On the Integration of Self-Attention and Convolution
2022 IEEE/CVF Conference on Computer Vision and Pattern Recognition (CVPR)(2022)
摘要
Convolution and self-attention are two powerful techniques for representation learning, and they are usually considered as two peer approaches that are distinct from each other. In this paper, we show that there exists a strong underlying relation between them, in the sense that the bulk of computations of these two paradigms are in fact done with the same operation. Specifically, we first show that a traditional convolution with kernel size k × k can be decomposed into k
2
individual 1 × 1 convolutions, followed by shift and summation operations. Then, we interpret the projections of queries, keys, and values in self-attention module as multiple 1 × 1 convolutions, followed by the computation of attention weights and aggregation of the values. Therefore, the first stage of both two modules comprises the similar operation. More importantly, the first stage contributes a dominant computation complexity (square of the channel size) comparing to the second stage. This observation naturally leads to an elegant integration of these two seemingly distinct paradigms, i.e., a mixed model that enjoys the benefit of both self-Attention and Convolution (ACmix), while having minimum compu-tational overhead compared to the pure convolution or self-attention counterpart. Extensive experiments show that our model achieves consistently improved results over com-petitive baselines on image recognition and downstream tasks. Code and pre-trained models will be released at https://github.com/LeapLabTHU/ACmix and https://gitee.com/mindspore/models.
更多查看译文
关键词
Deep learning architectures and techniques, Machine learning, Recognition: detection,categorization,retrieval, Representation learning
AI 理解论文
溯源树
样例
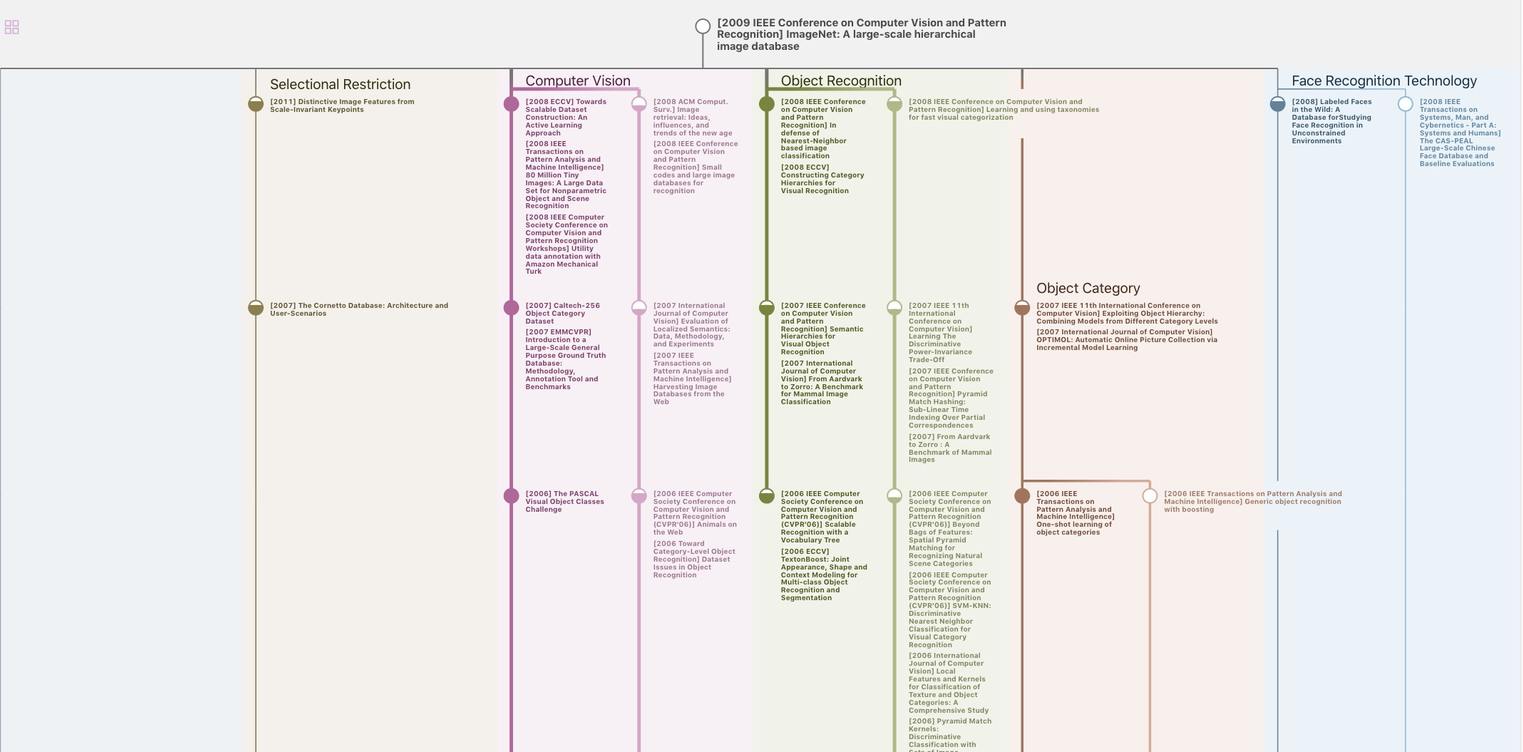
生成溯源树,研究论文发展脉络
Chat Paper
正在生成论文摘要