Animal Behavior Classification via Accelerometry Data and Recurrent Neural Networks
arxiv(2021)
摘要
We study the classification of animal behavior using accelerometry data through various recurrent neural network (RNN) models. We evaluate the classification performance and complexity of the considered models, which feature long short-time memory (LSTM) or gated recurrent unit (GRU) architectures with varying depths and widths, using four datasets acquired from cattle via collar or ear tags. We also include two state-of-the-art convolutional neural network (CNN)-based time-series classification models in the evaluations. The results show that the RNN-based models can achieve similar or higher classification accuracy compared with the CNN-based models while having less computational and memory requirements. We also observe that the models with GRU architecture generally outperform the ones with LSTM architecture in terms of classification accuracy despite being less complex. A single-layer uni-directional GRU model with 64 hidden units appears to offer a good balance between accuracy and complexity making it suitable for implementation on edge/embedded devices.
更多查看译文
关键词
animal behavior classification,animal behavior,accelerometry data
AI 理解论文
溯源树
样例
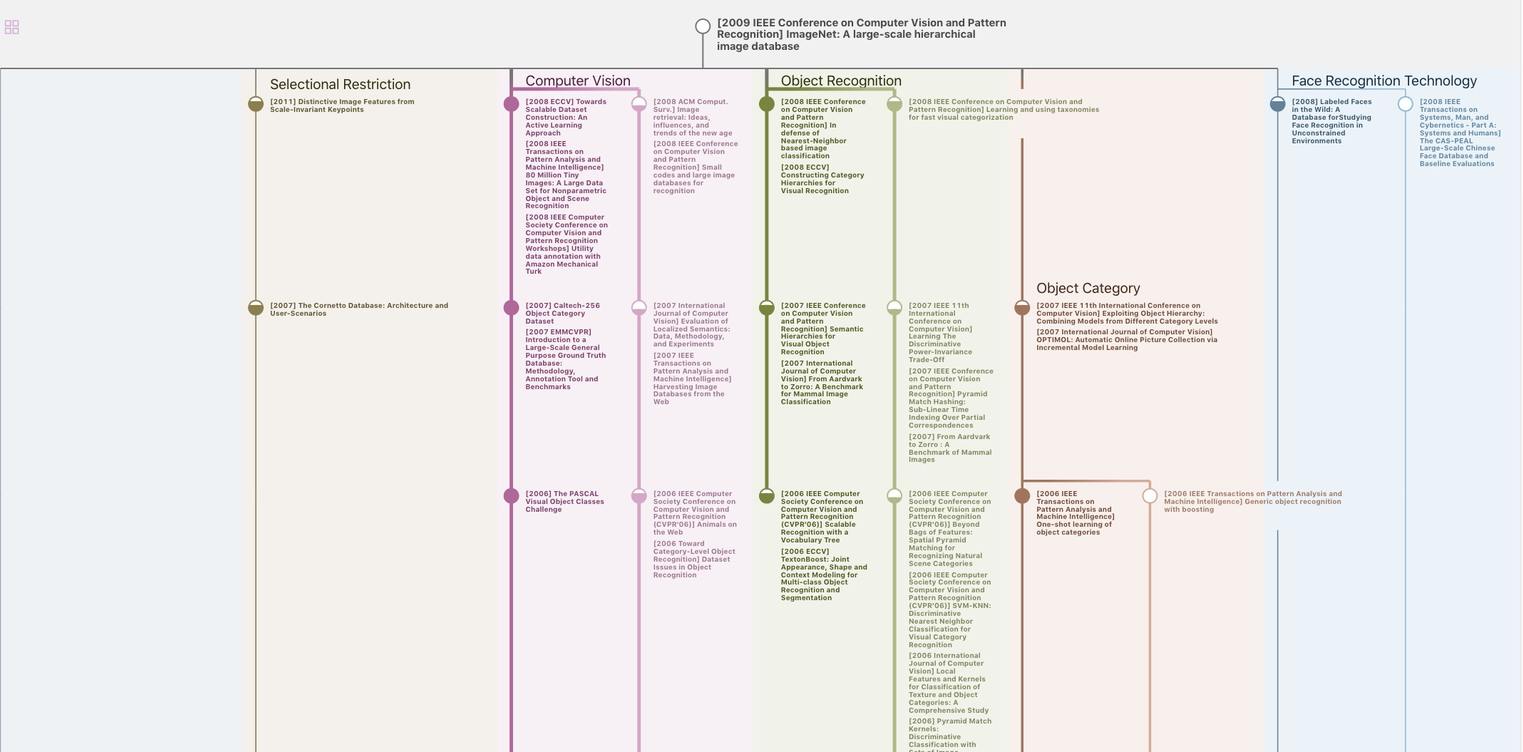
生成溯源树,研究论文发展脉络
Chat Paper
正在生成论文摘要