Leveraging Interpretability - Concept-based Pedestrian Detection with Deep Neural Networks.
CSCS(2021)
摘要
The automation of driving systems relies on proof of the correct functioning of perception. Arguing the safety of deep neural networks (DNNs) must involve quantifiable evidence. Currently, the application of DNNs suffers from an incomprehensible behavior. It is still an open question if post-hoc methods mitigate the safety concerns of trained DNNs. Our work proposes a method for inherently interpretable and concept-based pedestrian detection (CPD). CPD explicitly structures the latent space with concept vectors that learn features for body parts as predefined concepts. The distance-based clustering and separation of latent representations build an interpretable reasoning process. Hence, CPD predicts a body part segmentation based on distances of latent representations to concept vectors. A non-interpretable 2d bounding box prediction for pedestrians complements the segmentation. The proposed CPD generates additional information that can be of great value in a safety argumentation of a DNN for pedestrian detection. We report competitive performance for the task of pedestrian detection. Finally, CPD enables concept-based tests to quantify evidence of a safe perception in automated driving systems.
更多查看译文
AI 理解论文
溯源树
样例
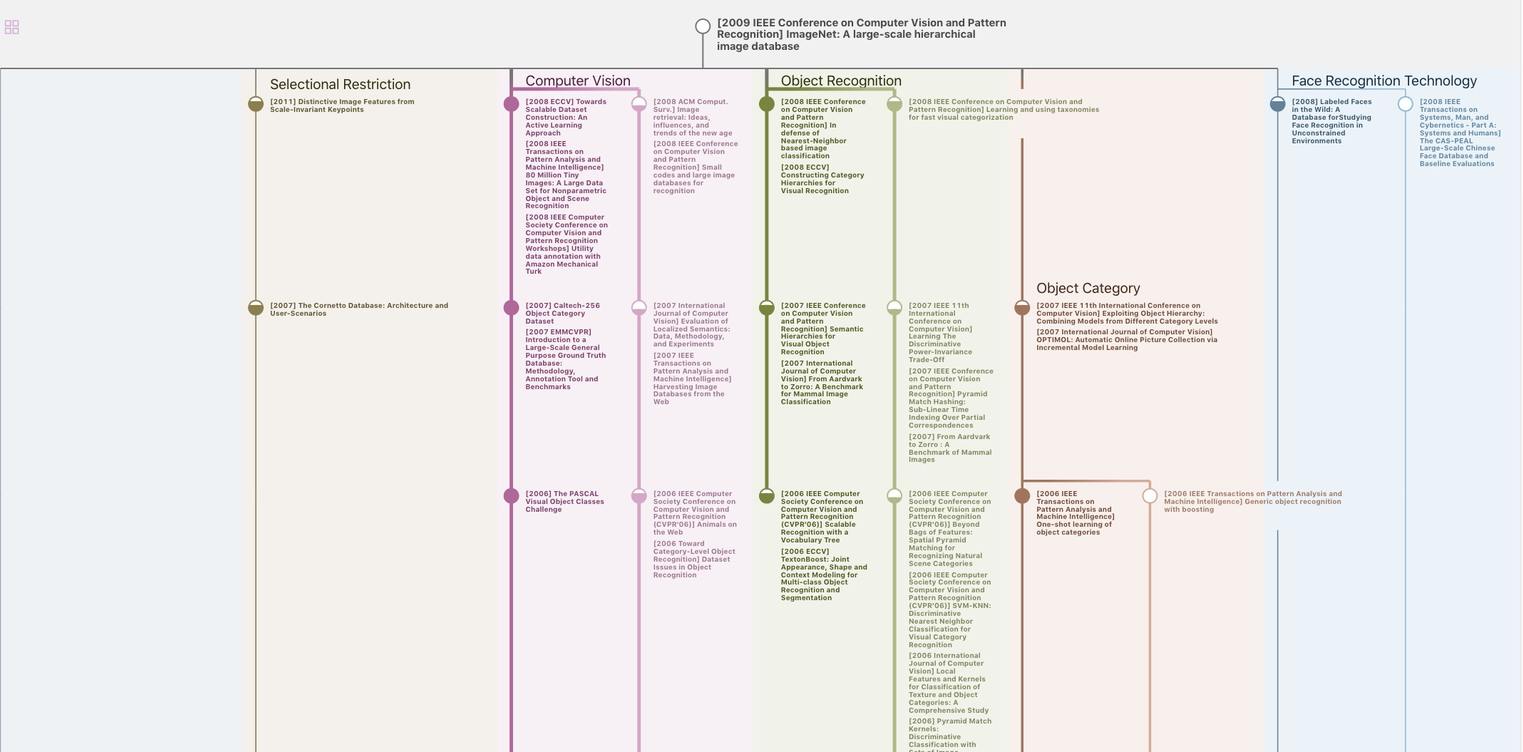
生成溯源树,研究论文发展脉络
Chat Paper
正在生成论文摘要