IMPROVED MODEL BASED DEEP LEARNING USING MONOTONE OPERATOR LEARNING (MOL)
2022 IEEE INTERNATIONAL SYMPOSIUM ON BIOMEDICAL IMAGING (IEEE ISBI 2022)(2022)
摘要
Model-based deep learning (MoDL) algorithms that rely on unrolling are emerging as powerful tools for image recovery. In this work, we introduce a novel monotone operator learning framework to overcome some of the challenges associated with current unrolled frameworks, including high memory cost, lack of guarantees on robustness to perturbations, and low interpretability. Unlike current unrolled architectures that use finite number of iterations, we use the deep equilibrium (DEQ) framework to iterate the algorithm to convergence and to evaluate the gradient of the convolutional neural network blocks using Jacobian iterations. This approach significantly reduces the memory demand, facilitating the extension of MoDL algorithms to high dimensional problems. We constrain the CNN to be a monotone operator, which allows us to introduce algorithms with guaranteed convergence properties and robustness guarantees. We demonstrate the utility of the proposed scheme in the context of parallel MRI.
更多查看译文
关键词
MoDL algorithms,high dimensional problems,guaranteed convergence properties,robustness guarantees,model-based deep learning algorithms,image recovery,novel monotone operator,current unrolled frameworks,high memory cost,current unrolled architectures,deep equilibrium framework,convolutional neural network blocks,Jacobian iterations
AI 理解论文
溯源树
样例
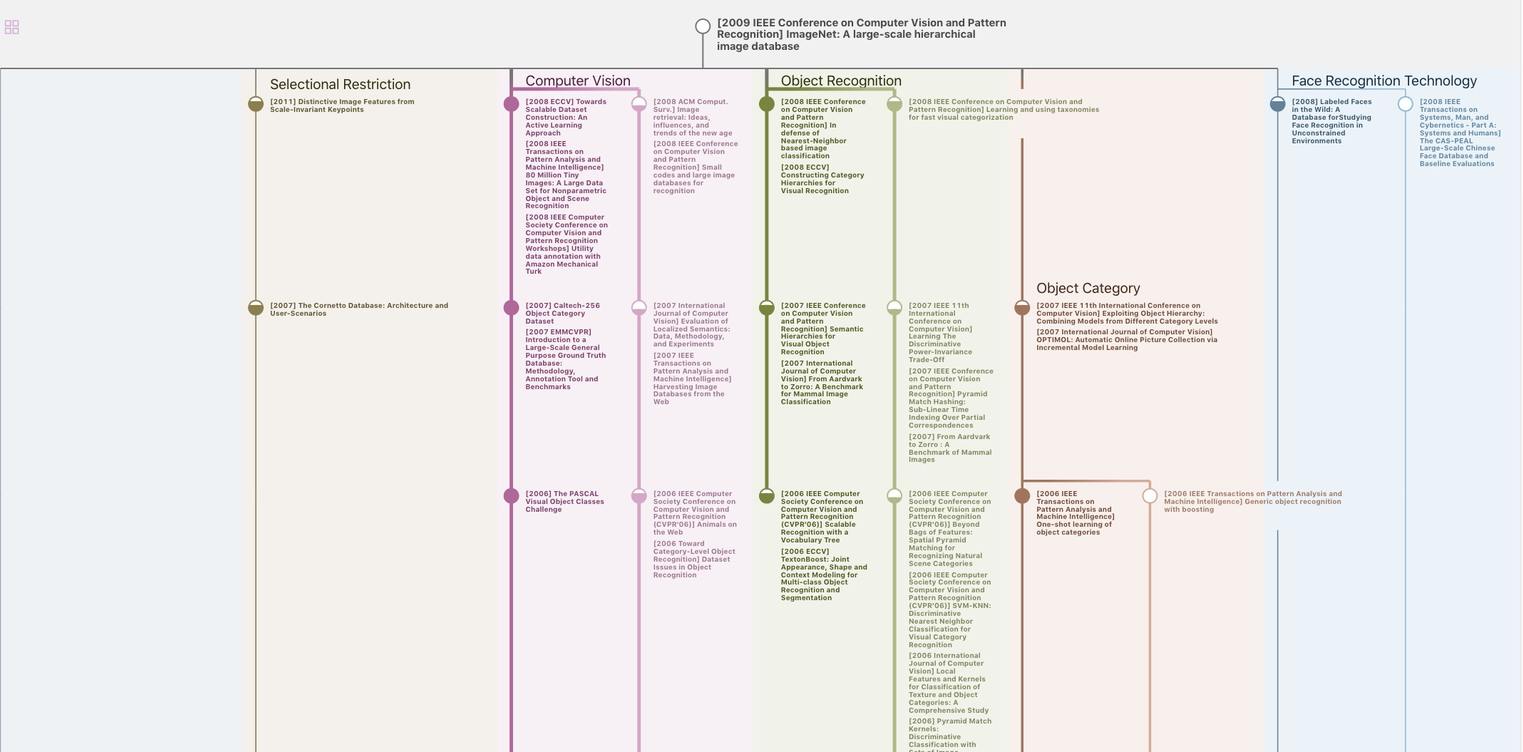
生成溯源树,研究论文发展脉络
Chat Paper
正在生成论文摘要