Video-Text Pre-training with Learned Regions for Retrieval.
arxiv(2023)
摘要
Video-Text pre-training aims at learning transferable representations from large-scale video-text pairs via aligning the semantics between visual and textual information. State-of-the-art approaches extract visual features from raw pixels in an end-to-end fashion. However, these methods operate at frame-level directly and thus overlook the spatio-temporal structure of objects in video, which yet has a strong synergy with nouns in textual descriptions. In this work, we propose a simple yet effective module for video-text representation learning, namely RegionLearner , which can take into account the structure of objects during pre-training on large-scale video-text pairs. Given a video, our module (1) first quantizes continuous visual features via clustering patch-features into the same cluster according to content similarity, then (2) generates learnable masks to aggregate fragmentary features into regions with complete semantics, and finally (3) models the spatio-temporal dependencies between different semantic regions. In contrast to using off-the-shelf object detectors. our proposed module does not require explicit supervision and is much more computationally efficient. We pre-train the proposed approach on the public WebVid2M and CC3M datasets. Extensive evaluations on four downstream video-text retrieval benchmarks clearly demonstrate the effectiveness of our RegionLearner.
更多查看译文
关键词
regions,video-text,pre-training
AI 理解论文
溯源树
样例
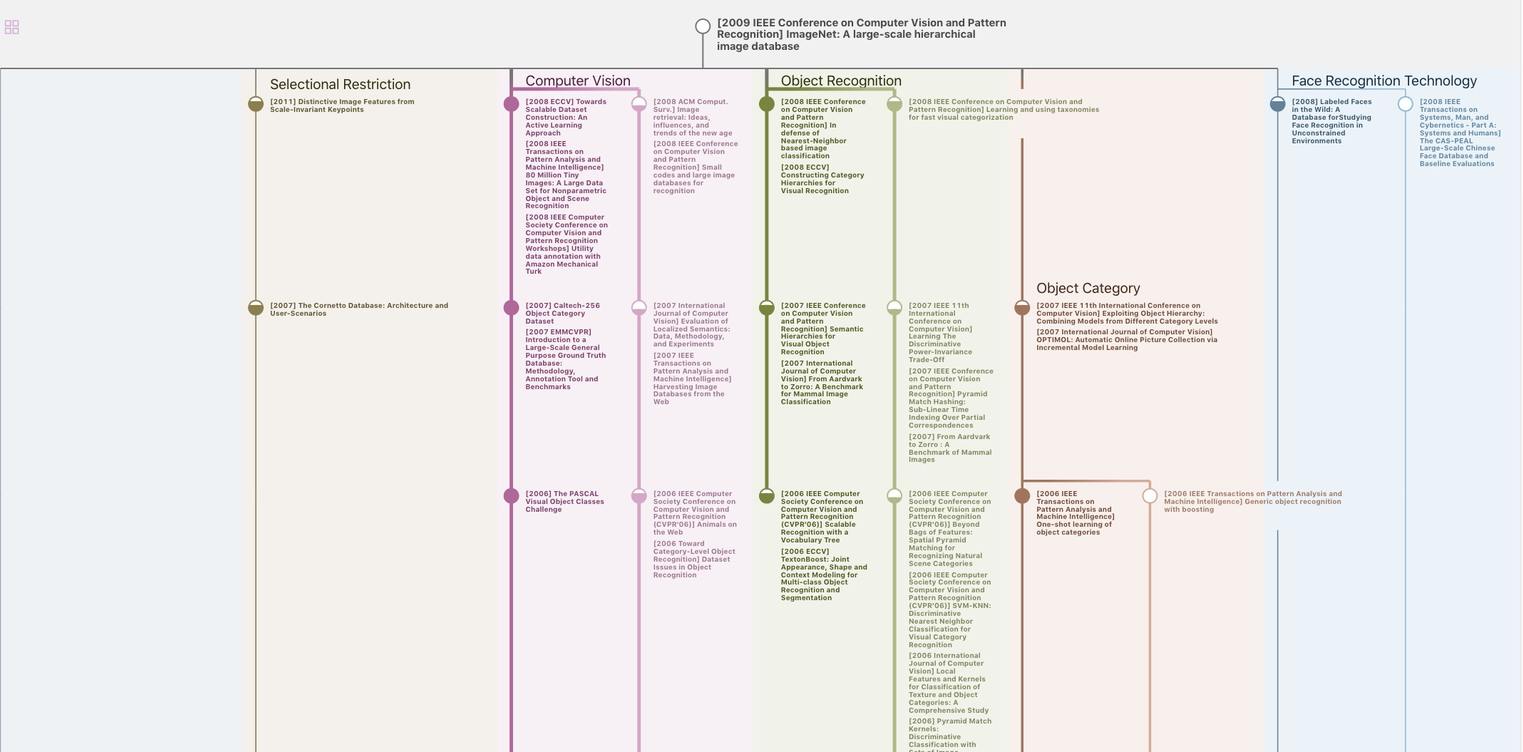
生成溯源树,研究论文发展脉络
Chat Paper
正在生成论文摘要