Robust Partial-to-Partial Point Cloud Registration in a Full Range
IEEE ROBOTICS AND AUTOMATION LETTERS(2024)
摘要
Registration of 3D objects from point clouds is a challenging task due to sparse and noisy measurements, incomplete observations, and large transformations. In this work, we propose the G raph M atching C onsensus Net work (GMCNet ) to estimate faithful correspondences for full-range Partial-to-Partial point cloud Registration (PPR) in object-level registration scenarios. To encode robust point descriptors, we employ a novel Transformation-robust Point Transformer (TPT ) module to adaptively aggregate local features with respect to the structural relations, taking advantage of both handcrafted rotation-invariant (RI) features and noise-resilient spatial coordinates. Based on the synergy of hierarchical graph networks and graphical modeling, we propose the Hierarchical Graphical Modeling (HGM ) architecture to encode robust descriptors comprising of i) a unary term learned from RI features, and ii) multiple smoothness terms encoded from neighboring point relations at different scales through our TPT modules. Extensive experiments show that GMCNet outperforms previous state-of-the-art methods for PPR.
更多查看译文
关键词
Point cloud compression,Three-dimensional displays,Solid modeling,Shape,Rail to rail inputs,Noise measurement,Feature extraction,Deep Learning for Visual Perception,Visual Learning
AI 理解论文
溯源树
样例
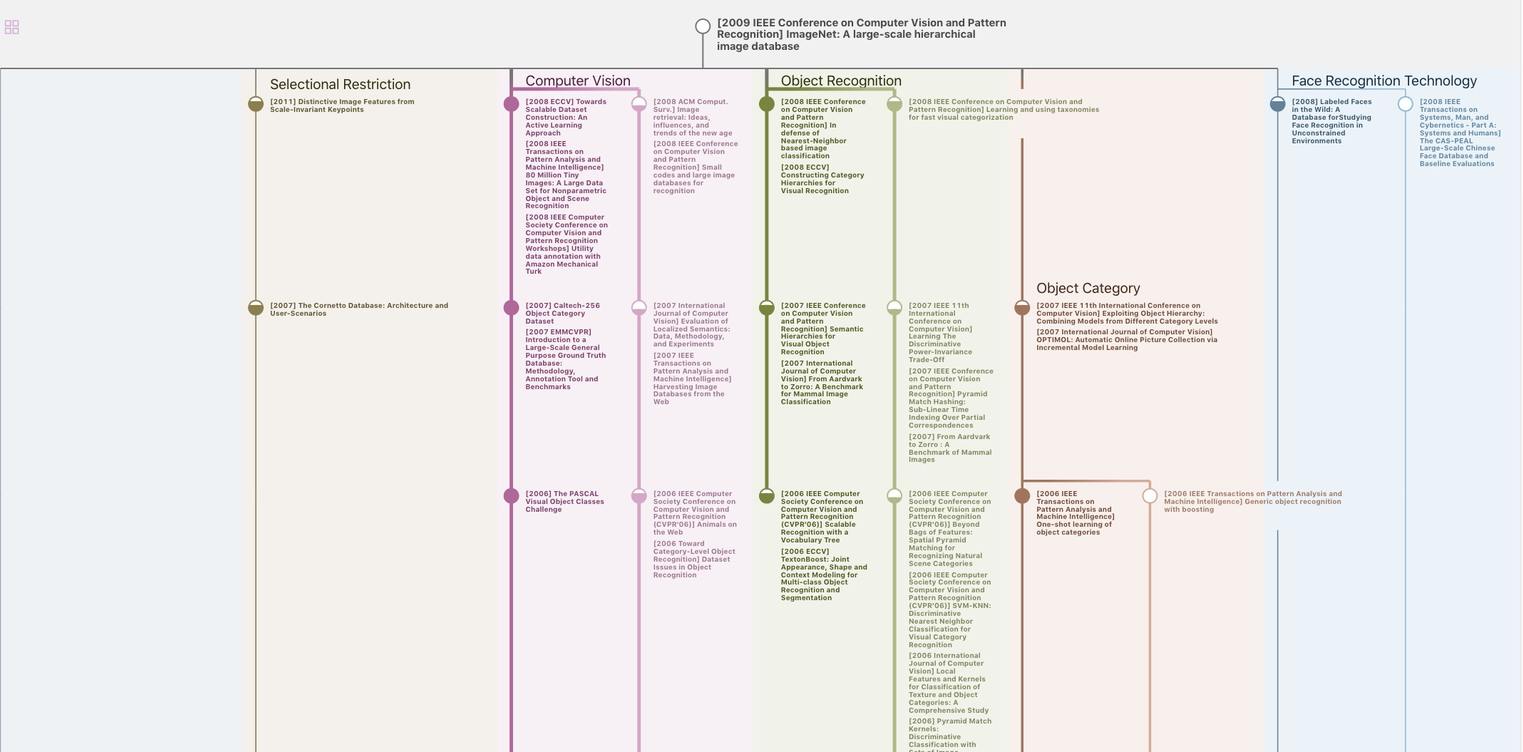
生成溯源树,研究论文发展脉络
Chat Paper
正在生成论文摘要