DeepGlobal - A Global Robustness Verifiable FNN Framework.
SETTA(2021)
摘要
Feed forward neural networks (FNNs) have been deployed in a variety of domains, though achieving great success, also pose severe safety and reliability concerns. Existing adversarial attack generation and automatic verification techniques cannot formally verify a network globally, i.e., finding all adversarial dangerous regions (ADRs) of a network is out of their reach. To address this problem, we develop a global robustness verifiable FNN framework DeepGlobal with three components: 1) a rule-generator finding all potential boundaries of a network by logical reasoning; 2) a new network architecture Sliding Door Network (SDN) enabling rule generation in a feasible way; 3) a selection approach which selects real boundaries from the generated potential boundaries. The ADRs can be further represented by the identified real boundaries. We demonstrate the effectiveness of our approach on both synthetic and real datasets.
更多查看译文
关键词
Feed forward neural networks, Robustness, Global verification
AI 理解论文
溯源树
样例
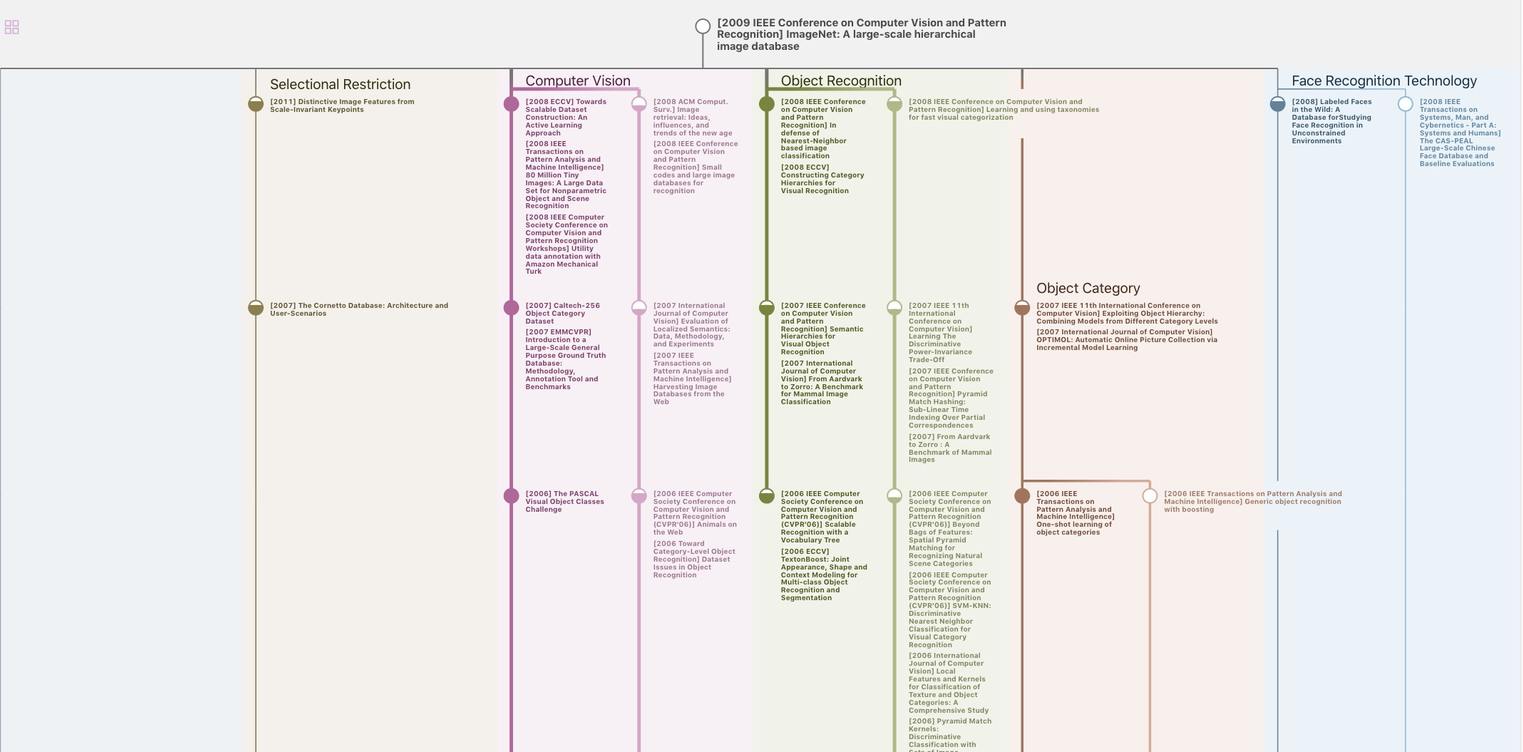
生成溯源树,研究论文发展脉络
Chat Paper
正在生成论文摘要