GC and Other Methods for Full and Partial Context Coverage
KNOWLEDGE-BASED AND INTELLIGENT INFORMATION & ENGINEERING SYSTEMS (KSE 2021)(2021)
摘要
Formal Concept Analysis (FCA) is a popular method for knowledge discovery and data mining in binary data. A shortcoming of FCA is the huge number of formal concepts that may be drawn from formal contexts (binary data tables) of moderate size or larger. A strategy to deal with this shortcoming is to extract a subset of formal concepts that cover the context either fully or partially. We compare different methods for generating full and partial concept cover, present a simple Greedy Coverage (GC) method, and show that it is an efficient option, especially for generating partial concept cover. (C) 2021 The Authors. Published by Elsevier B.V. This is an open access article under the CC BY-NC-ND license (https://crativecommons.org/licenses/by-nc-nd/4.0) Peer-review under responsibility of the scientific committee of KES International.
更多查看译文
关键词
Knowledge Discovery and Data Mining, Data Analysis and Pattern Recognition, Formal Concept Analysis, Knowledge Representation and Management
AI 理解论文
溯源树
样例
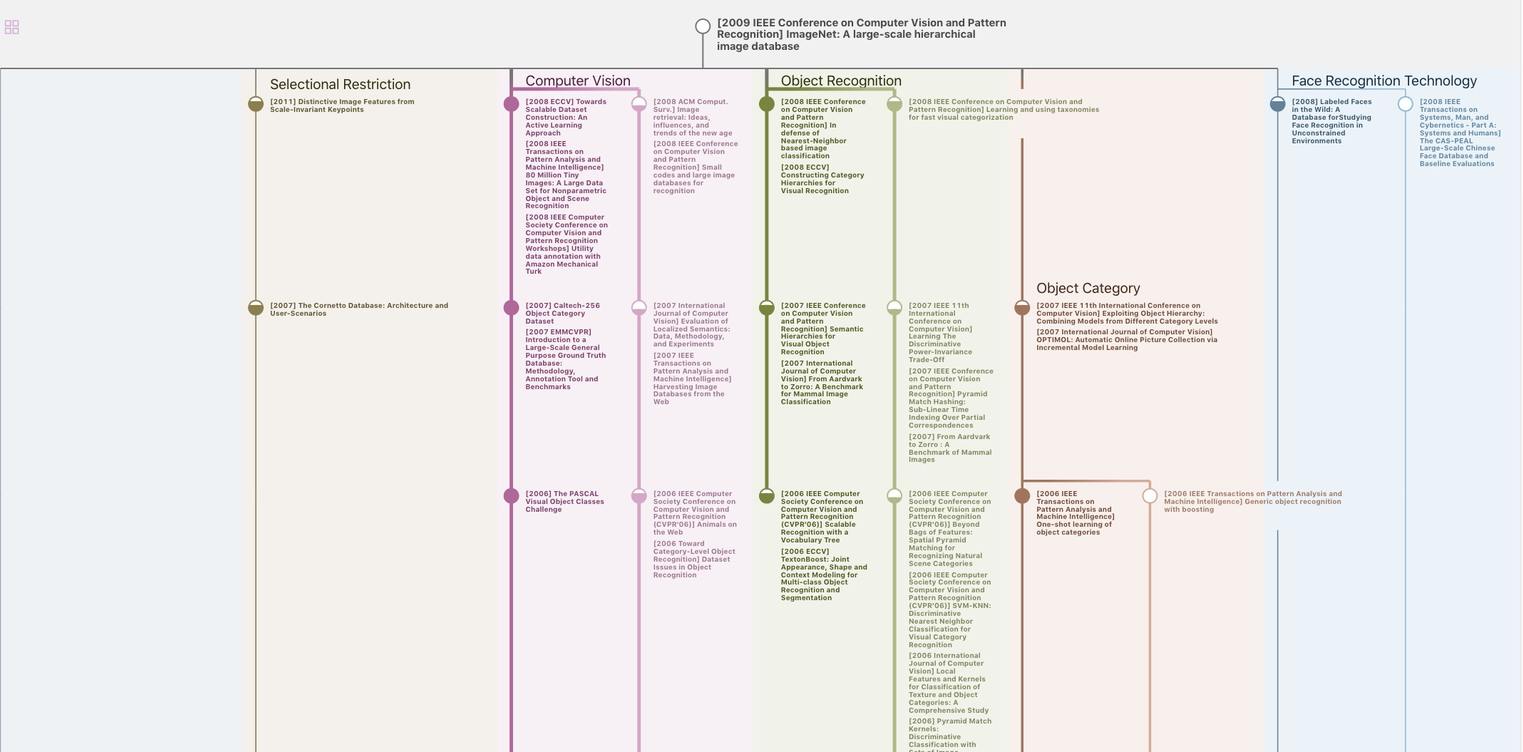
生成溯源树,研究论文发展脉络
Chat Paper
正在生成论文摘要