Neural Symplectic Integrator with Hamiltonian Inductive Bias for the Gravitational $N$-body Problem
arxiv(2021)
摘要
The gravitational $N$-body problem, which is fundamentally important in astrophysics to predict the motion of $N$ celestial bodies under the mutual gravity of each other, is usually solved numerically because there is no known general analytical solution for $N>2$. Can an $N$-body problem be solved accurately by a neural network (NN)? Can a NN observe long-term conservation of energy and orbital angular momentum? Inspired by Wistom & Holman (1991)'s symplectic map, we present a neural $N$-body integrator for splitting the Hamiltonian into a two-body part, solvable analytically, and an interaction part that we approximate with a NN. Our neural symplectic $N$-body code integrates a general three-body system for $10^{5}$ steps without diverting from the ground truth dynamics obtained from a traditional $N$-body integrator. Moreover, it exhibits good inductive bias by successfully predicting the evolution of $N$-body systems that are no part of the training set.
更多查看译文
关键词
neural symplectic integrator,hamiltonian inductive bias
AI 理解论文
溯源树
样例
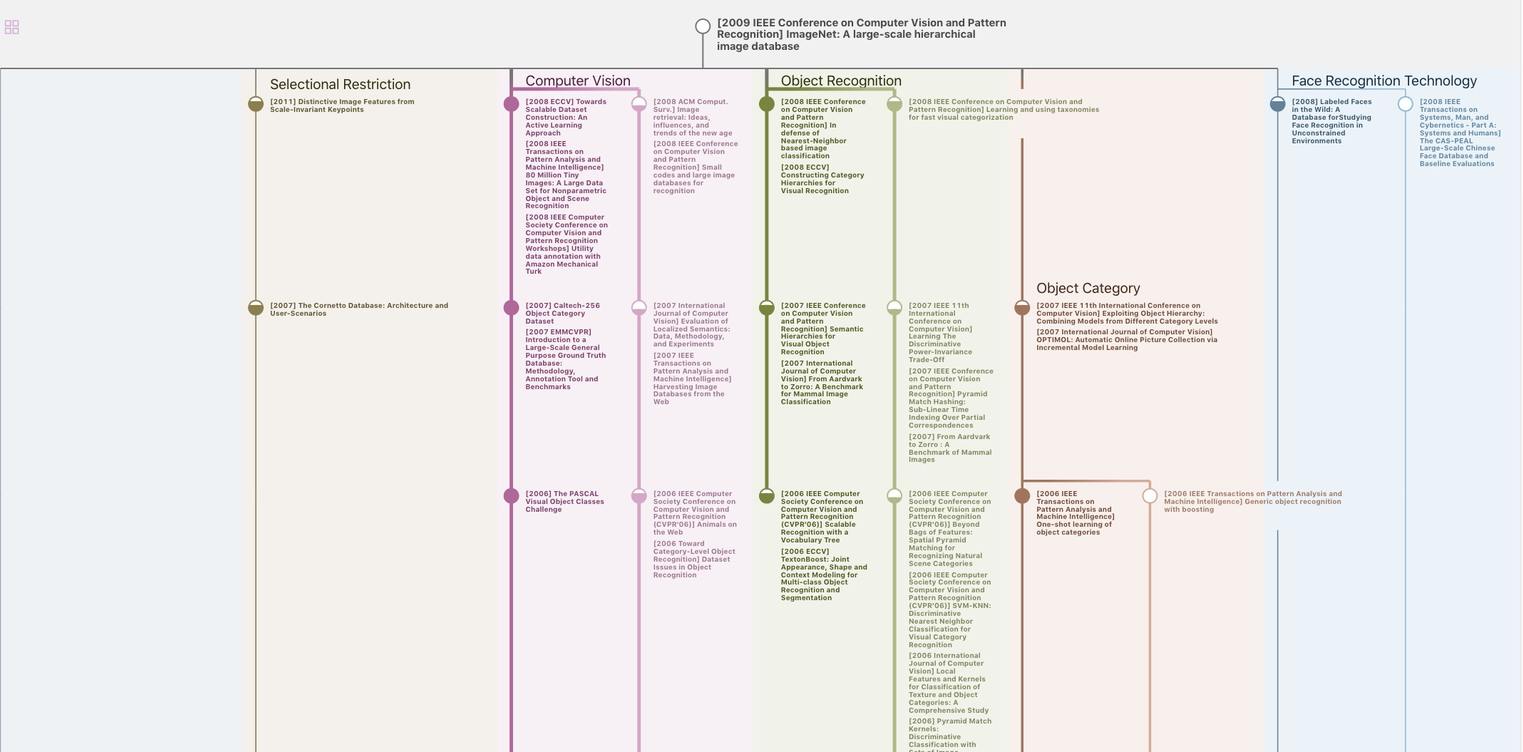
生成溯源树,研究论文发展脉络
Chat Paper
正在生成论文摘要