Privacy-Preserving Cross-Environment Human Activity Recognition
IEEE Transactions on Cybernetics(2023)
摘要
Recent studies have demonstrated the success of using the channel state information (CSI) from the WiFi signal to analyze human activities in a fixed and well-controlled environment. Those systems usually degrade when being deployed in new environments. A straightforward solution to solve this limitation is to collect and annotate data samples from different environments with advanced learning strategies. Although workable as reported, those methods are often privacy sensitive because the training algorithms need to access the data from different environments, which may be owned by different organizations. We present a practical method for the WiFi-based privacy-preserving cross-environment human activity recognition (HAR). It collects and shares information from different environments, while maintaining the privacy of individual person being involved. At the core of our approach is the utilization of the Johnson–Lindenstrauss transform, which is theoretically shown to be differentially private. Based on that, we further design an adversarial learning strategy to generate environment-invariant representations for HAR. We demonstrate the effectiveness of the proposed method with different data modalities from two real-life environments. More specifically, on the raw CSI dataset, it shows 2.18% and 1.24% improvements over challenging baselines for two environments, respectively. Moreover, with the discrete wavelet transform features, it further yields 5.71% and 1.55% improvements, respectively.
更多查看译文
关键词
Adversarial learning,deep learning,differential privacy,federated learning,human activity recognition (HAR),multimodal learning
AI 理解论文
溯源树
样例
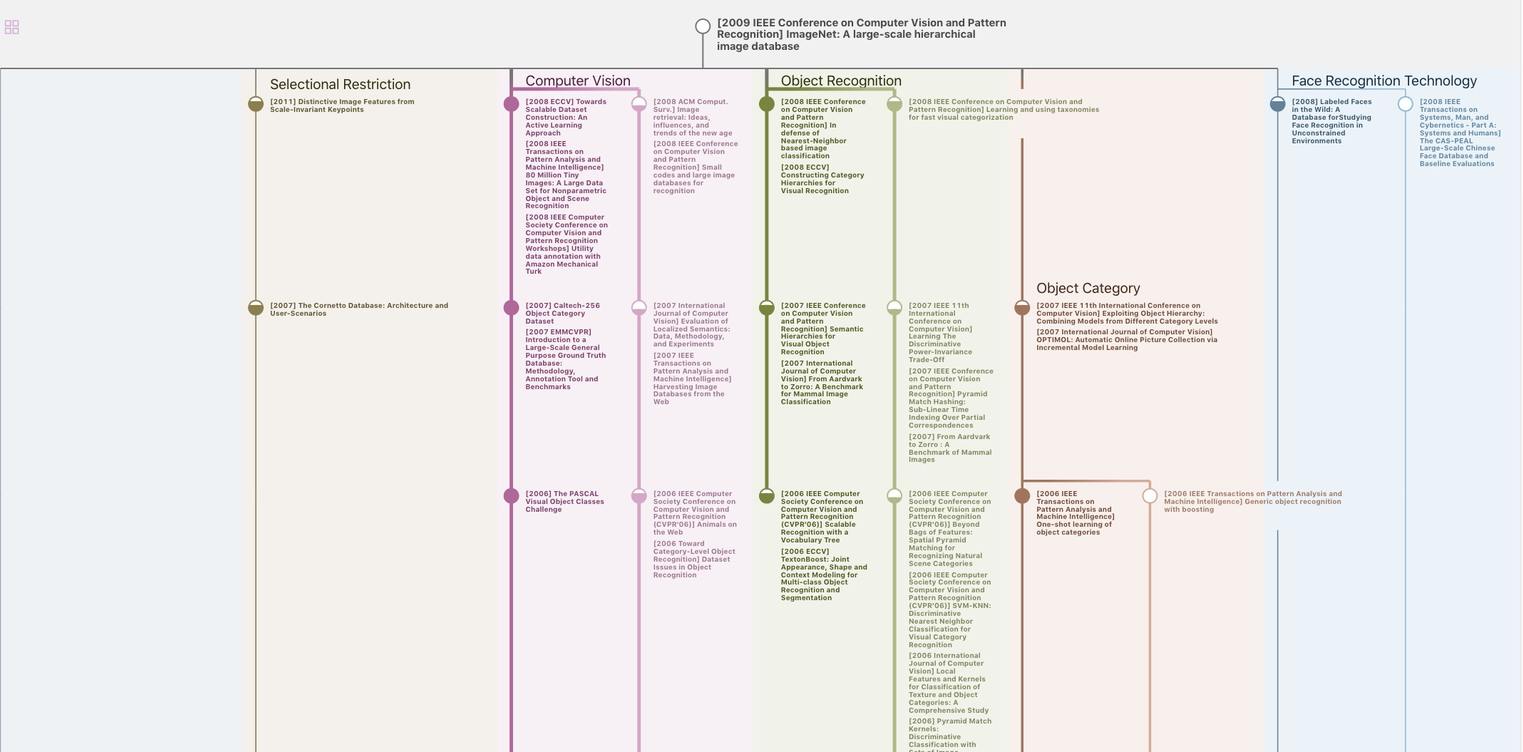
生成溯源树,研究论文发展脉络
Chat Paper
正在生成论文摘要