Research on hybrid feature selection method of power transformer based on fuzzy information entropy
Advanced Engineering Informatics(2021)
摘要
The data of dissolved gas in oil analysis (DGA) is uncertain affected by the influence of transformer capacity and fault location, which makes transformer fault diagnosis model based on DGA has low accuracy. Therefore, we propose a hybrid feature selection method based on fuzzy information entropy, whereby optimizing the reasonable DGA feature parameter according to the feature information between the parameter and fault type, to reduce the influence of DGA data uncertainty on the fault diagnosis accuracy. Firstly, the characteristic relevance and redundancy functions are constructed based on fuzzy information entropy theory. Secondly, these functions are taken as the optimization objectives of binary-chaotic multi-objective particle swarm optimization algorithm(B-CMOPSO), to search for the feature subsets in the feature space composed of 46 DGA feature parameters. Then, the optimal feature subset is selected based on the simulation accuracy of ELM, SVM, Adaboost.M1 and BPNN on the feature subsets. Finally, 30 simulation experiments are carried out to compare with several multi-objective optimization algorithms, common Filter methods and common DGA feature combinations, and the rationality of the proposed method is verified by the t-test method. The results show that the 4 classifiers accuracy means is maximatily improved by 18.95%, 20.77%, 19.85% and 21.27% respectively compared with common DGA feature combinations, indicating that the optimal feature subset preserves more feature information and can effectively reduce the influence of DGA data uncertainty on diagnostic accuracy.
更多查看译文
关键词
Power transformer,DGA,Feature selection,Multi-objective programming,Fuzzy information entropy
AI 理解论文
溯源树
样例
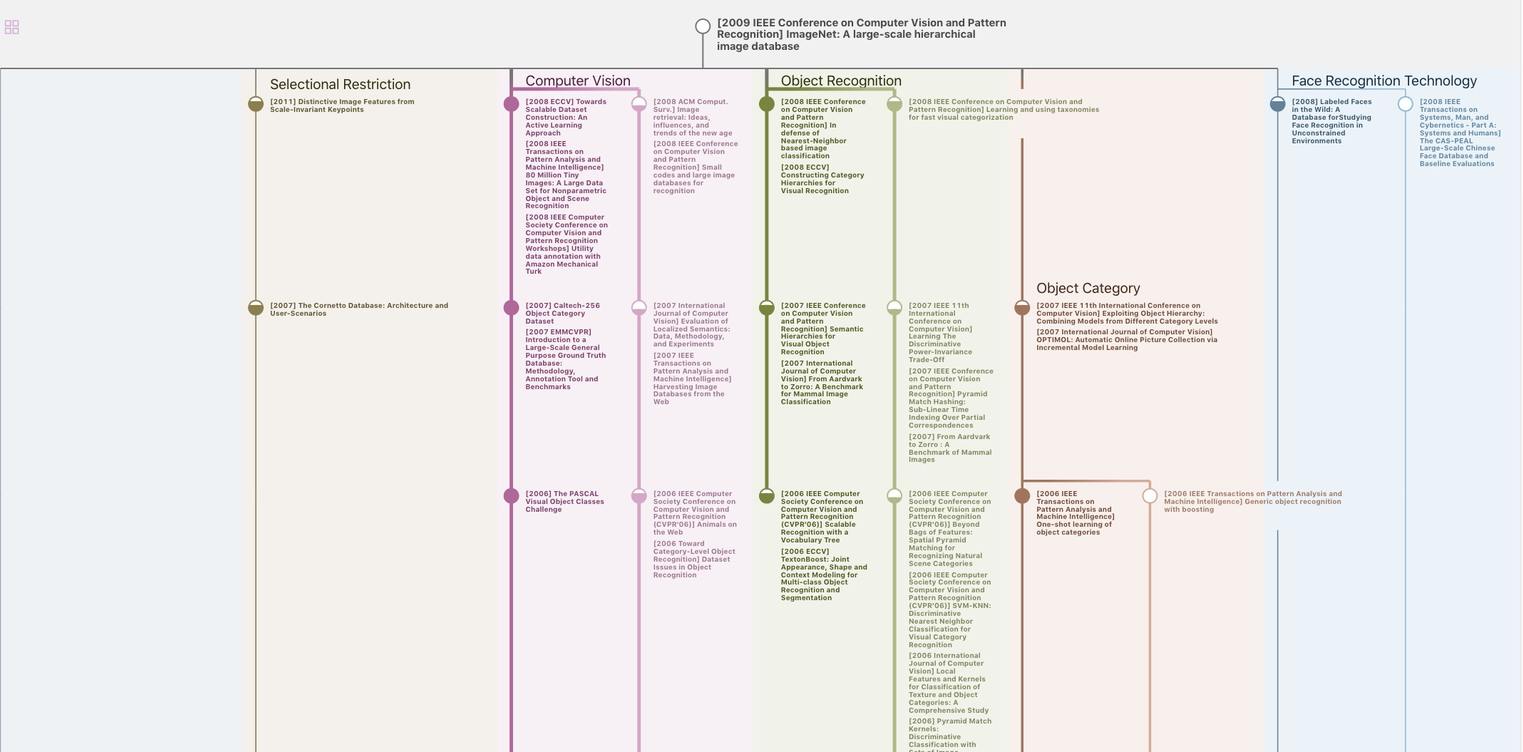
生成溯源树,研究论文发展脉络
Chat Paper
正在生成论文摘要