Deep learning based fall detection using smartwatches for healthcare applications
BIOMEDICAL SIGNAL PROCESSING AND CONTROL(2022)
摘要
We implement a smart watch-based system to predict fall detection. We differentiate fall detection from four common daily activities: sitting, squatting, running, and walking. Moreover, we separate falling into falling from a chair and falling from a standing position. We develop a mobile application that collects the acceleration and gyroscope sensor data and transfers them to the cloud. In the cloud, we implement a deep learning algorithm to classify the activity according to the given classes. To increase the number of data samples available for training, we use the Bica cubic Hermite interpolation, which allows us to improve the accuracy of the neural network. The 38 statistical data features were calculated using the rolling update approach and used as input to the classifier. For activity classification, we have adopted the bi-directional long short-term memory (BiLSTM) neural network. The results demonstrate that our system can detect falling with an accuracy of 99.59% (using leave-one-activityout cross-validation) and 97.35% (using leave-one-subject-out cross-validation) considering all activities. When considering only binary classification (falling vs. all other activities), perfect accuracy is achieved.
更多查看译文
关键词
Fall detection, Activity recognition, Smartwatch, Digital health
AI 理解论文
溯源树
样例
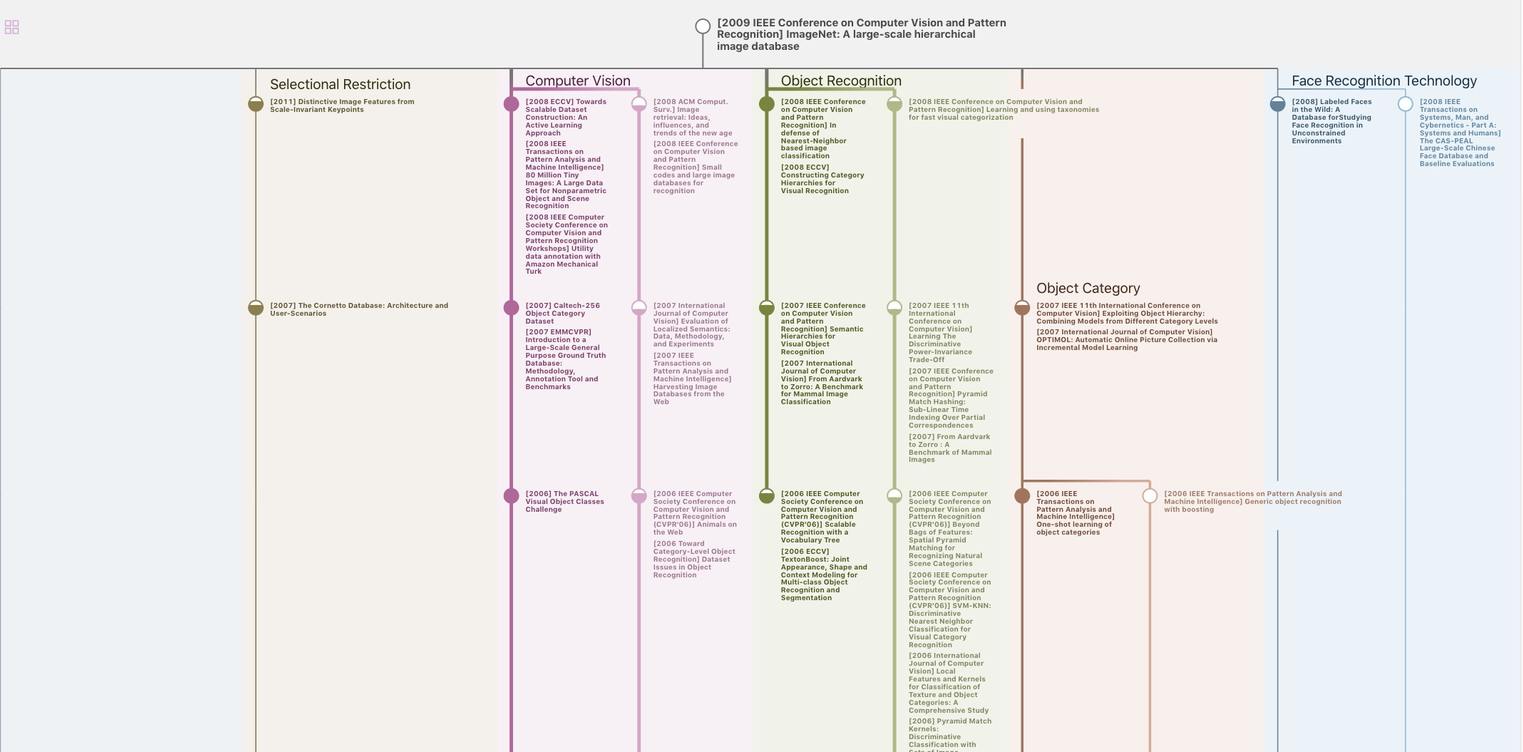
生成溯源树,研究论文发展脉络
Chat Paper
正在生成论文摘要