Effective Meta-Regularization by Kernelized Proximal Regularization.
Neural Information Processing Systems(2021)
摘要
We study the problem of meta-learning, which has proved to be advantageous to accelerate learning new tasks with a few samples. The recent approaches based on deep kernels achieve the state-of-the-art performance. However, the regularizers in their base learners are not learnable. In this paper, we propose an algorithm called MetaProx to learn a proximal regularizer for the base learner. We theoretically establish the convergence of MetaProx. Experimental results confirm the advantage of the proposed algorithm.
更多查看译文
AI 理解论文
溯源树
样例
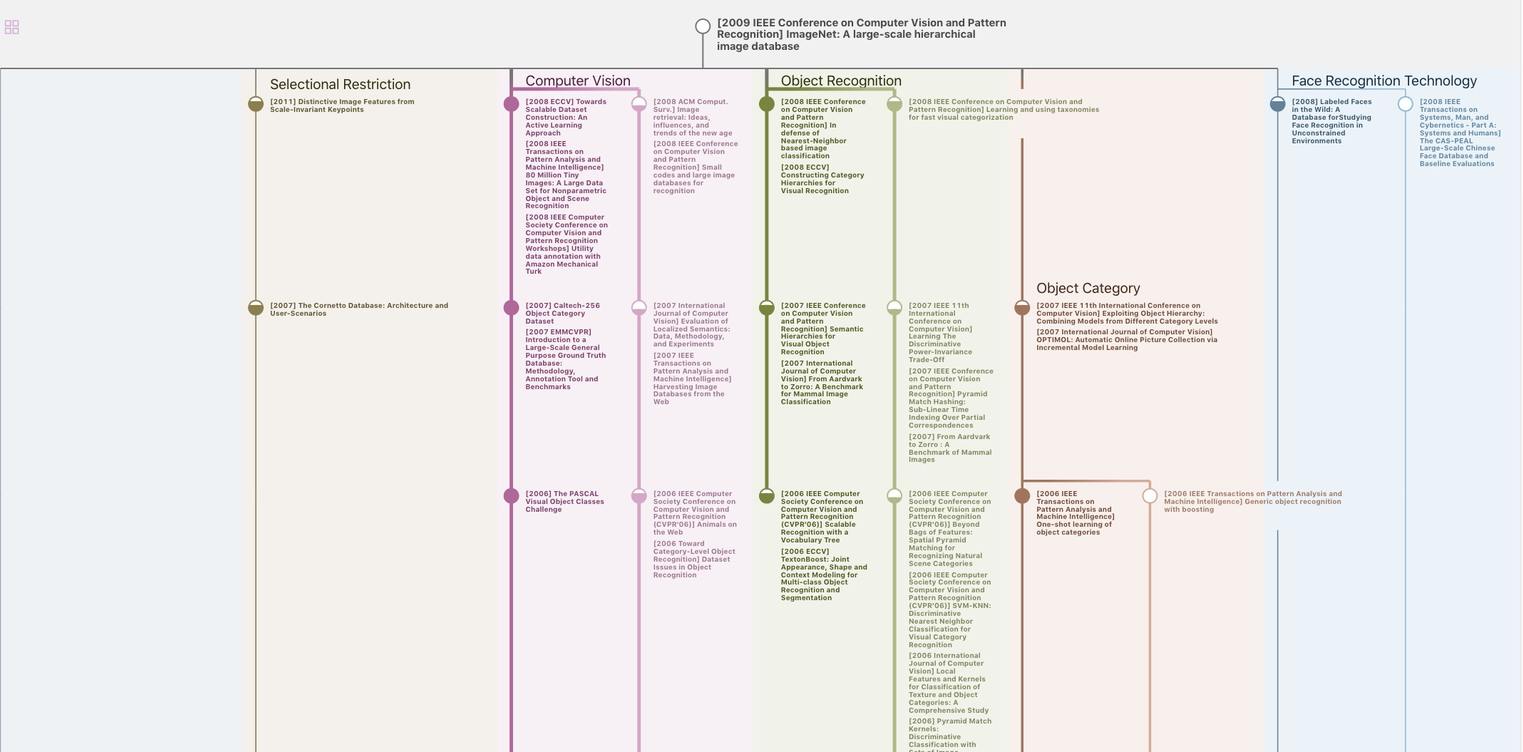
生成溯源树,研究论文发展脉络
Chat Paper
正在生成论文摘要