Attentive Transfer Learning Via Self-Supervised Learning For Cervical Dysplasia Diagnosis
JOURNAL OF INFORMATION PROCESSING SYSTEMS(2021)
摘要
Many deep learning approaches have been studied for image classification in computer vision. However, there are not enough data to generate accurate models in medical fields, and many datasets are not annotated. This study presents a new method that can use both unlabeled and labeled data. The proposed method is applied to classify cervix images into normal versus cancerous, and we demonstrate the results. First, we use a patch self-supervised learning for training the global context of the image using an unlabeled image dataset. Second, we generate a classifier model by using the transferred knowledge from self-supervised learning. We also apply attention learning to capture the local features of the image. The combined method provides better performance than state-of-the-art approaches in accuracy and sensitivity.
更多查看译文
关键词
Attention Learning, Cervical Dysplasia, Patch self-supervised Learning, Transfer Learning
AI 理解论文
溯源树
样例
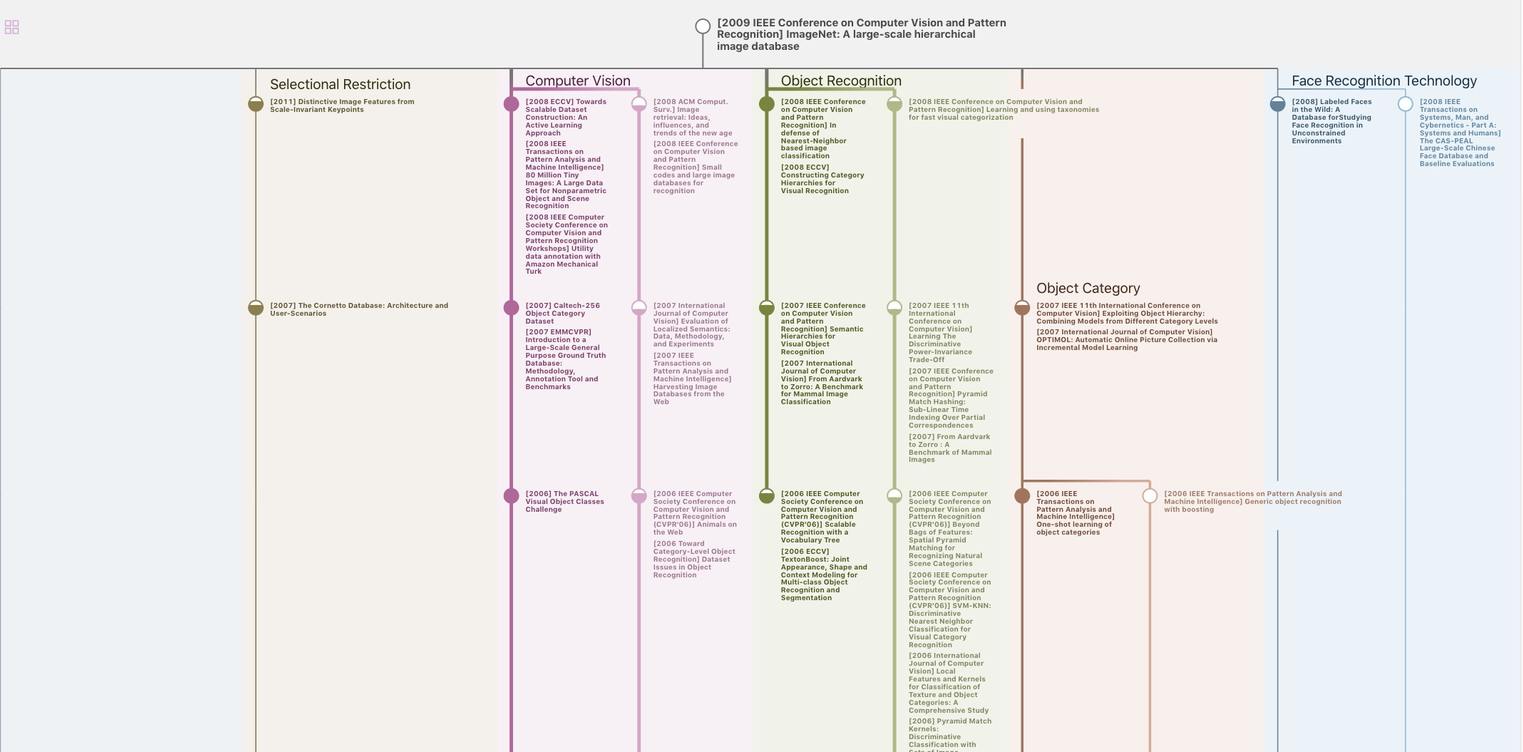
生成溯源树,研究论文发展脉络
Chat Paper
正在生成论文摘要