Multi-center sparse learning and decision fusion for automatic COVID-19 diagnosis
APPLIED SOFT COMPUTING(2022)
摘要
The coronavirus disease 2019 (COVID-19) pandemic caused by the novel severe acute respiratory syndrome coronavirus 2 (SARS-CoV-2) has led to a sharp increase in hospitalized patients with multiorgan disease pneumonia. Early and automatic diagnosis of COVID-19 is essential to slow down the spread of this epidemic and reduce the mortality of patients infected with SARS-CoV-2. In this paper, we propose a joint multi-center sparse learning (MCSL) and decision fusion scheme exploiting chest CT images for automatic COVID-19 diagnosis. Specifically, considering the inconsistency of data in multiple centers, we first convert CT images into histogram of oriented gradient (HOG) images to reduce the structural differences between multi-center data and enhance the generalization performance. We then exploit a 3-dimensional convolutional neural network (3D-CNN) model to learn the useful information between and within 3D HOG image slices and extract multi-center features. Furthermore, we employ the proposed MCSL method that learns the intrinsic structure between multiple centers and within each center, which selects discriminative features to jointly train multi-center classifiers. Finally, we fuse these decisions made by these classifiers. Extensive experiments are performed on chest CT images from five centers to validate the effectiveness of the proposed method. The results demonstrate that the proposed method can improve COVID-19 diagnosis performance and outperform the state-of-the-art methods. (C) 2021 Published by Elsevier B.V.
更多查看译文
关键词
COVID-19 diagnosis, Histogram of oriented gradient, 3D-CNN, Multi-center sparse learning, Decision fusion
AI 理解论文
溯源树
样例
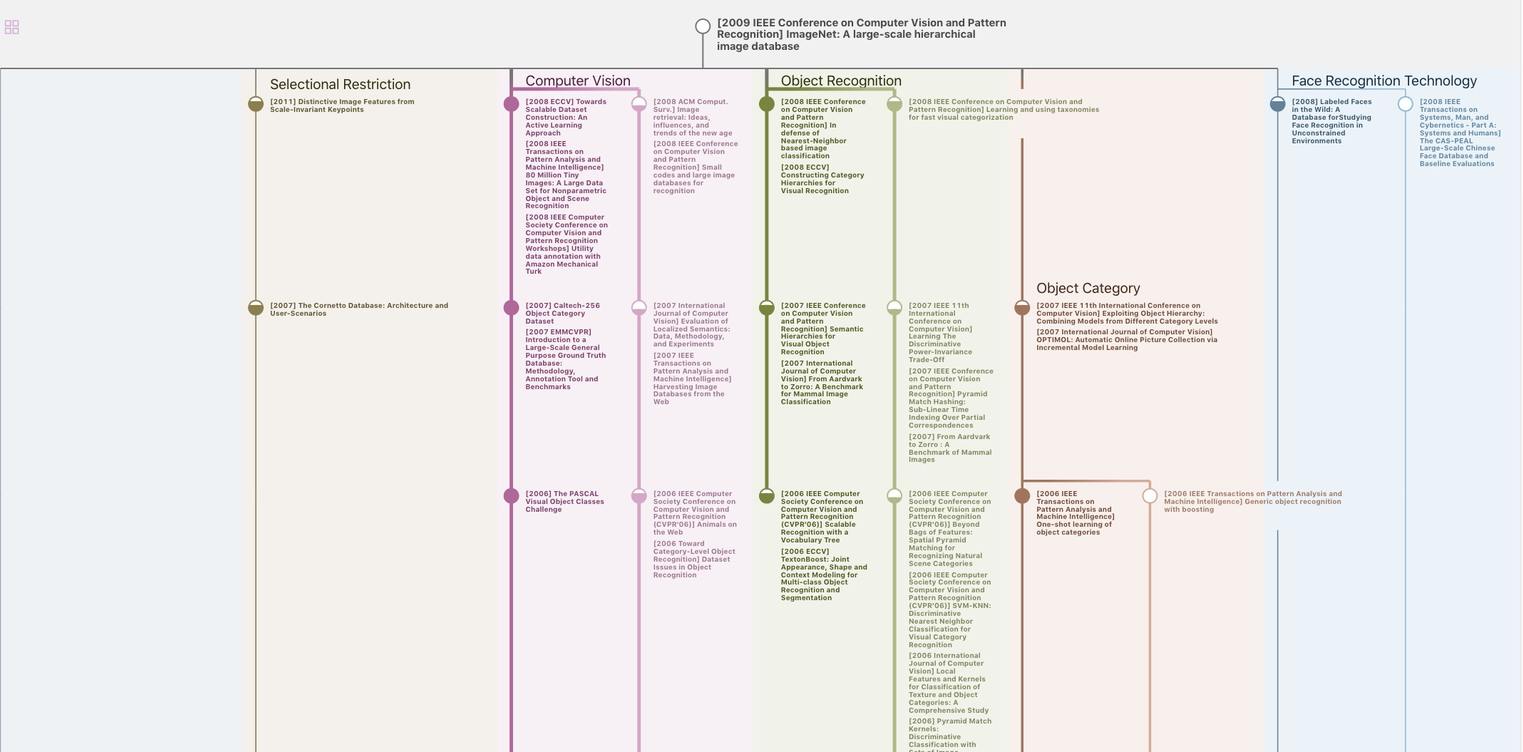
生成溯源树,研究论文发展脉络
Chat Paper
正在生成论文摘要