A parallel splitting ALM-based algorithm for separable convex programming
COMPUTATIONAL OPTIMIZATION AND APPLICATIONS(2021)
摘要
The augmented Lagrangian method (ALM) provides a benchmark for solving the canonical convex optimization problem with linear constraints. The direct extension of ALM for solving the multiple-block separable convex minimization problem, however, is proved to be not necessarily convergent in the literature. It has thus inspired a number of ALM-variant algorithms with provable convergence. This paper presents a novel parallel splitting method for the multiple-block separable convex optimization problem with linear equality constraints, which enjoys a larger step size compared with the existing parallel splitting methods. We first show that a fully Jacobian decomposition of the regularized ALM can contribute a descent direction yielding the contraction of proximity to the solution set; then, the new iterate is generated via a simple correction step with an ignorable computational cost. We establish the convergence analysis for the proposed method, and then demonstrate its numerical efficiency by solving an application problem arising in statistical learning.
更多查看译文
关键词
Convex programming, Augmented Lagrangian method, Jacobian decomposition, Parallel computing, Operator splitting methods
AI 理解论文
溯源树
样例
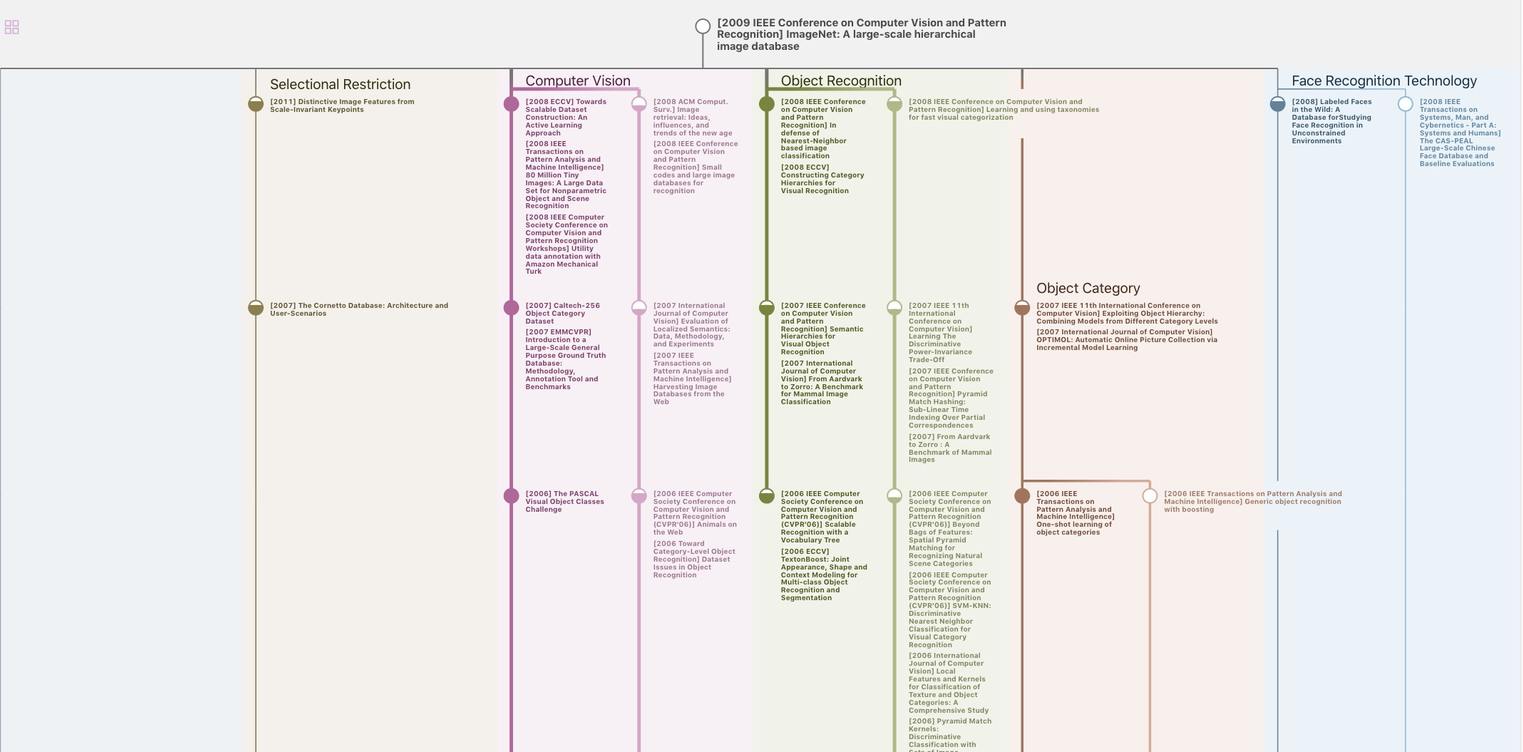
生成溯源树,研究论文发展脉络
Chat Paper
正在生成论文摘要