TraDE: A Simple Self-Attention-Based Density Estimator
user-613ea93de55422cecdace10f(2021)
摘要
We present TraDE, a self-attention-based architecture for auto-regressive density estimation with continuous and discrete valued data. Our model is trained using a penalized maximum likelihood objective, which ensures that samples from the density estimate resemble the training data distribution. The use of self-attention means that the model need not retain conditional sufficient statistics during the auto-regressive process beyond what is needed for each covariate. On standard tabular and image data benchmarks, TraDE produces significantly better density estimates than existing approaches such as normalizing flow estimators and recurrent auto-regressive models. However log-likelihood on held-out data only partially reflects how useful these estimates are in real-world applications. In order to systematically evaluate density estimators, we present a suite of tasks such as regression using generated samples, out-of-distribution detection, and robustness to noise in the training data and demonstrate that TraDE works well in these scenarios.
更多查看译文
关键词
Density estimation,Estimator,Sufficient statistic,Covariate,Robustness (computer science),Regression,Noise,Algorithm,Image (mathematics),Computer science
AI 理解论文
溯源树
样例
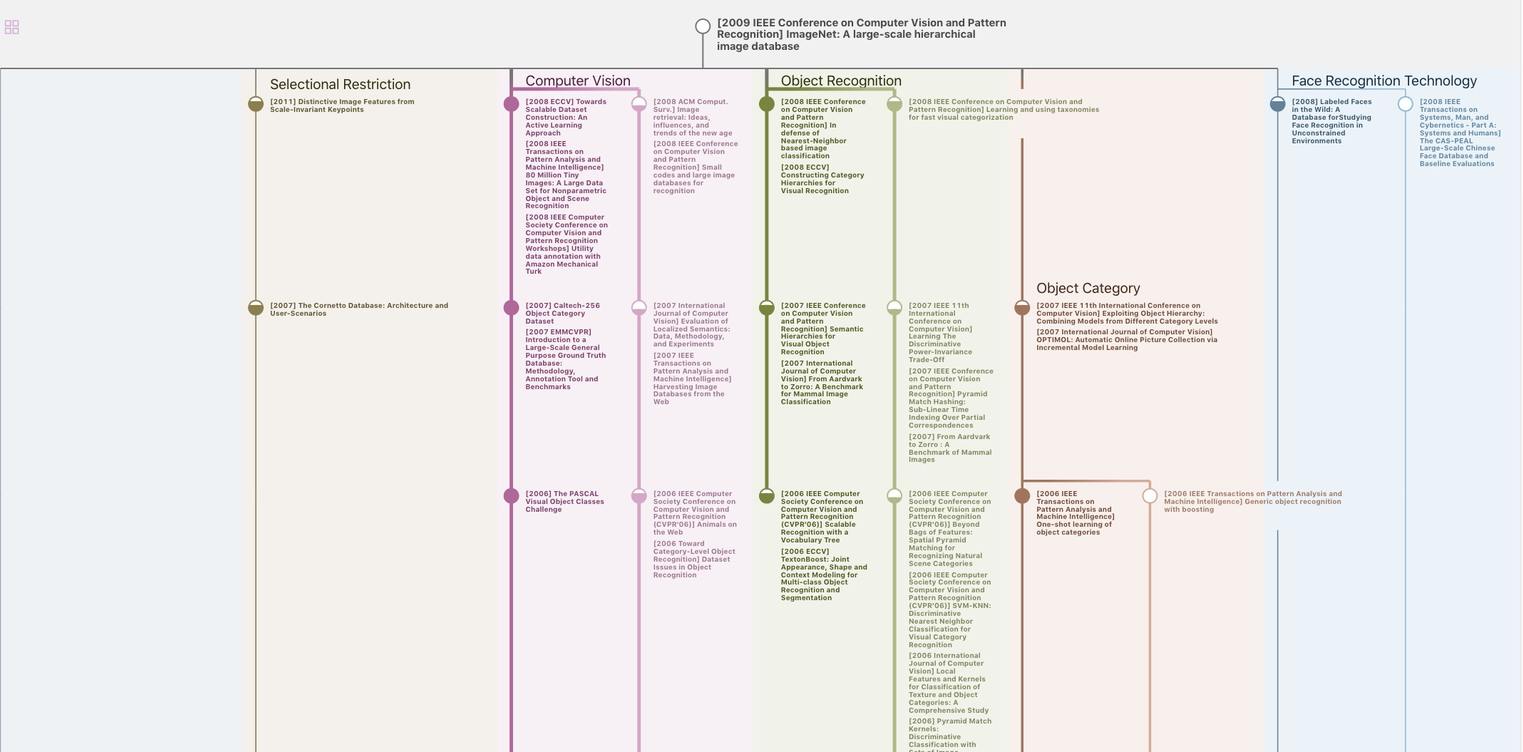
生成溯源树,研究论文发展脉络
Chat Paper
正在生成论文摘要