Comparisons Where It Matters: Using Layer-Wise Regularization to Improve Federated Learning on Heterogeneous Data
APPLIED SCIENCES-BASEL(2022)
摘要
Federated Learning is a widely adopted method for training neural networks over distributed data. One main limitation is the performance degradation that occurs when data are heterogeneously distributed. While many studies have attempted to address this problem, a more recent understanding of neural networks provides insight to an alternative approach. In this study, we show that only certain important layers in a neural network require regularization for effective training. We additionally verify that Centered Kernel Alignment (CKA) most accurately calculates similarities between layers of neural networks trained on different data. By applying CKA-based regularization to important layers during training, we significantly improved performances in heterogeneous settings. We present FedCKA, a simple framework that outperforms previous state-of-the-art methods on various deep learning tasks while also improving efficiency and scalability.
更多查看译文
关键词
federated learning, heterogeneity, non-IID, regularization, layer-wise similarity
AI 理解论文
溯源树
样例
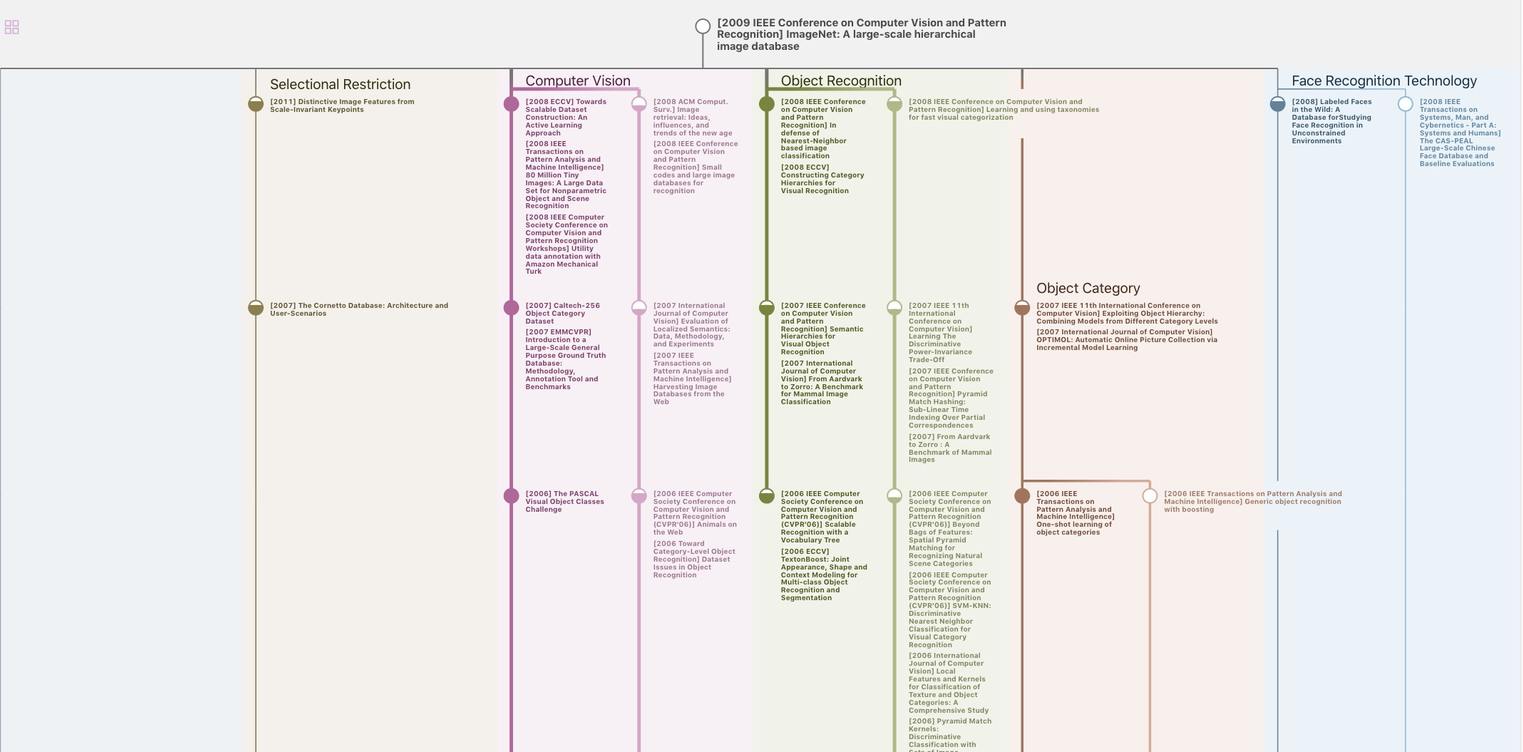
生成溯源树,研究论文发展脉络
Chat Paper
正在生成论文摘要