. In practice, the model trained over multiple source domains may have poor generalization performance on unseen target domains. To address this issue, we propose FedADG to equip federated learning with domain generalization capability. FedADG employs the federated adversarial learning approach to measure and align the distributions among different source domains via matching each distribution to a reference distribution. The reference distribution is adaptively generated (by accommodating all source domains) to minimize the domain shift distance during alignment. In FedADG, the alignment is fine-grained since each class is aligned independently. In this way, the learned feature representation is supposed to be universal, so it can generalize well on the unseen domains. Intensive experiments on various datasets demonstrate that FedADG has comparable performance with the state-of-the-art.
更多查看译文
关键词
federated learning,domain
AI 理解论文
溯源树
样例
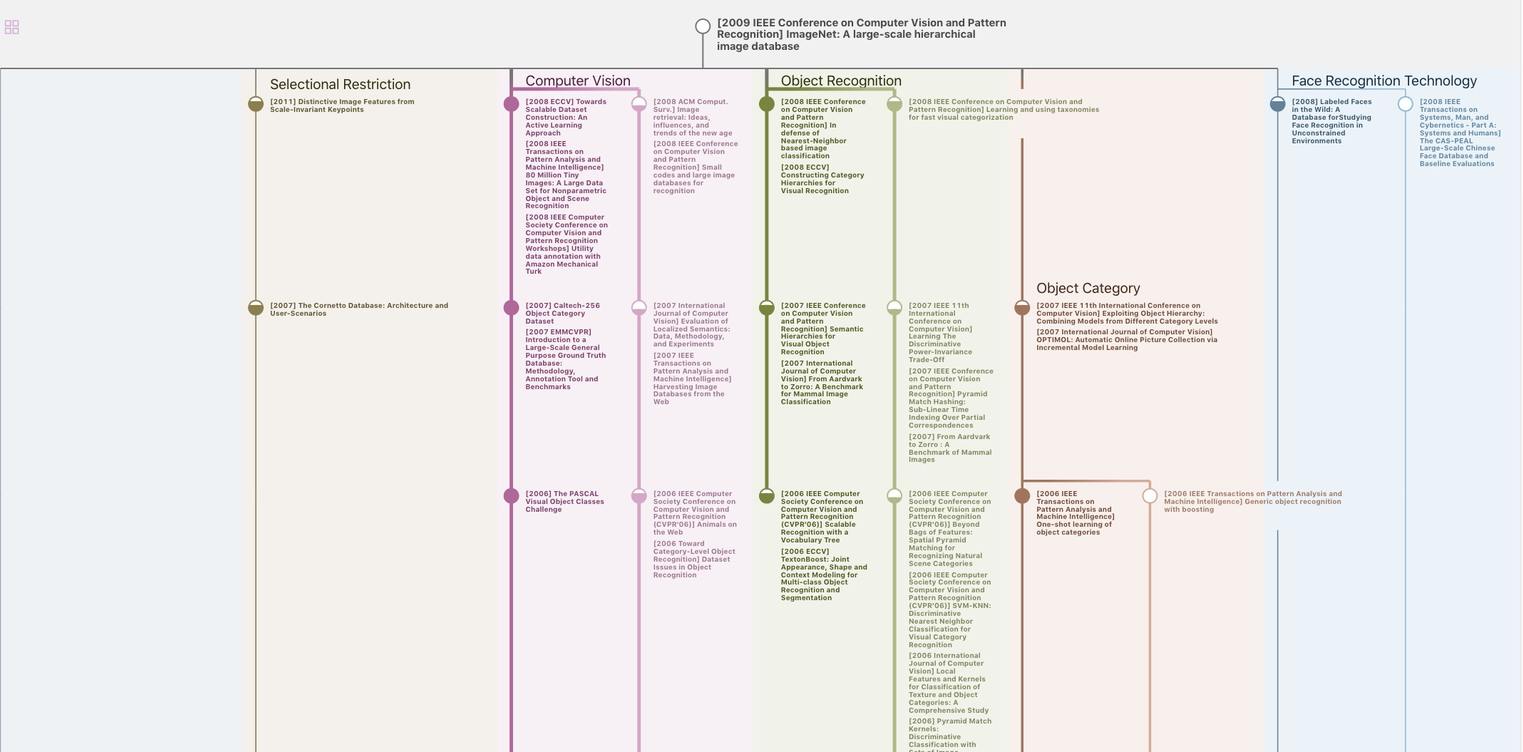
生成溯源树,研究论文发展脉络
Chat Paper
正在生成论文摘要