Sequential Causal Imitation Learning with Unobserved Confounders.
Annual Conference on Neural Information Processing Systems(2021)
摘要
"Monkey see monkey do" is an age-old adage, referring to naive imitation without a deep understanding of a system's underlying mechanics. Indeed, if a demonstrator has access to information unavailable to the imitator (monkey), such as a different set of sensors, then no matter how perfectly the imitator models its perceived environment (See), attempting to directly reproduce the demonstrator's behavior (Do) can lead to poor outcomes. Imitation learning in the presence of a mismatch between demonstrator and imitator has been studied in the literature under the rubric of causal imitation learning (Zhang et. al. 2020), but existing solutions are limited to single-stage decision-making. This paper investigates the problem of causal imitation learning in sequential settings, where the imitator must make multiple decisions per episode. We develop a graphical criterion that is both necessary and sufficient for determining the feasibility of causal imitation, providing conditions when an imitator can match a demonstrator's performance despite differing capabilities. Finally, we provide an efficient algorithm for determining imitability, and corroborate our theory with simulations.
更多查看译文
关键词
unobserved confounders,learning
AI 理解论文
溯源树
样例
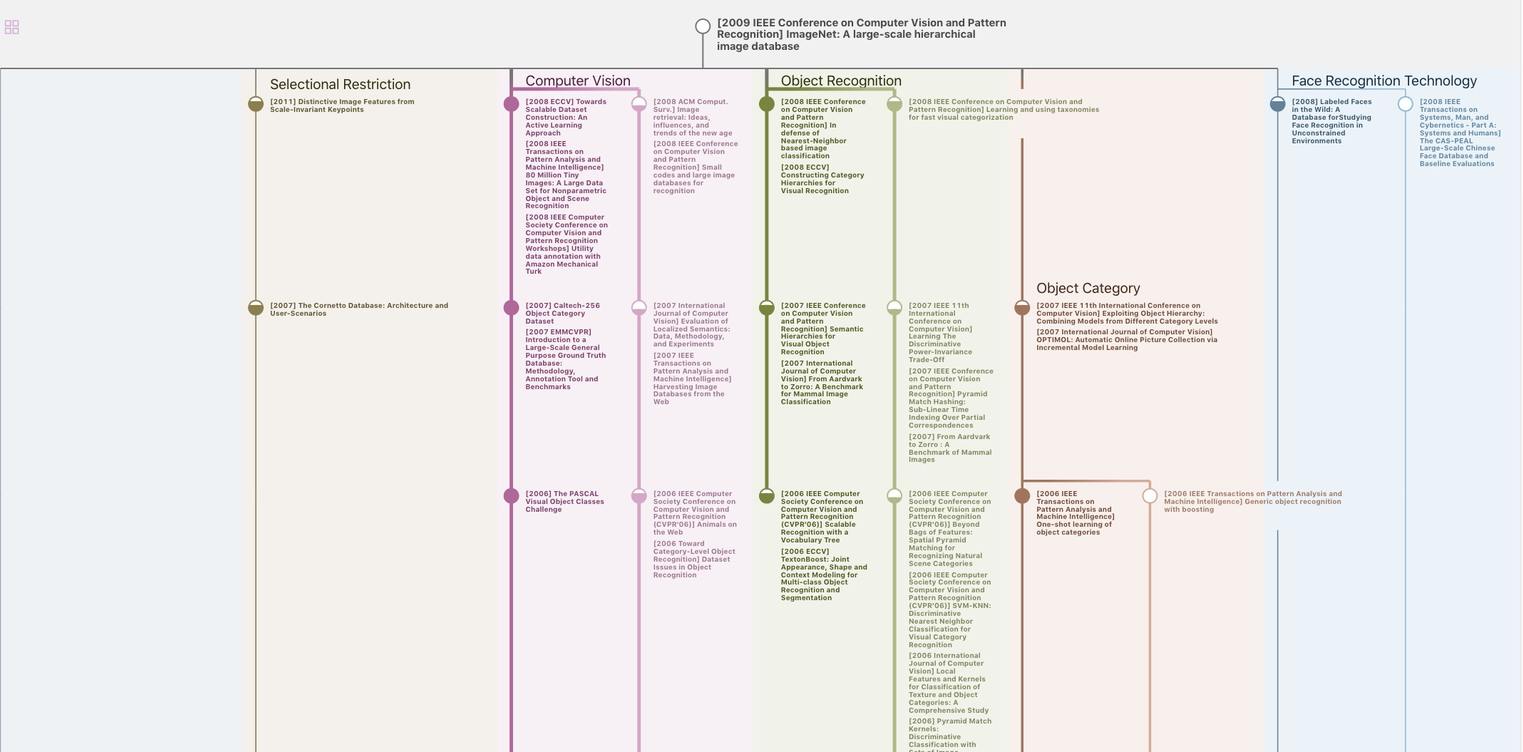
生成溯源树,研究论文发展脉络
Chat Paper
正在生成论文摘要