Temporal Effects on Pre-trained Models for Language Processing Tasks.
Transactions of the Association for Computational Linguistics(2022)
摘要
Keeping the performance of language technologies optimal as time passes is of great practical interest. Here we survey prior work concerned with the effect of time on system performance, establishing more nuanced terminology for discussing the topic and proper experimental design to support solid conclusions about the observed phenomena. We present a set of experiments with systems powered by large neural pretrained representations for English to demonstrate that {\em temporal model deterioration} is not as big a concern, with some models in fact improving when tested on data drawn from a later time period. It is however the case that {\em temporal domain adaptation} is beneficial, with better performance for a given time period possible when the system is trained on temporally more recent data. Our experiments reveal that the distinctions between temporal model deterioration and temporal domain adaptation becomes salient for systems built upon pretrained representations. Finally we examine the efficacy of two approaches for temporal domain adaptation without human annotations on new data, with self-labeling proving to be superior to continual pre-training. Notably, for named entity recognition, self-labeling leads to better temporal adaptation than human annotation.
更多查看译文
关键词
temporal effects,language,processing,models,pre-trained
AI 理解论文
溯源树
样例
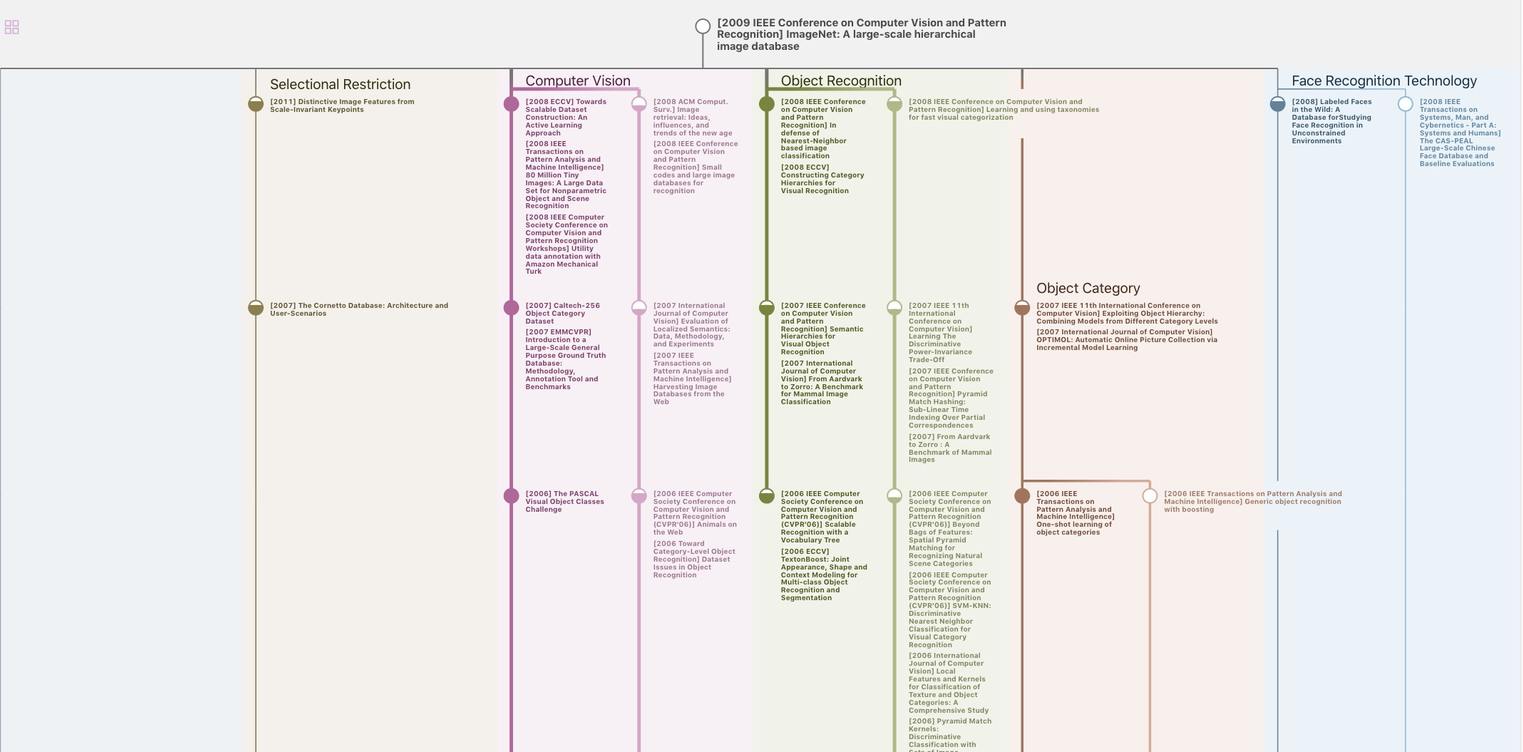
生成溯源树,研究论文发展脉络
Chat Paper
正在生成论文摘要