LTT-GAN: Looking Through Turbulence by Inverting GANs
arxiv(2023)
摘要
In many applications of long-range imaging, such as surveillance, we are faced with a scenario where we have to recover and identify facial images appearing in the captured imagery degraded by atmospheric turbulence. One way to deal with this problem is to develop methods that can remove the effect of turbulence from images. However, restoring images degraded by atmospheric turbulence is difficult since it causes images to be geometrically distorted and blurry. To mitigate the effect of turbulence, we propose a method in which well-trained Generative Adversarial Networks (GANs) are leveraged as effective priors. Different from the existing GAN inversion models, it learns to restore images in the context of semantic space that is represented by a set of augmented images with the same identity but different appearances. Such a context can enforce the network to learn to preserve identity while producing visually pleasant results. In addition, we propose to connect hierarchical layers of a GAN to get multiple images with the same identity at the same time, which enriches the context and helps to better improve the restoration performance. Extensive experiments on both synthetic and real images show that our method outperforms the existing state-of-the-art methods.
更多查看译文
关键词
turbulence,ltt-gan
AI 理解论文
溯源树
样例
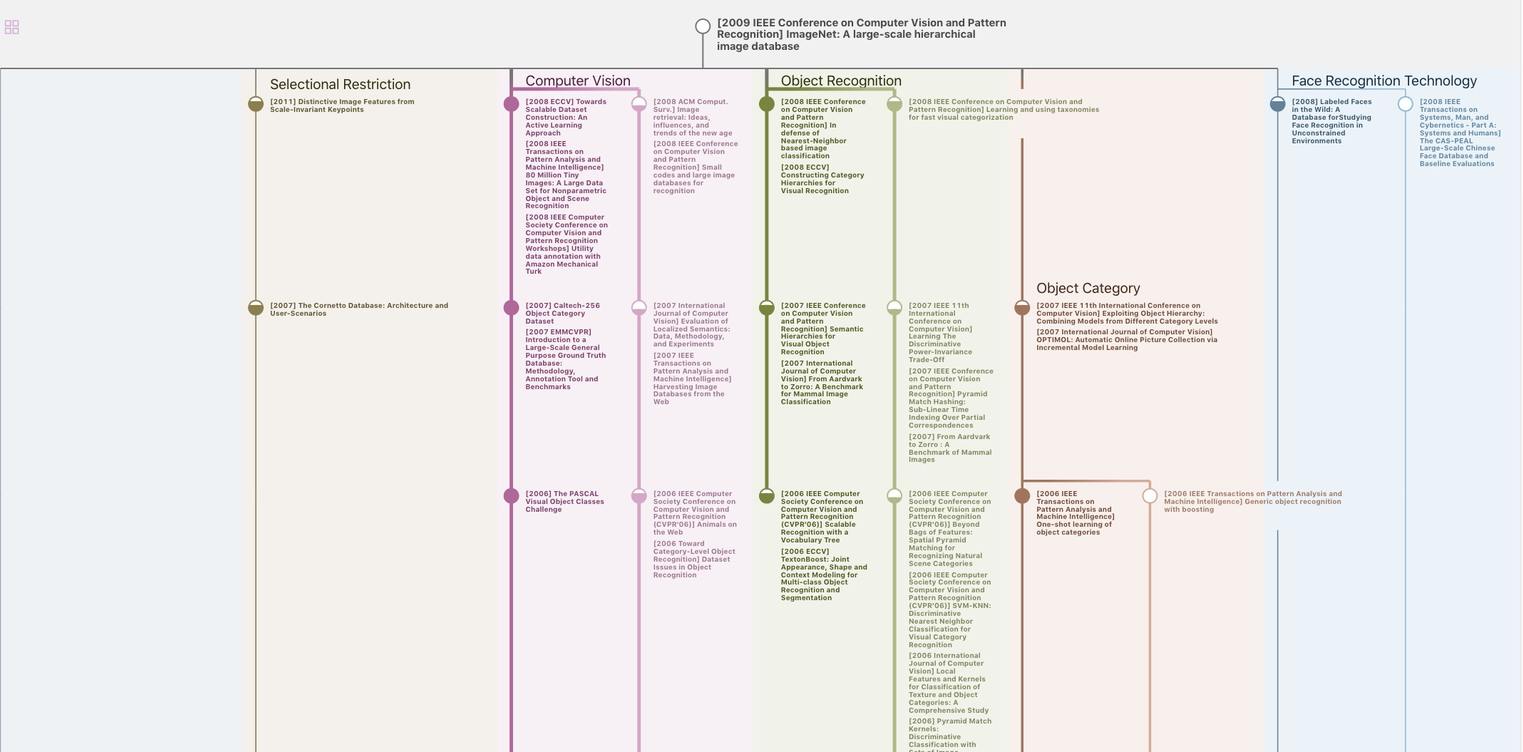
生成溯源树,研究论文发展脉络
Chat Paper
正在生成论文摘要