Environmental Sound Classification Based on Stacked Concatenated DNN using Aggregated Features
JOURNAL OF SIGNAL PROCESSING SYSTEMS FOR SIGNAL IMAGE AND VIDEO TECHNOLOGY(2021)
摘要
In recent years, there has been an increasing interest in Environmental Sound Classification (ESC), and it is a challenging non-speech audio event classification problem because of the complexity of the environment. However, the classification accuracy of the conventional methods is significantly dependent on the robustness of representative features and the effectiveness of the constructed model, which causes the poor adaptability of current models. Considering this, a novel ESC scheme based on stacked Deep Neural Networks with multi-dimensional aggregated features is proposed. Firstly, we use the aggregated features composed of time-domain features and time–frequency (TF) domain features to capture a more comprehensive representation of sounds. Afterward, the feature reduction based on Principal Component Analysis (PCA) is employed to select the most discriminative representations. Finally, a novel Stacked Deep Neural Networks based on ensemble learning and data augmentation is presented to improve the ESC scheme's generalizing capability. The experimental results demonstrate that the proposed method is appropriate for ESC problems, which achieves 96.1% and 98.1% accuracy scores for ESC-10 and UrbanSound8K datasets, respectively, and outperforms most state-of-art methods in ESC tasks at the aspect of both accuracy and computational burden.
更多查看译文
关键词
Environmental Sound Classification, Auditory Feature, Deep Neural Networks, Feature Aggregation
AI 理解论文
溯源树
样例
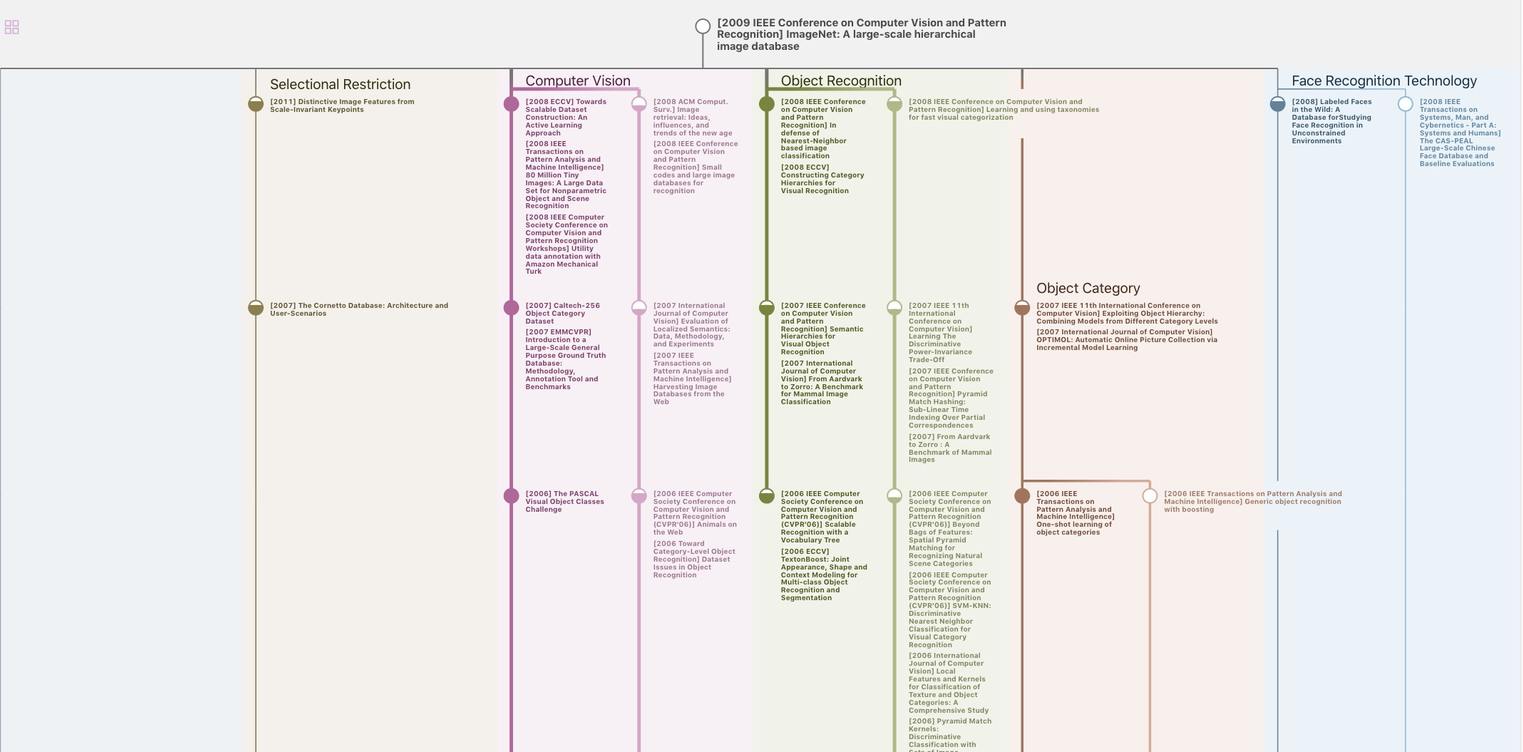
生成溯源树,研究论文发展脉络
Chat Paper
正在生成论文摘要