STEM: Unsupervised STructural EMbedding for Stance Detection.
AAAI Conference on Artificial Intelligence(2022)
摘要
Stance detection is an important task, supporting many downstream tasks such as discourse parsing and modeling the propagation of fake news, rumors, and science denial. In this paper, we propose a novel framework for stance detection. Our framework is unsupervised and domain-independent. Given a claim and a multi-participant discussion -- we construct the interaction network from which we derive topological embedding for each speaker. These speaker embedding enjoy the following property: speakers with the same stance tend to be represented by similar vectors, while antipodal vectors represent speakers with opposing stances. These embedding are then used to divide the speakers into stance-partitions. We evaluate our method on three different datasets from different platforms. Our method outperforms or is comparable with supervised models while providing confidence levels for its output. Furthermore, we demonstrate how the structural embedding relate to the valence expressed by the speakers. Finally, we discuss some limitations inherent to the framework.
更多查看译文
关键词
Speech & Natural Language Processing (SNLP),Data Mining & Knowledge Management (DMKM)
AI 理解论文
溯源树
样例
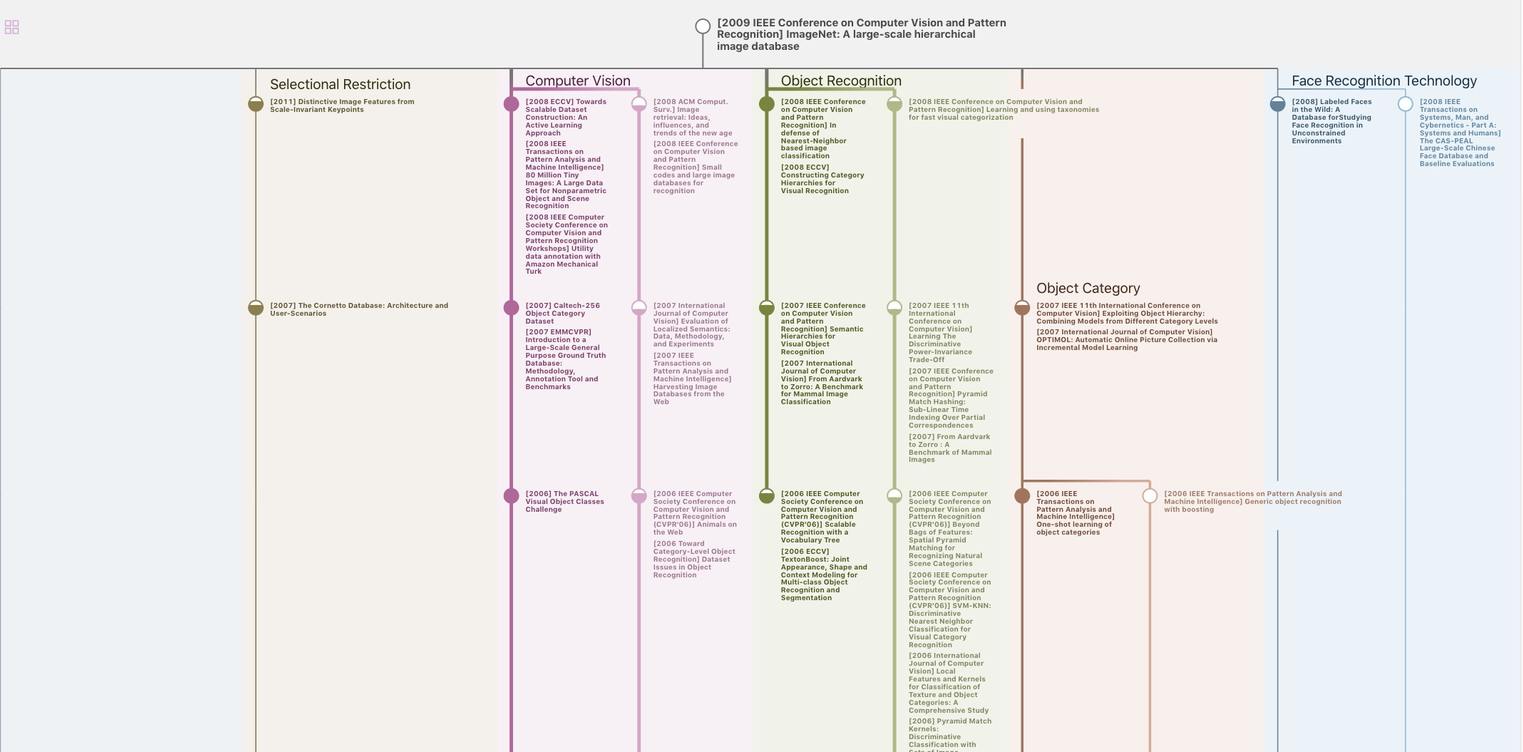
生成溯源树,研究论文发展脉络
Chat Paper
正在生成论文摘要