BenchML: an extensible pipelining framework for benchmarking representations of materials and molecules at scale
arxiv(2021)
摘要
We introduce a machine-learning (ML) framework for high-throughput benchmarking of diverse representations of chemical systems against datasets of materials and molecules. The guiding principle underlying the benchmarking approach is to evaluate raw descriptor performance by limiting model complexity to simple regression schemes while enforcing best ML practices, allowing for unbiased hyperparameter optimization, and assessing learning progress through learning curves along series of synchronized train-test splits. The resulting models are intended as baselines that can inform future method development, next to indicating how easily a given dataset can be learnt. Through a comparative analysis of the training outcome across a diverse set of physicochemical, topological and geometric representations, we glean insight into the relative merits of these representations as well as their interrelatedness.
更多查看译文
关键词
benchmarking,representations,machine learning,chemistry and materials
AI 理解论文
溯源树
样例
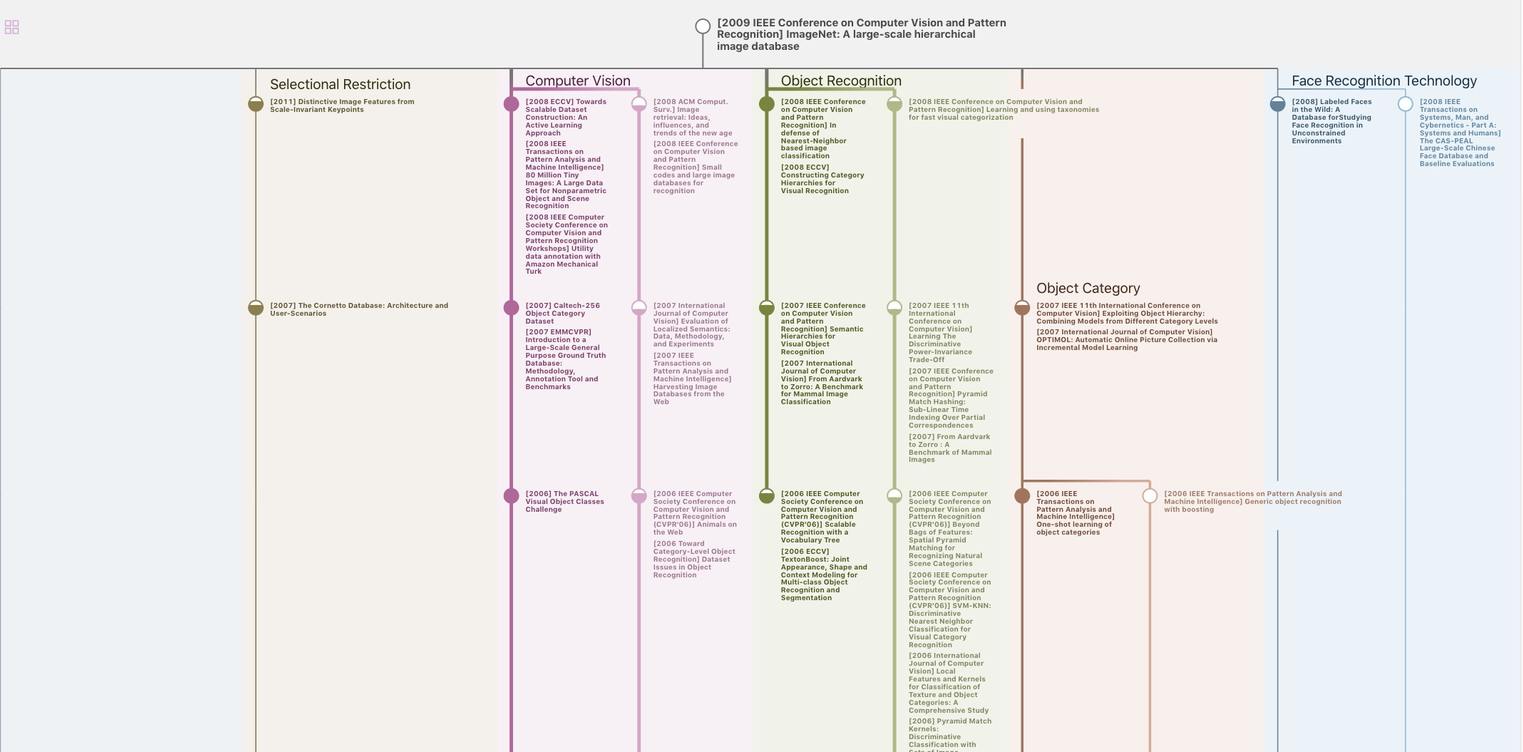
生成溯源树,研究论文发展脉络
Chat Paper
正在生成论文摘要