Implicit Task-Driven Probability Discrepancy Measure for Unsupervised Domain Adaptation.
PubMed(2021)
摘要
Probability discrepancy measure is a fundamental construct for numerous machine learning models such as weakly supervised learning and generative modeling. However, most measures overlook the fact that the distributions are not the end-product of learning, but are the input of a downstream predictor. Therefore, it is important to warp the probability discrepancy measure towards the end tasks, and towards this goal, we propose a new bi-level optimization based approach so that the two distributions are compared not uniformly against the entire hypothesis space, but only with respect to the optimal predictor for the downstream end task. When applied to margin disparity discrepancy and contrastive domain discrepancy, our method significantly improves the performance in unsupervised domain adaptation, and enjoys a much more principled training process.
更多查看译文
AI 理解论文
溯源树
样例
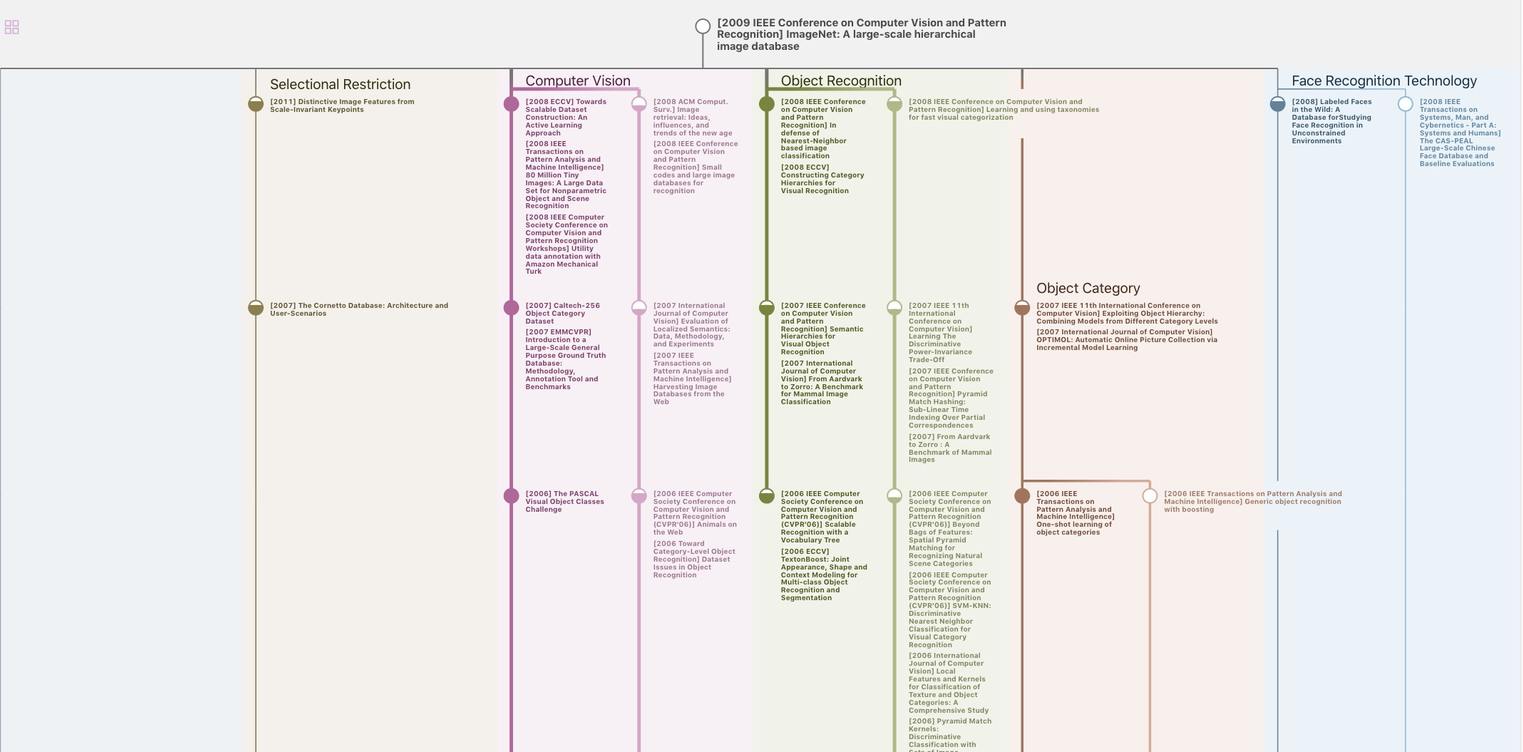
生成溯源树,研究论文发展脉络
Chat Paper
正在生成论文摘要