Reliable Graph Neural Networks for Drug Discovery Under Distributional Shift
arxiv(2021)
摘要
The concern of overconfident mis-predictions under distributional shift demands extensive reliability research on Graph Neural Networks used in critical tasks in drug discovery. Here we first introduce CardioTox, a real-world benchmark on drug cardio-toxicity to facilitate such efforts. Our exploratory study shows overconfident mis-predictions are often distant from training data. That leads us to develop distance-aware GNNs: GNN-SNGP. Through evaluation on CardioTox and three established benchmarks, we demonstrate GNN-SNGP's effectiveness in increasing distance-awareness, reducing overconfident mis-predictions and making better calibrated predictions without sacrificing accuracy performance. Our ablation study further reveals the representation learned by GNN-SNGP improves distance-preservation over its base architecture and is one major factor for improvements.
更多查看译文
关键词
reliable graph neural networks,drug discovery,neural networks
AI 理解论文
溯源树
样例
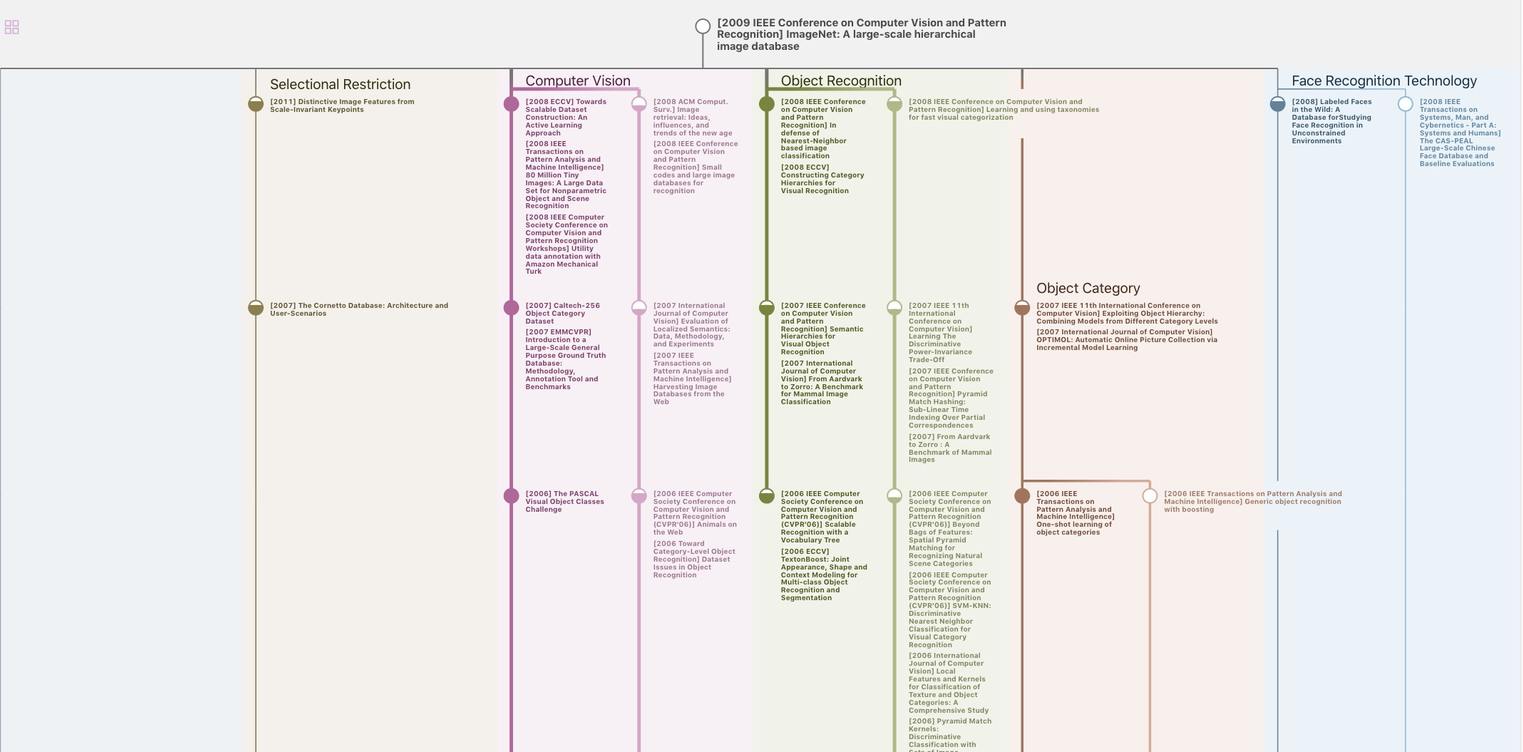
生成溯源树,研究论文发展脉络
Chat Paper
正在生成论文摘要