Point cloud completion by dynamic transformer with adaptive neighbourhood feature fusion
IET COMPUTER VISION(2022)
摘要
Point cloud completion aims to reconstruct detailed structures from partial observations. However, previous methods often suffered from inaccurate neighbourhood feature extraction and rough reconstruction of complex structures, which is difficult to complete detailed semantic shapes. To solve this problem, we present a method that applies dynamic transformers with adaptive neighbourhood feature fusion operations to resume complete point clouds. Firstly, we propose an adaptive neighbourhood feature extraction module, which contains a learnable global neighbourhood selection strategy and a traditional local k-nearest neighbour strategy to dynamically select neighbourhood points according to the shape of different objects. Secondly, we observed that traditional point generation methods based on folding-series operations limit their capacity of generating complex and faithful shapes. Inspired by cell division, we regard the process of reconstructing as point splitting and propose a genetic hierarchical point generation module, which means current points can inherit shape features from previous points and generate more detailed structures of their own. Extensive experiments of point cloud completion are carried out on PCN and Completion3D datasets to verify the effectiveness of our method. The average category chamfer distances of our method are 7.17(x10(-3)) in PCN and 7.96(x10(-4)) in Completion3D, which has better completion performance than other methods.
更多查看译文
AI 理解论文
溯源树
样例
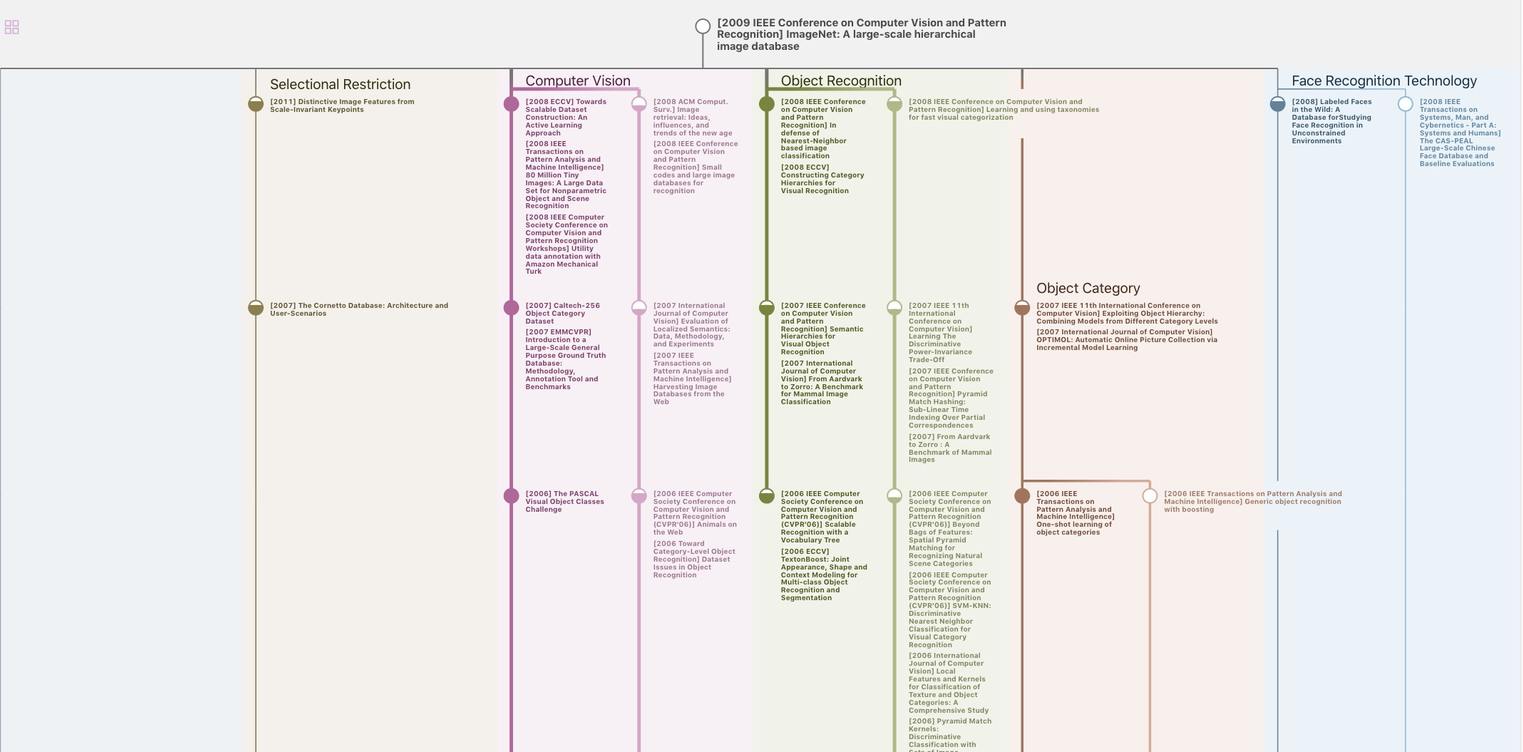
生成溯源树,研究论文发展脉络
Chat Paper
正在生成论文摘要