SCHMEAR: scalable construction of holistic models for energy analysis from rooftops
Embedded Network Sensor Systems(2021)
摘要
ABSTRACTAs the world moves to decarbonize, the built environment commands attention for its intensity of energy consumption. Potential pathways for decarbonizing the built environment can be discovered through the aid of building energy modeling, which helps identify potential retrofit strategies and simulate integration with renewable energy sources. Energy modeling is complicated however, due to compound interactions between building materials, structural design, and urban form. Significant domain knowledge, modeling expertise, and extensive time investment are required for accurate modeling to accommodate this complexity. In this work, we explore the potential of accurately modeling building energy consumption at scale through the application of modern computer vision algorithms. We demonstrate that our computer vision system can accurately predict energy consumption through the extraction of meaningful features contained in satellite imagery. To accomplish this, we introduce a data-collection pipeline and a computer vision architecture to process satellite photos and contextual information from the urban texture. We also demonstrate a method of comparing the relative significance of the automatically extracted features in informing building decarbonization decision making and policy. Our results indicate that this approach reveals valuable insights into the dynamics of building energy consumption on the city scale and enables the rapid analysis of urban energy dynamics with readily available data.
更多查看译文
关键词
Convolutional Neural Network, Building Energy Modelling, Feature Extraction, Computer Vision
AI 理解论文
溯源树
样例
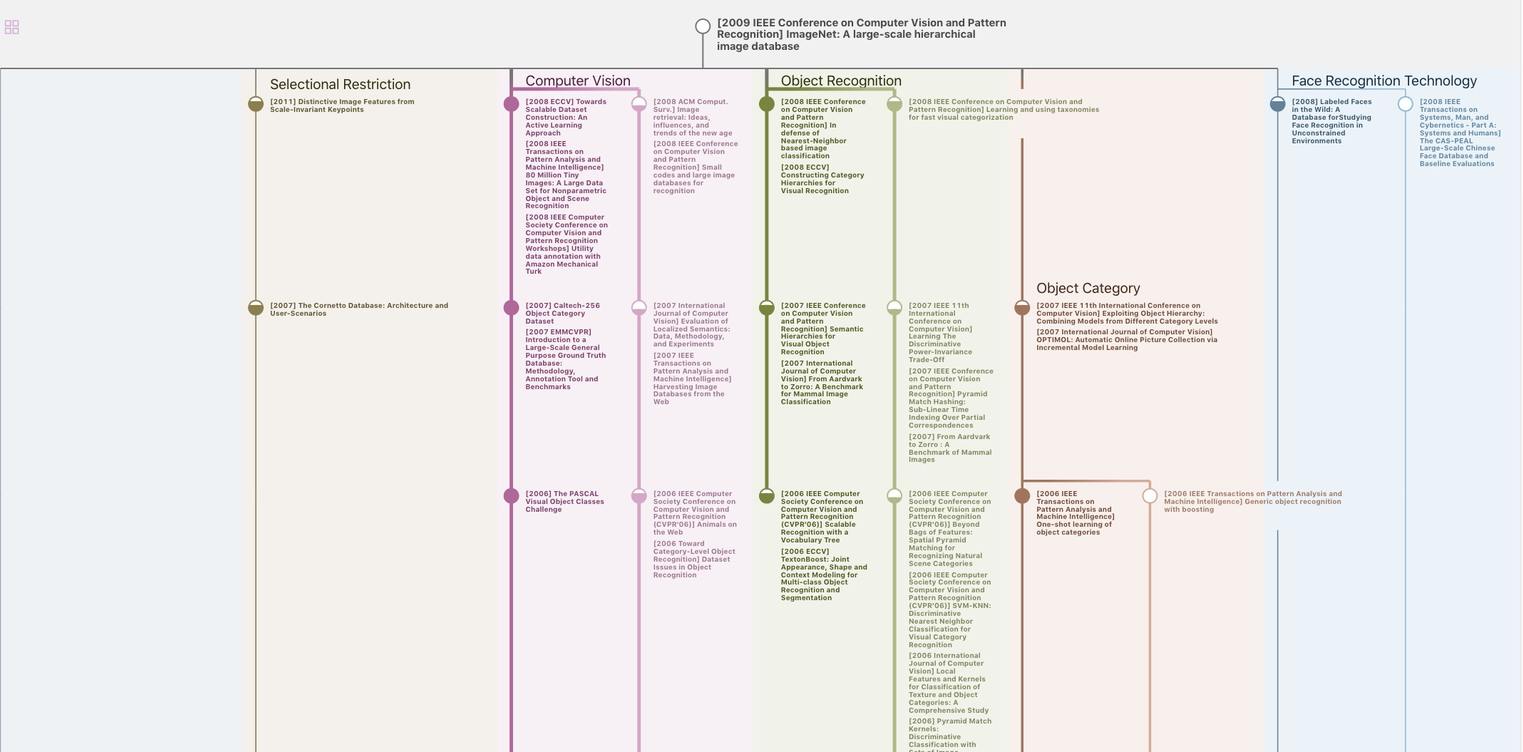
生成溯源树,研究论文发展脉络
Chat Paper
正在生成论文摘要