Monitoring deterioration of knee osteoarthritis using vibration arthrography in daily activities
COMPUTER METHODS AND PROGRAMS IN BIOMEDICINE(2022)
摘要
Background and Objective: Pathological recognition of knee joint using vibration arthrography (VAG) is increasingly becoming prevailed, due to the non-invasive and non-radiative benefits. However, knee joint health monitoring using VAG signals is a difficult problem, since VAG signals are contaminated by strong motion artifacts (MA) caused by knee movements during daily activities, such as squatting. So far few works have investigated this problem. Existing studies mainly focused on clinical diagnosis of knee disorders for 2-class (normal/abnormal) classification using VAG signals, which are less contaminated by MA in the scene when subjects perform knee extension and flexion movements in seated position. The purpose of this study is to propose a framework to monitor knee joint health during daily activities. Methods: In this paper, a general framework is designed to monitor knee joint health, which consists of VAG enhancement, feature extraction and fusion, and classification. VAG enhancement aims to remove MA and irrelevant components of knee joint pathologies in raw VAG signals. Distinctive features from enhanced VAG signals are obtained in feature extraction and fusion. Classification can not only distinguish whether the knee joint is normal or abnormal, but also distinguish the grade of deterioration of knee osteoarthritis. Results: 813 VAG signals from VAG-OA dataset, which is currently the largest VAG dataset, have been collected from medical cases in Xijing Hospital of the Fourth Military Medical University during daily activities. Experimental results on VAG-OA dataset showed that the accuracy of 2-class (normal/abnormal) classification was 95.9% with sensitivity 98.1% and specificity 93.3%. For 5-class classification based on deterioration grades of osteoarthritis (OA), we obtained accuracy 74.4%, sensitivity 52.6% and specificity 78.3%. Conclusion: The VAG-OA dataset can be used not only for knee joint health monitoring but also for clinical diagnosis. The designed framework on VAG-OA dataset has high classification accuracy, which is of great value to monitor knee joint health using VAG signals during daily activities. The results also demonstrate that the designed framework significantly outperforms the baselines and several state-of-the-art methods. (C) 2021 Elsevier B.V. All rights reserved.
更多查看译文
关键词
Keywords: Vibration arthrography (VAG), VAG Enhancement, Monitoring, Osteoarthritis (OA), VAG Datasets
AI 理解论文
溯源树
样例
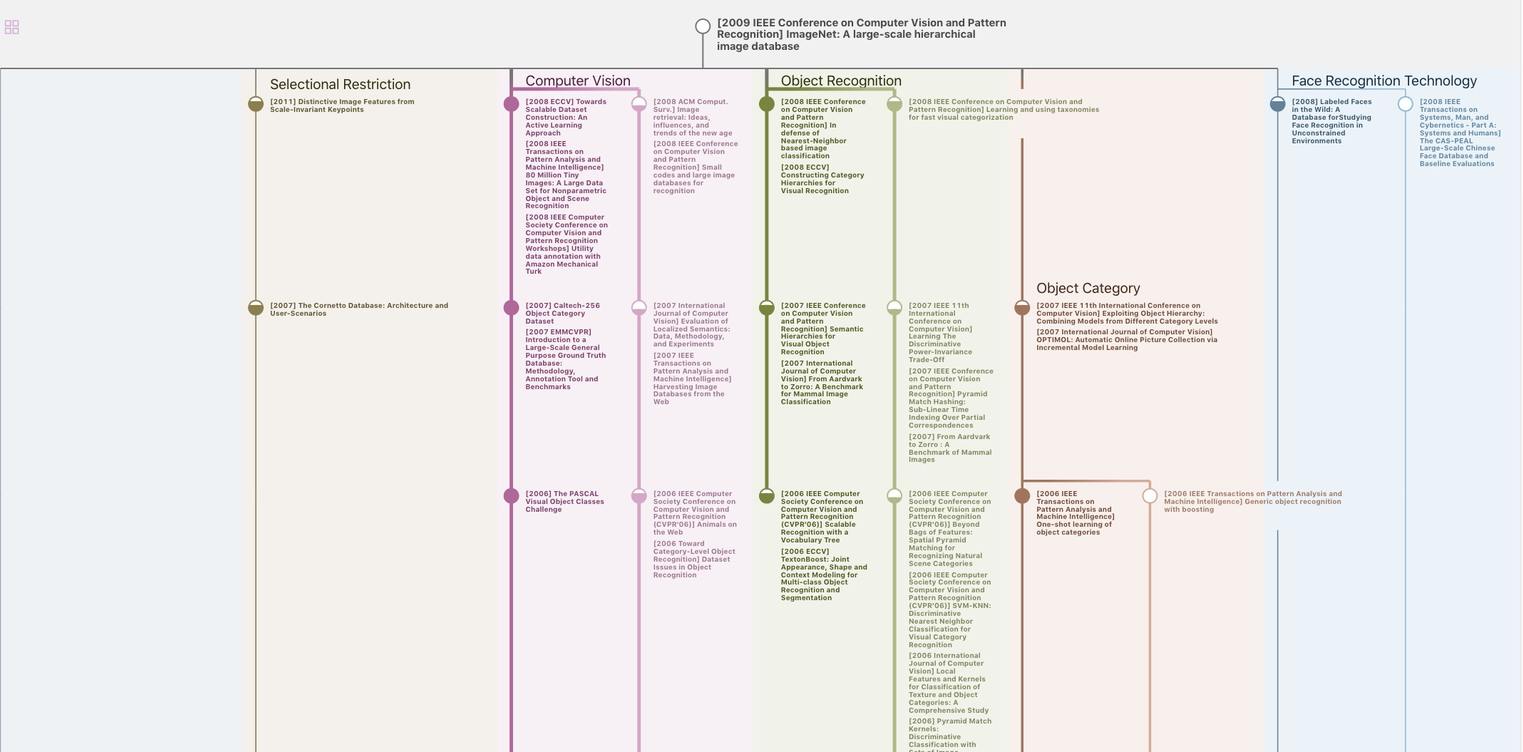
生成溯源树,研究论文发展脉络
Chat Paper
正在生成论文摘要