Toe PPG sample extension for supervised machine learning approaches to simultaneously predict type 2 diabetes and peripheral neuropathy
BIOMEDICAL SIGNAL PROCESSING AND CONTROL(2022)
摘要
The rapid increase in machine learning prediction algorithms has generated a strong demand for applications that can successfully predict type 2 diabetes and/or peripheral neuropathy in elderly subjects with metabolic disorders; however, the number of samples is a key issue determining accurate prediction. To address this challenge, streaming data of the instantaneous frequency of the maximal energy (fEmax) were calculated from toe photoplethysmography signals, followed by procedures to increase the quality of the machine learning model. Three important machine learning prediction algorithms (Fisher discriminant analysis, multinomial logistics regression, and artificial neural network) were adopted for proof. The correspondence to all parameters was statistically significant using the streaming data of fEmax, whereas only three were significant when there was no sample extension. The prediction rate of Fisher discriminant analysis, multinomial logistics regression, and artificial neural network increased from 75.30% to 85.43%, 80.07% to 86.30%, and 86.85% to 93.07%, respectively, when the streaming fEmax data were used. This study is a novel attempt to establish clinical multi prediction through the application of toe PPG sample extension, demonstrating enhanced accuracy in predicting which elderly subjects among those with type 2 diabetes will develop diabetic peripheral neuropathy (DPN) within six years.
更多查看译文
关键词
Photoplethysmography (PPG), Sample extension, Type 2 diabetes, Diabetic peripheral neuropathy (DPN), Instantaneous frequency of the maximal energy (f(Emax)), Machine learning algorithms, Fisher discriminant analysis, Multinomial logistics regression, Artificial neural network (ANN)
AI 理解论文
溯源树
样例
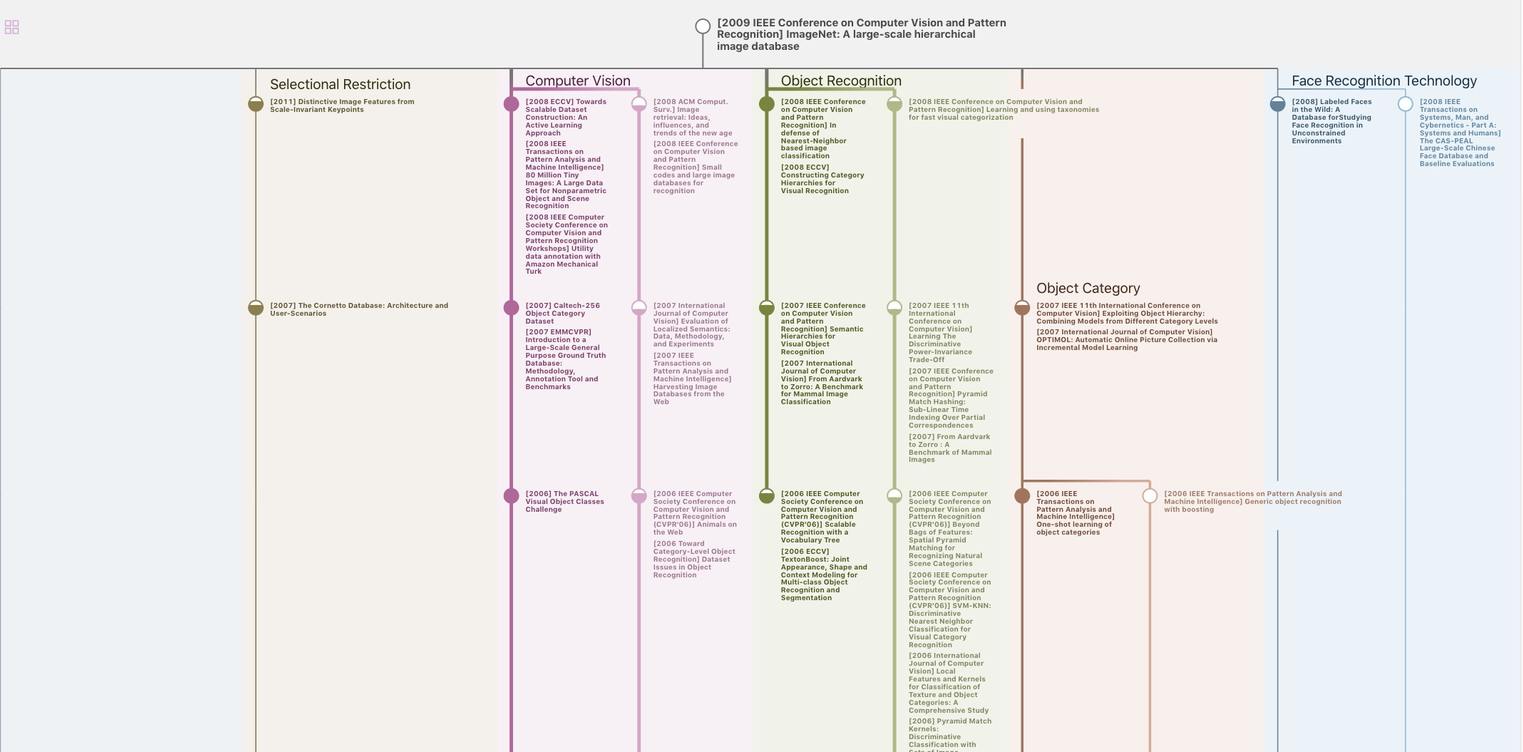
生成溯源树,研究论文发展脉络
Chat Paper
正在生成论文摘要