Quantum Deep Descriptor: Physically Informed Transfer Learning from Small Molecules to Polymers
JOURNAL OF CHEMICAL THEORY AND COMPUTATION(2021)
摘要
In this study, we propose a physically informed transfer learning approach for materials informatics (MI) using a quantum deep descriptor (QDD) obtained from the quantum deep field (QDF). The QDF is a machine learning model based on density functional theory (DFT) and can be trained with a large database of molecular properties. The pre-trained QDF model can provide an effective molecular descriptor that encodes the fundamental quantum-chemical characteristics (i.e., the wave function or orbital, electron density, and energies of a molecule) learned from the large database; we refer to this descriptor as a QDD. We show that a QDD pre-trained with certain properties of small molecules can predict different properties (e.g., the band gap and dielectric constant) of polymers compared with some existing descriptors. We believe that our DFT-based, physically informed transfer learning approach will not only be useful for practical applications in MI but will also provide quantum-chemical insights into materials in the future. All codes used in this study are available at https: //github.com/masashitsubaki.
更多查看译文
AI 理解论文
溯源树
样例
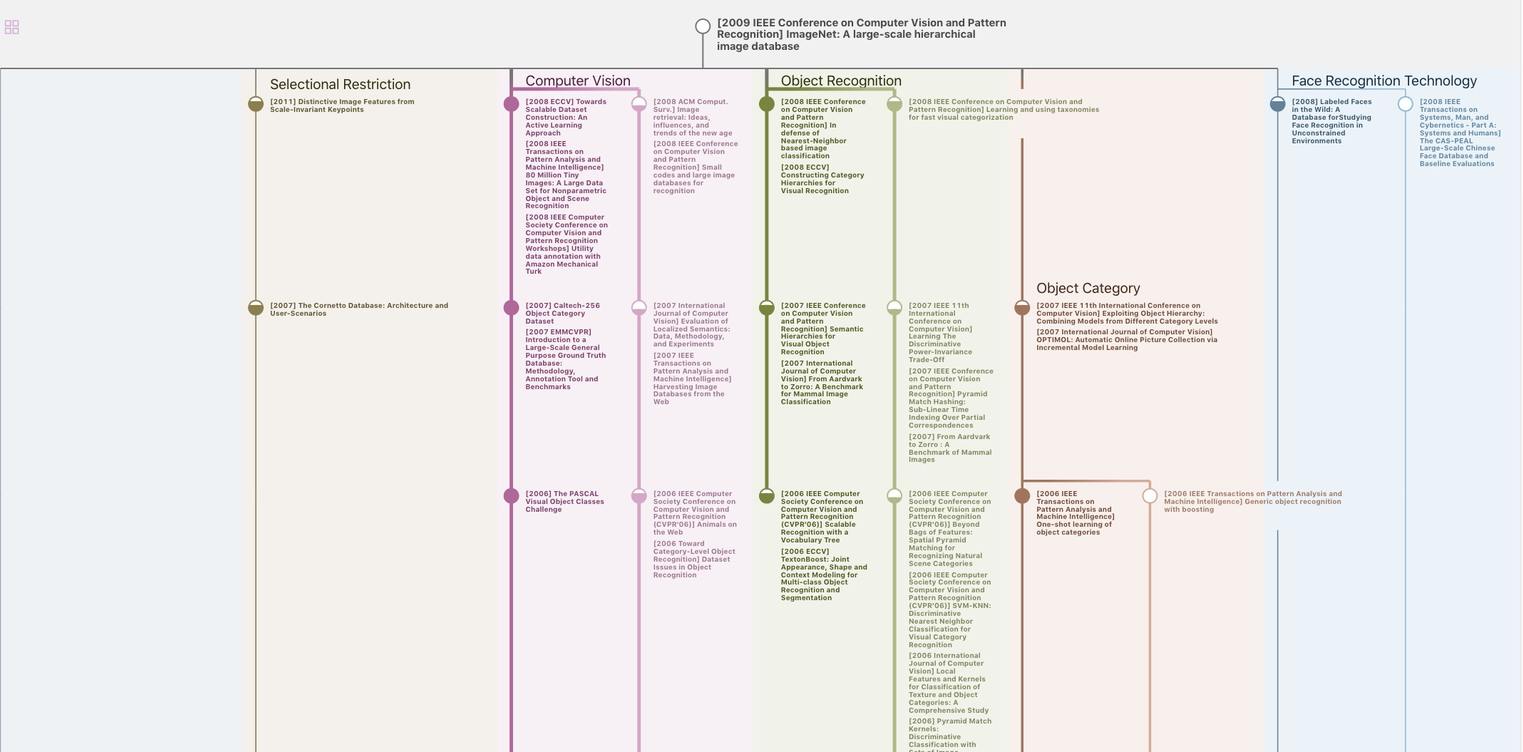
生成溯源树,研究论文发展脉络
Chat Paper
正在生成论文摘要