Cost-Sensitive Hypergraph Learning With F-Measure Optimization.
IEEE transactions on cybernetics(2023)
摘要
The imbalanced issue among data is common in many machine-learning applications, where samples from one or more classes are rare. To address this issue, many imbalanced machine-learning methods have been proposed. Most of these methods rely on cost-sensitive learning. However, we note that it is infeasible to determine the precise cost values even with great domain knowledge for those cost-sensitive machine-learning methods. So in this method, due to the superiority of F-measure on evaluating the performance of imbalanced data classification, we employ F-measure to calculate the cost information and propose a cost-sensitive hypergraph learning method with F-measure optimization to solve the imbalanced issue. In this method, we employ the hypergraph structure to explore the high-order relationships among the imbalanced data. Based on the constructed hypergraph structure, we optimize the cost value with F-measure and further conduct cost-sensitive hypergraph learning with the optimized cost information. The comprehensive experiments validate the effectiveness of the proposed method.
更多查看译文
关键词
Cost-sensitive,F-measure optimization,hypergraph learning,imbalanced data
AI 理解论文
溯源树
样例
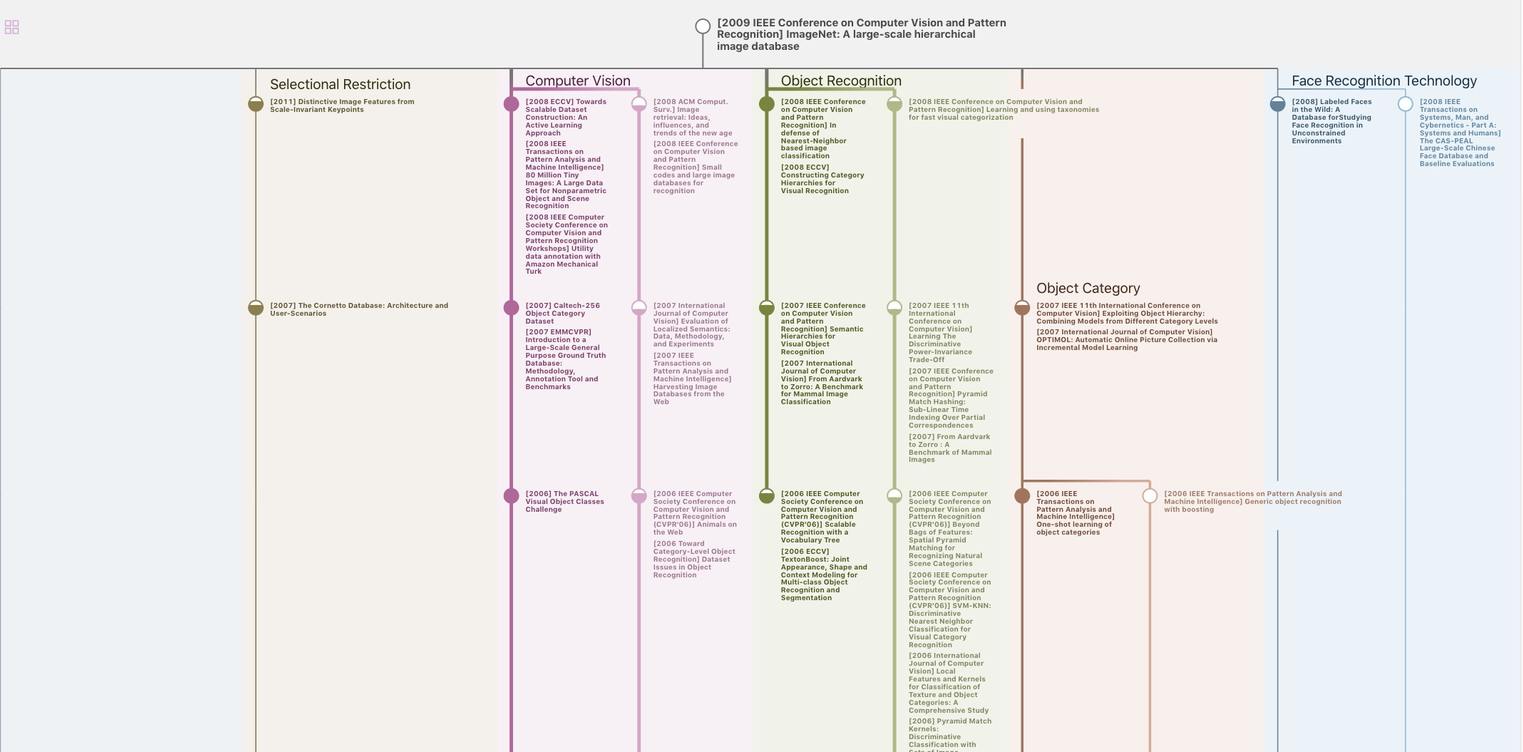
生成溯源树,研究论文发展脉络
Chat Paper
正在生成论文摘要