Predicting Stages in Omnichannel Path to Purchase: A Deep Learning Model
INFORMATION SYSTEMS RESEARCH(2022)
摘要
The proliferation of omnichannel practices and emerging technologies opens up new opportunities for companies to collect voluminous data across multiple channels. This study examines whether leveraging omnichannel data can lead to, statistically and economically, significantly better predictions on consumers' online path-to-purchase journeys, given the intrinsic fluidity in and heterogeneity brought forth by the digital transformation of traditional marketing. Using an omnichannel data set that captures consumers' online behavior in terms of their website browsing trajectories and their offline behavior in terms of physical location trajectories, we predict consumers' future path-to-purchase journeys based on their historical omnichannel behaviors. Using a state-of-the-art deep-learning algorithm, we find that using omnichannel data can significantly improve our model's predictive power. The lift curve analysis reveals that the omnichannel model outperforms the corresponding single-channel model by 7.38%. This enhanced predictive power benefits various heterogeneous online firms, regardless of their size, offline presence, mobile app availability, or whether they are selling single- or multicategory products. Using an illustrative example of targeted marketing, we further quantify the economic value of the improved predictive power using a cost-revenue analysis. Our paper contributes to the emerging literature on omnichannel marketing and sheds light on the inherent dynamics and fluidity in consumers' online path-to-purchase journeys.
更多查看译文
关键词
data value, omnichannel marketing, purchase journey prediction, deep learning
AI 理解论文
溯源树
样例
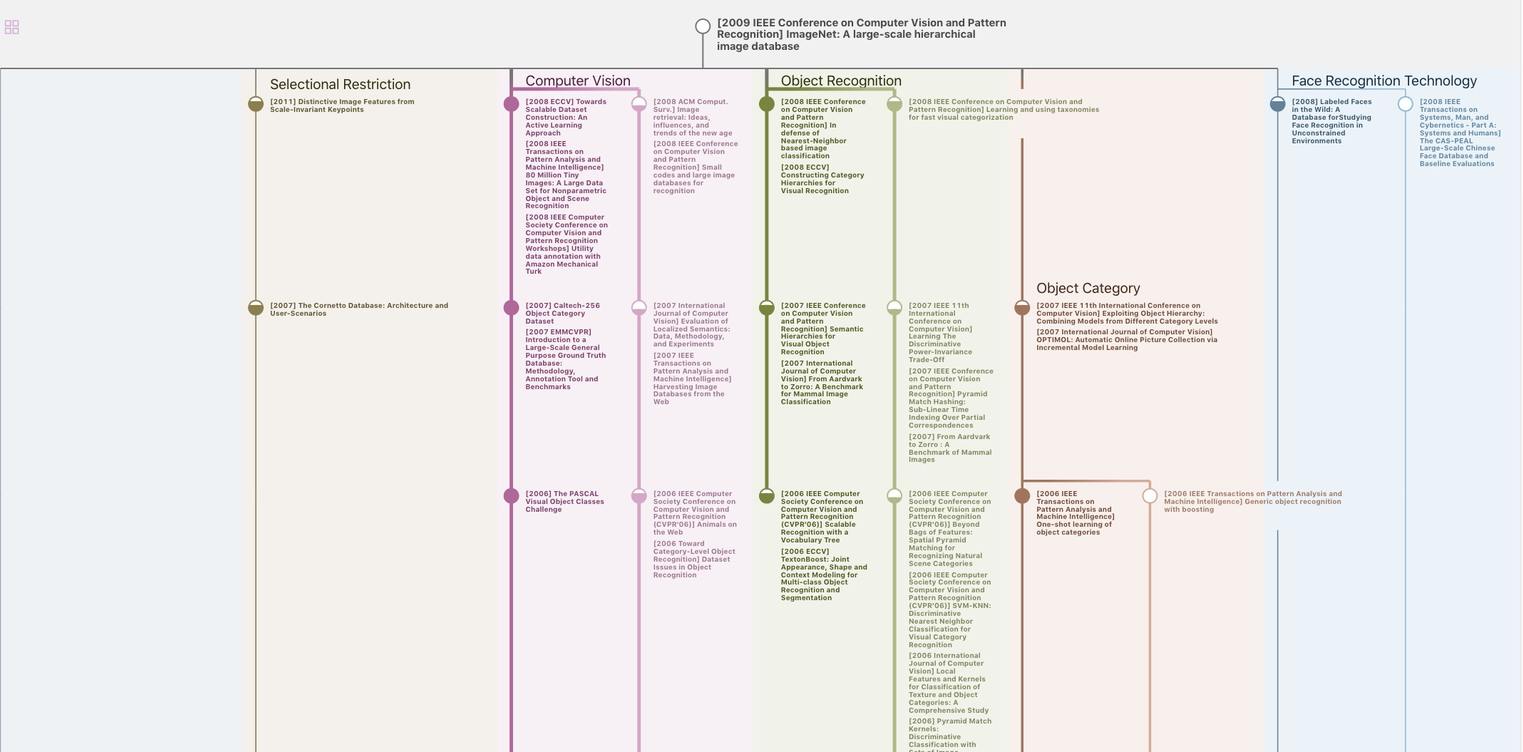
生成溯源树,研究论文发展脉络
Chat Paper
正在生成论文摘要