Towards Quantum Advantage in Financial Market Risk using Quantum Gradient Algorithms
QUANTUM(2022)
摘要
We introduce a quantum algorithm to compute the market risk of financial derivatives. Previous work has shown that quantum amplitude estimation can accelerate derivative pricing quadratically in the target error and we extend this to a quadratic error scaling advantage in market risk computation. We show that employing quantum gradient estimation algorithms can deliver a further quadratic advantage in the number of the associated market sensitivities, usually called greeks. By numerically simulating the quantum gradient estimation algorithms on financial derivatives of practical interest, we demonstrate that not only can we successfully estimate the greeks in the examples studied, but that the resource requirements can be significantly lower in practice than what is expected by theoretical complexity bounds. This additional advantage in the computation of financial market risk lowers the estimated logical clock rate required for financial quantum advantage from Chakrabarti et al. [Quantum 5, 463 (2021)] by a factor of similar to 7, from 50MHz to 7MHz, even for a modest number of greeks by industry standards (four). Moreover, we show that if we have access to enough resources, the quantum algorithm can be parallelized across up to 60 QPUs, in which case the logical clock rate of each device required to achieve the same overall runtime as the serial execution would be similar to 100 kHz. Throughout this work, we summarize and compare several different combinations of quantum and classical approaches that could be used for computing the market risk of financial derivatives.
更多查看译文
关键词
quantum advantage,financial market risk
AI 理解论文
溯源树
样例
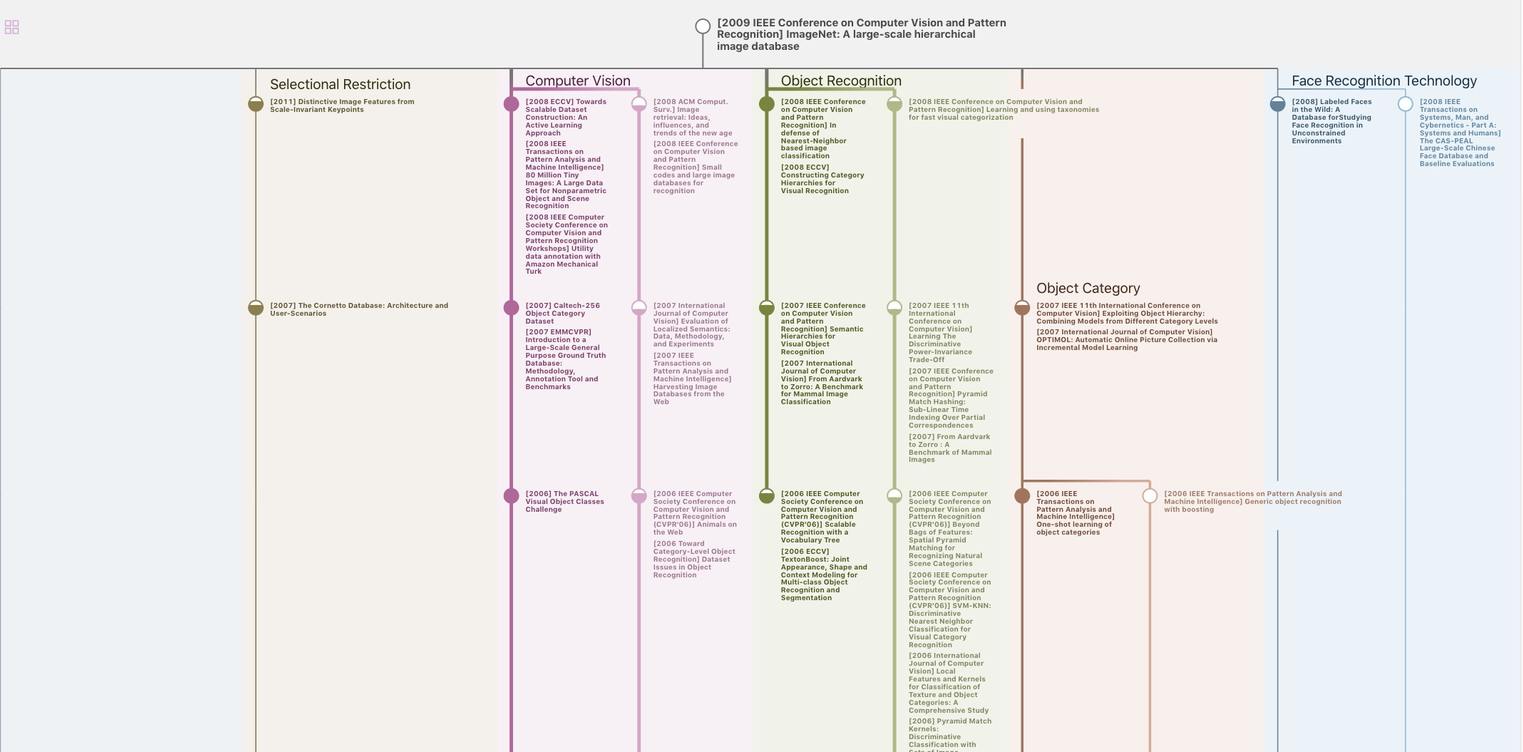
生成溯源树,研究论文发展脉络
Chat Paper
正在生成论文摘要