Quantifying the uncertainty of neural networks using Monte Carlo dropout for deep learning based quantitative MRI
arxiv(2021)
摘要
Dropout is conventionally used during the training phase as regularization method and for quantifying uncertainty in deep learning. We propose to use dropout during training as well as inference steps, and average multiple predictions to improve the accuracy, while reducing and quantifying the uncertainty. The results are evaluated for fractional anisotropy (FA) and mean diffusivity (MD) maps which are obtained from only 3 direction scans. With our method, accuracy can be improved significantly compared to network outputs without dropout, especially when the training dataset is small. Moreover, confidence maps are generated which may aid in diagnosis of unseen pathology or artifacts.
更多查看译文
关键词
monte carlo dropout,deep learning,mri,neural networks,uncertainty
AI 理解论文
溯源树
样例
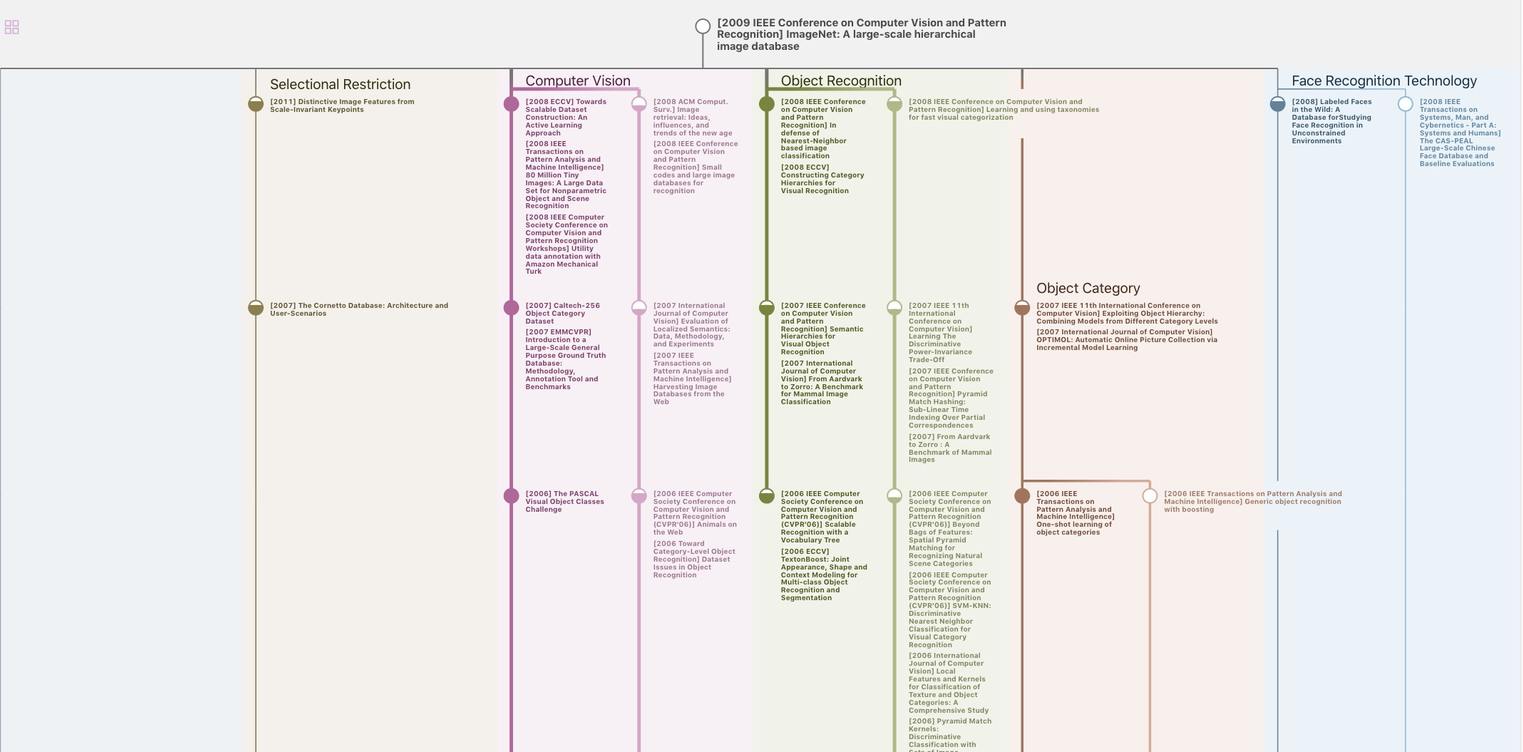
生成溯源树,研究论文发展脉络
Chat Paper
正在生成论文摘要