Russian Doll Network: Learning Nested Networks for Sample-Adaptive Dynamic Inference.
2021 IEEE/CVF International Conference on Computer Vision Workshops (ICCVW)(2021)
摘要
This work bridges recent advances in once-for-all (OFA) networks [1] and sample-adaptive dynamic networks. We propose a novel neural architecture dubbed as Russian doll network (RDN). Key differentiators of RDN are two-folds: first, a RDN topologically consists of a few nested subnetworks. Any smaller sub-network is completely embedded in all larger ones in a parameter-sharing manner. The computation flow of a RDN starts from the inner-most (and smallest) sub-network and sequentially executes larger ones according to the nesting order. A larger sub-network can re-use all intermediate features calculated at their inner sub-networks. This crucially ensures that each sub-network can conduct inference independently. Secondly, the nesting order of RDNs naturally plots the sequential neural path of a sample in the network. For an easy sample, much computation can be saved without much sacrifice of accuracy if an early-termination point can be intelligently determined. To this end, we formulate satisfying a specific accuracy-complexity tradeoff as a constrained optimization problem, solved via the Lagrangian multiplier theory. Comprehensive experiments of transforming several base models into RDN on ImageNet clearly demonstrate the superior accuracy-complexity balance of RDN.
更多查看译文
关键词
Bridges,Computer vision,Conferences,Computational modeling,Transforms,Computer architecture,Optimization
AI 理解论文
溯源树
样例
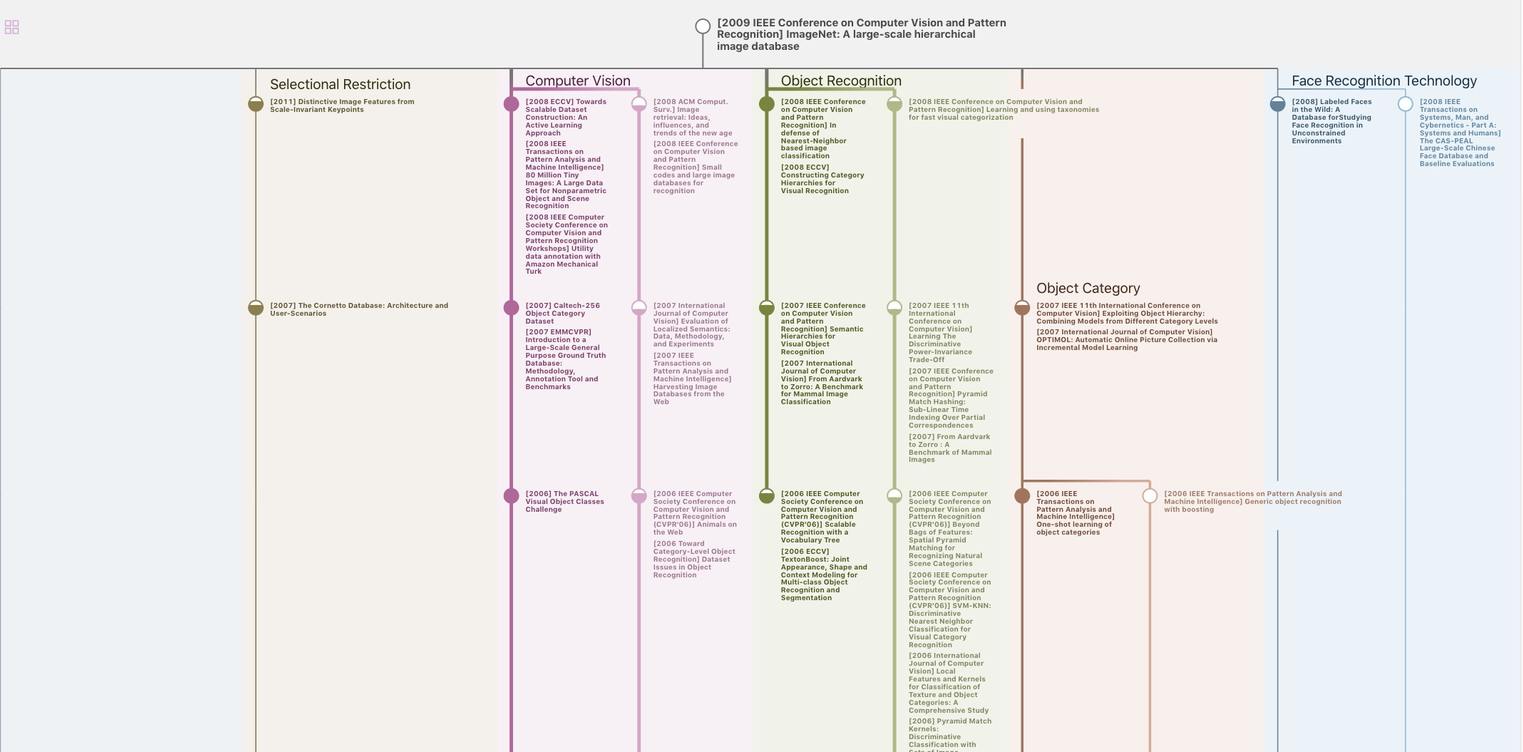
生成溯源树,研究论文发展脉络
Chat Paper
正在生成论文摘要