Combining Model-Based and Model-Free Reinforcement Learning Policies for More Efficient Sepsis Treatment
ISBRA(2021)
摘要
Sepsis is the main cause of mortality in intensive care units (ICUs), but the optimal treatment strategy still remains unclear. Managing the treatment of sepsis is challenging because individual patients respond differently to the treatment, thus calling for a pressing need of personalized treatment strategies. Reinforcement learning (RL) has been widely used to learn optimal strategies for sepsis treatment, especially for the administration of intravenous fluids and vasopressors. RL can be generally categorized into two types of approaches: the model-based and the model-free approaches. It has been shown that model-based approaches, with the prerequisite of accurate estimation of environment models, are more sample efficient than model-free approaches, but at the same time can only achieve inferior asymptotic performance. In this paper, we propose a policy mixture framework to make the best of both model-based and model-free RL approaches to achieve more efficient personalized sepsis treatment. We demonstrate that the policy derived from our framework outperforms policies prescribed by physicians, model-based only methods, and model-free only approaches.
更多查看译文
关键词
Sepsis,ICU,Reinforcement learning,Model-based,Model-free,Policy mixture
AI 理解论文
溯源树
样例
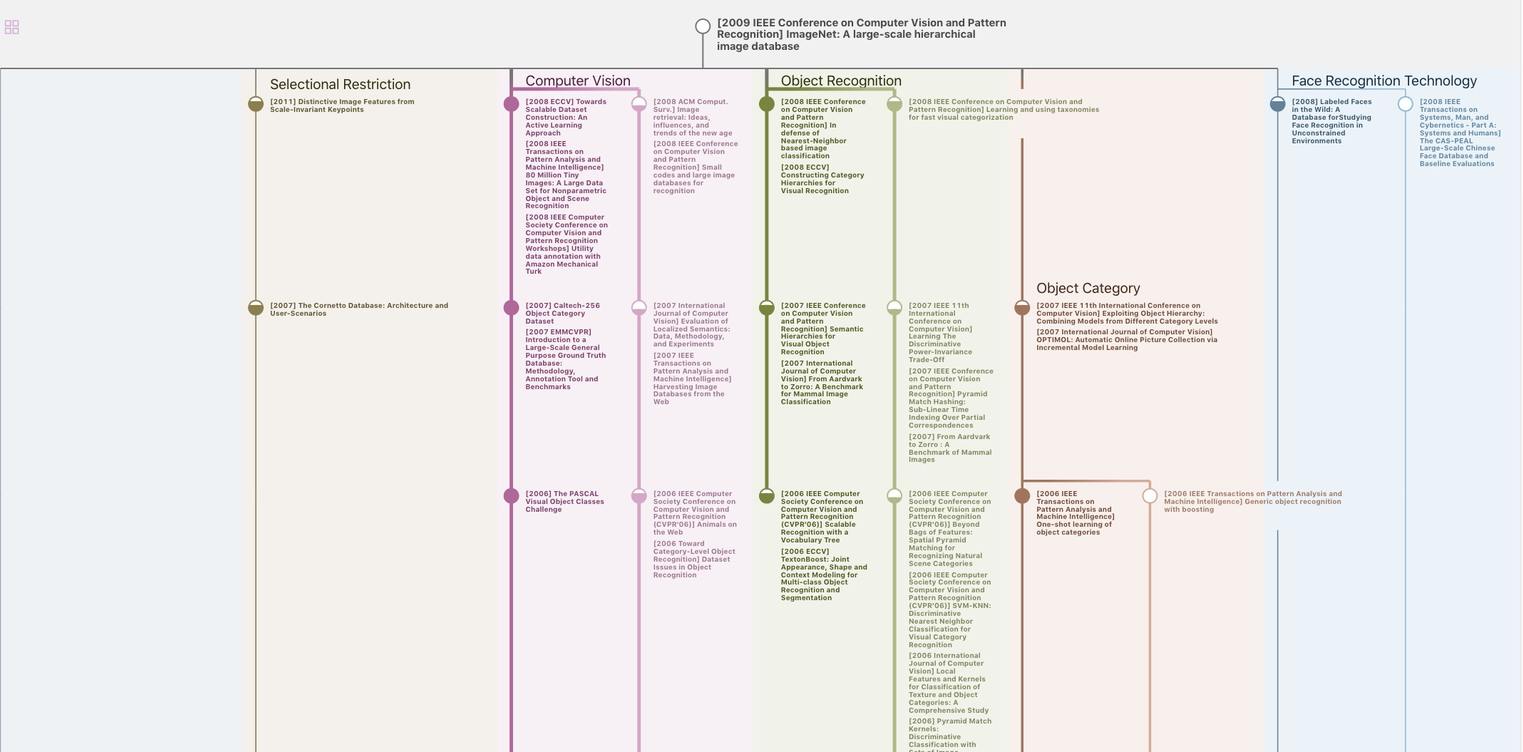
生成溯源树,研究论文发展脉络
Chat Paper
正在生成论文摘要