Tracking urban heartbeat and policy compliance through vision and language-based sensing
SENSYS(2021)
摘要
ABSTRACTSensing activities at the city scale using big data can enable applications to improve the quality of citizen life. While there are approaches to sense the urban heartbeat using sound, vision, radio frequency (RF), and other sensors, capturing changes at urban scale using such sensing modalities is challenging. Due to the enormous amount of data they produce and the associated annotation and processing requirement, such data can be of limited use. In this paper, we present a vision-to-language modeling approach to capture patterns and transitions that occur in New York City from March 2020 to August 2020. We use the model on ~1 million street images captured by dashcams over 6 months. We then use the captions to train a language model based on Latent Dirichlet Allocation [4] and compare models from different periods using probabilistic distance measures. We observe distribution shifts in the model that correlate well with social distancing policies and are corroborated by different data sources, such as mobility traces. This language-based sensing introduces a new sensing modality to capture dynamics in the city with lower storage requirements and privacy concerns.
更多查看译文
AI 理解论文
溯源树
样例
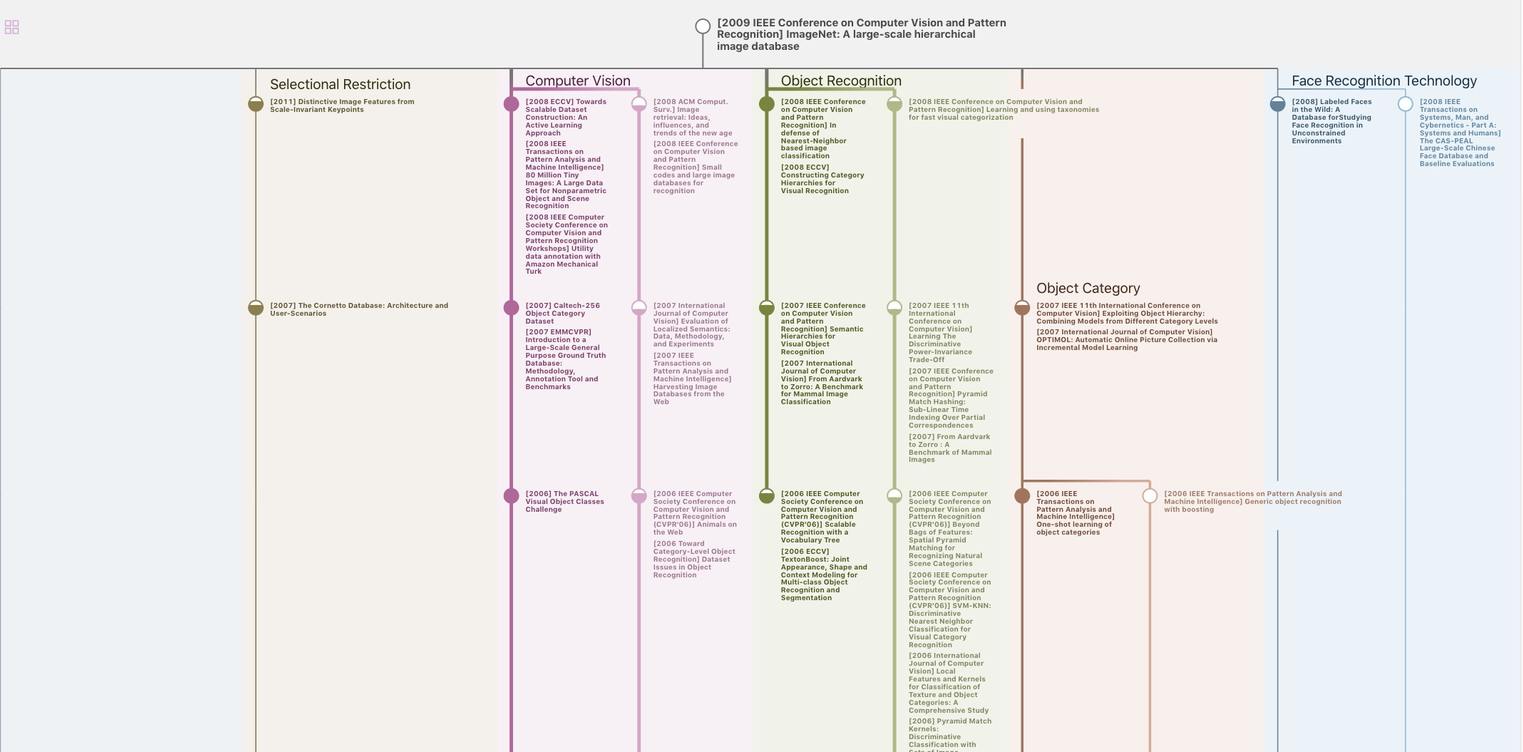
生成溯源树,研究论文发展脉络
Chat Paper
正在生成论文摘要