Consensus Synergizes With Memory: A Simple Approach for Anomaly Segmentation in Urban Scenes
IEEE TRANSACTIONS ON CIRCUITS AND SYSTEMS FOR VIDEO TECHNOLOGY(2024)
摘要
Anomaly segmentation is a critical task for safety-critical applications, such as autonomous driving in urban environments. Its objective is to detect out-of-distribution (OOD) samples with unseen categories, given a pre-trained segmentation model. The core challenge of this task is how to distinguish hard in-distribution samples from OOD samples, which has not been explicitly discussed in previous research. In this paper, we propose a simple yet effective approach named CosMe (Consensus Synergizes with Memory) to address this challenge. CosMe consists of two key components: 1) building a memory bank comprising seen prototypes extracted from multiple layers of the given segmentation model, and 2) training an auxiliary model that mimics the behavior of the given model and using the consensus of their mid-level features as complementary cues that synergize with the memory bank. The former serves as a baseline that can detect all potential outliers, including both OOD and hard in-distribution samples; the latter assists in distinguishing between these two types of outliers. Experimental results on several urban scene anomaly segmentation datasets demonstrate that CosMe outperforms previous approaches by a significant margin.
更多查看译文
关键词
Training,Task analysis,Uncertainty,Prototypes,Feature extraction,Image reconstruction,Autonomous vehicles,Semantic segmentation,anomaly detection,clustering
AI 理解论文
溯源树
样例
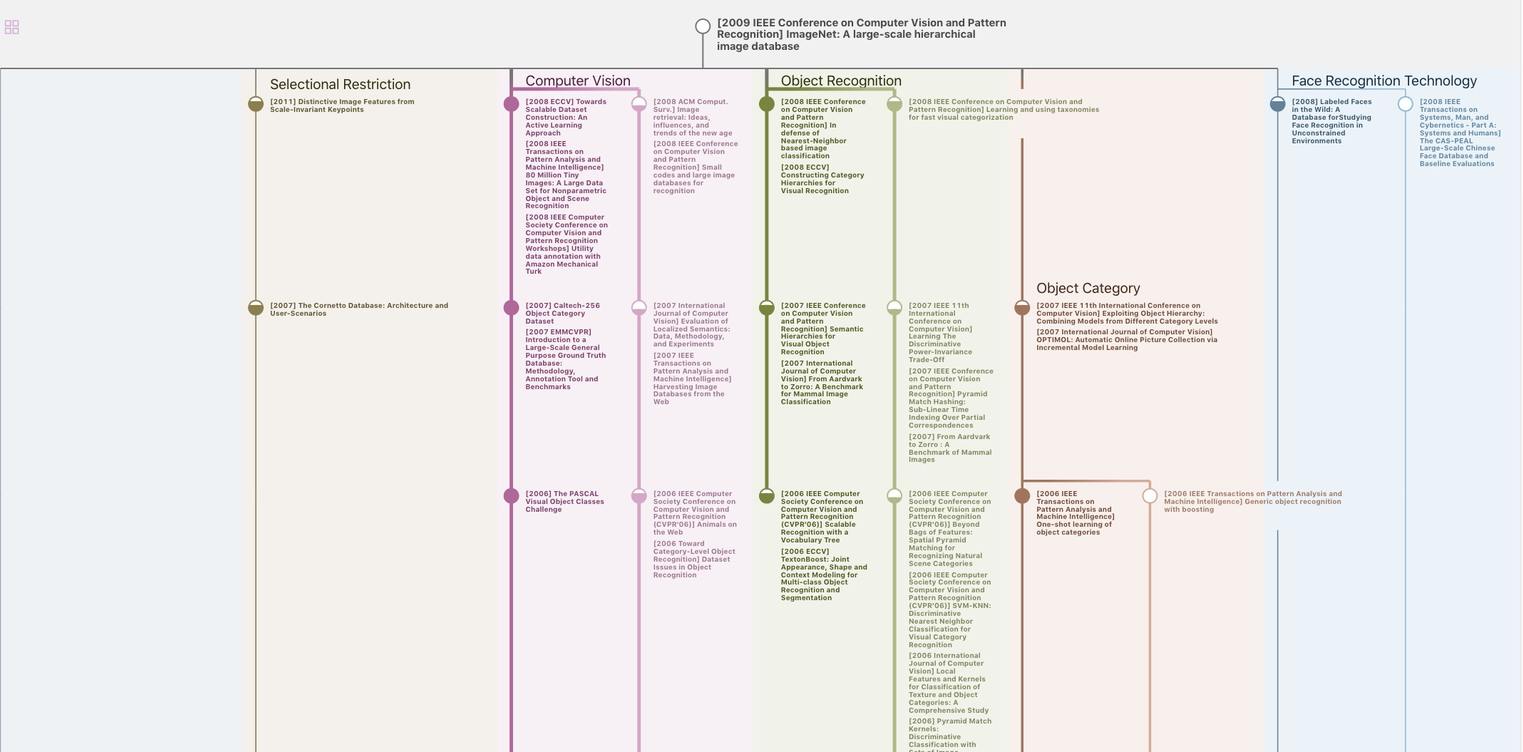
生成溯源树,研究论文发展脉络
Chat Paper
正在生成论文摘要