Reinforcement learning for few-shot text generation adaptation
NEUROCOMPUTING(2023)
摘要
This paper proposes a novel method based on reinforcement learning (RL) to control the generation model in adapting to new domains with limited samples. To avoid the problem of overfitting, the method combines maximum likelihood estimation (MLE) with RL process to improve the sample utilization rate and reduce the sample requirement of RL. The training process is divided into two parts: pre-training and fine-tuning, to effectively express the semantic of the target domain. In order to ensure the robustness of the reward function, adversarial training is introduced. A new measurement called "Net Accuracy"is proposed to better evaluate the domain relevance of the generated text and eliminate the problem of inaccurate domain relevance measurement caused by overfitting and generating a large amount of duplicate text. Finally, experimental results show the effectiveness and superiority of the proposed method in five target domains.
更多查看译文
关键词
Text generation,Domain adaption,Few-shot learning,Reinforcement learning
AI 理解论文
溯源树
样例
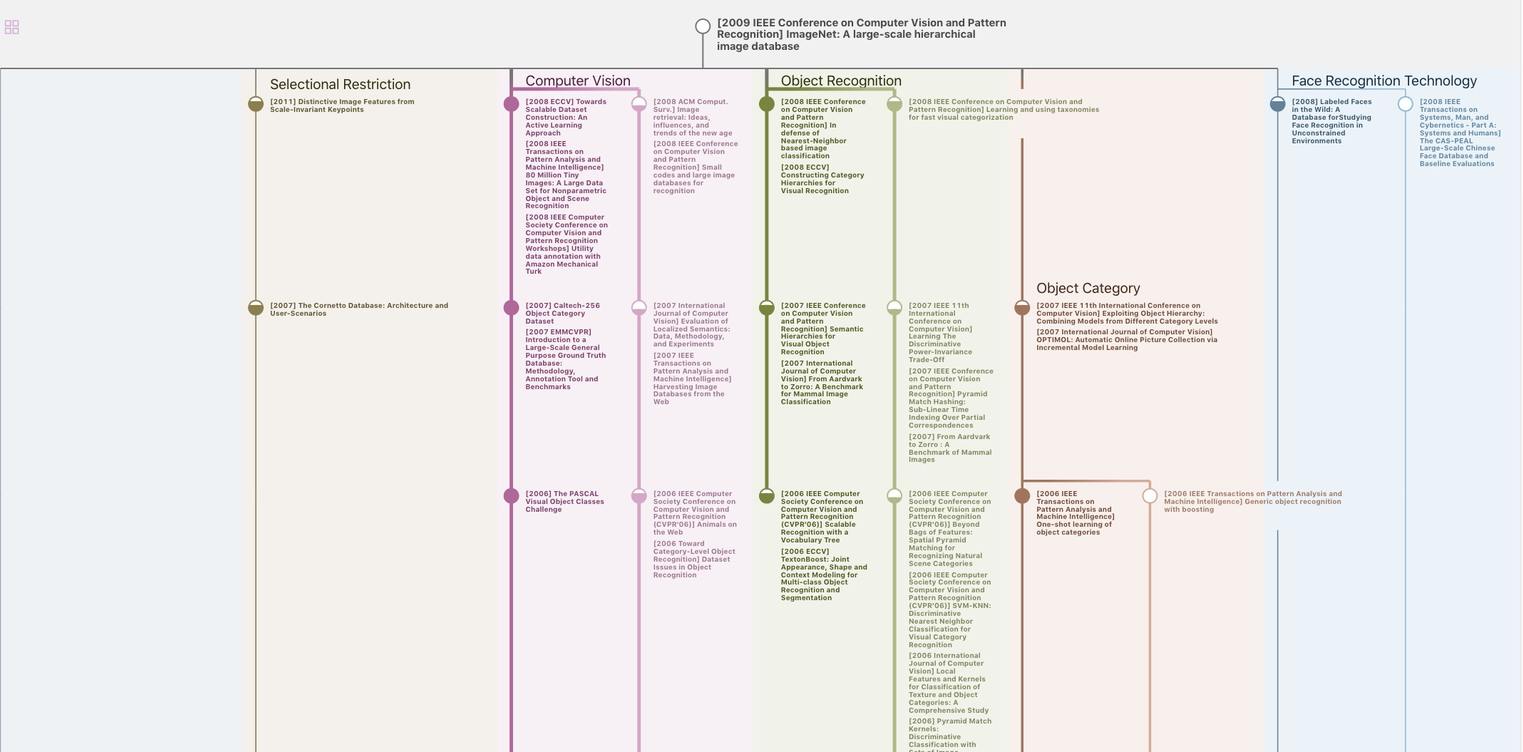
生成溯源树,研究论文发展脉络
Chat Paper
正在生成论文摘要