FENeRF: Face Editing in Neural Radiance Fields
IEEE Conference on Computer Vision and Pattern Recognition(2022)
摘要
Previous portrait image generation methods roughly fall into two categories: 2D GANs and 3D-aware GANs. 2D GANs can generate high fidelity portraits but with low view consistency. 3D-aware GAN methods can maintain view consistency but their generated images are not locally editable. To overcome these limitations, we propose FENeRF, a 3D-aware generator that can produce view-consistent and locally-editable portrait images. Our method uses two decoupled latent codes to generate corresponding facial semantics and texture in a spatial-aligned 3D volume with shared geometry. Benefiting from such underlying 3D representation, FENeRF can Jointly render the boundary-aligned image and semantic mask and use the semantic mask to edit the 3D volume via GAN inversion. We further show such 3D representation can be learned from widely available monocular image and semantic mask pairs. Moreover, we reveal that Joint learning semantics and texture helps to generate finer geometry. Our experiments demonstrate that FENeRF outperforms state-of-the-art methods in various face editing tasks. Code is available at https://github.com/MrTornado24/FENeRF.
更多查看译文
关键词
Image and video synthesis and generation, Face and gestures
AI 理解论文
溯源树
样例
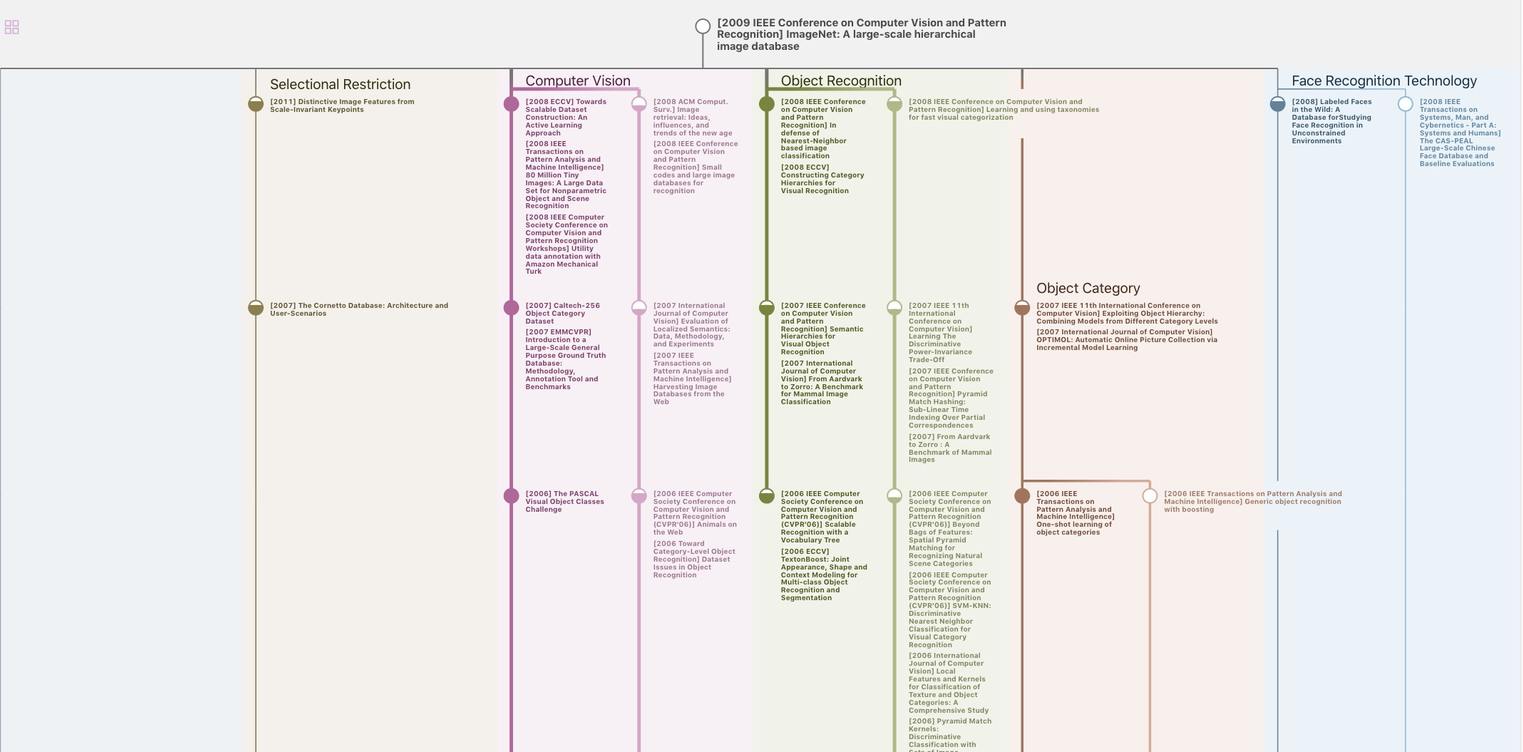
生成溯源树,研究论文发展脉络
Chat Paper
正在生成论文摘要