Approximating Length-Restricted Means Under Dynamic Time Warping
Approximation and Online Algorithms(2022)
摘要
We study variants of the mean problem under the p-Dynamic Time Warping (p-DTW) distance, a popular and robust distance measure for sequential data. In our setting we are given a set of finite point sequences over an arbitrary metric space and we want to compute a mean point sequence of given length that minimizes the sum of p-DTW distances, each raised to the qth power, between the input sequences and the mean sequence. In general, the problem is
$$\textrm{NP}$$
-hard and known not to be fixed-parameter tractable in the number of sequences. On the positive side, we show that restricting the length of the mean sequence significantly reduces the hardness of the problem. We give an exact algorithm running in polynomial time for constant-length mean s. We explore various approximation algorithms that provide a trade-off between the approximation factor and the running time. Our approximation algorithms have a running time with only linear dependency on the number of input sequences. In addition, we use our mean algorithms to obtain clustering algorithms with theoretical guarantees.
更多查看译文
AI 理解论文
溯源树
样例
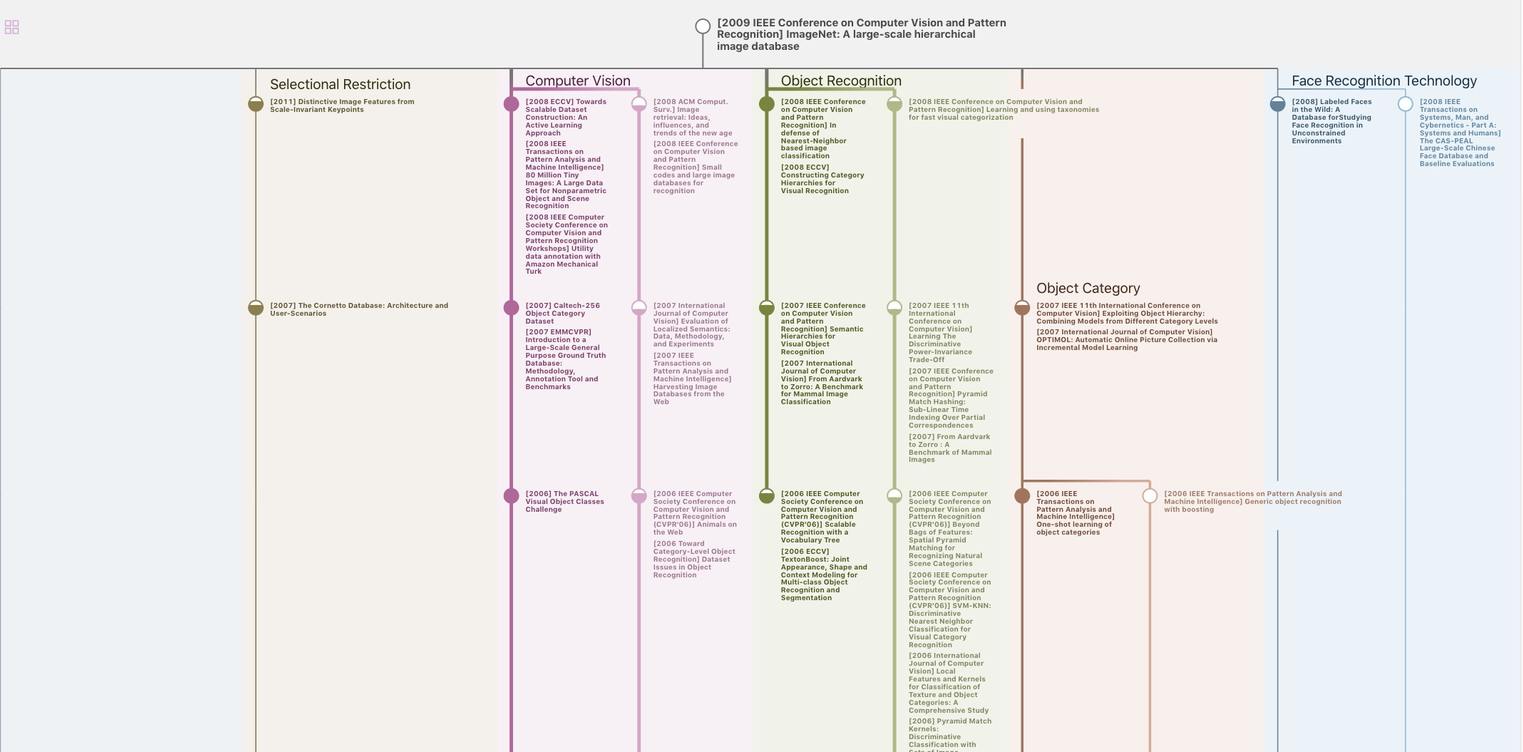
生成溯源树,研究论文发展脉络
Chat Paper
正在生成论文摘要