A Fast and Scalable Polyatomic Frank-Wolfe Algorithm for the LASSO
IEEE SIGNAL PROCESSING LETTERS(2022)
摘要
We propose a fast and scalable polyatomic Frank-Wolfe (P-FW) algorithm for the resolution of high-dimensional LASSO regression problems. This algorithm improves upon traditional Frank-Wolfe methods by considering generalized greedy steps with polyatomic (i.e. linear combinations of multiple atoms) update directions, hence allowing for a more efficient exploration of the search space. To preserve sparsity of the intermediate iterates, we re-optimize the LASSO problem over the set of selected atoms at each iteration. For efficiency reasons, the accuracy of this re-optimization step is relatively low for early iterations and gradually increases with the iteration count. We provide convergence guarantees for our algorithm and validate it in simulated compressed sensing setups. Our experiments reveal that P-FW outperforms state-of-the-art methods in terms of runtime, both for FW methods and optimal first-order proximal gradient methods such as the Fast Iterative Soft-Thresholding Algorithm (FISTA).
更多查看译文
关键词
Signal processing algorithms, Approximation algorithms, Matching pursuit algorithms, Convergence, Optimization, Search problems, Minimization, Conditional gradient, Frank-Wolfe, LASSO, sparse recovery, convex optimisation
AI 理解论文
溯源树
样例
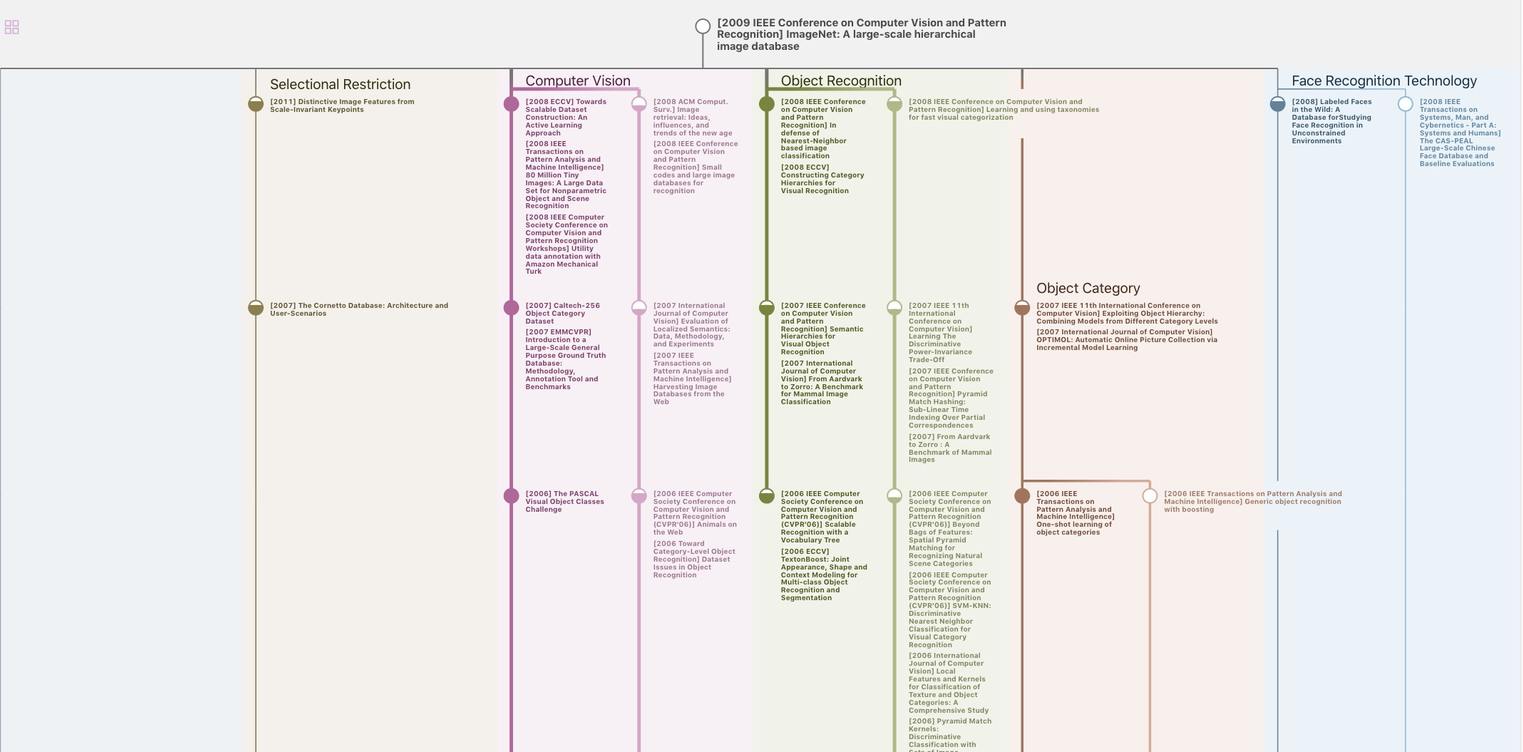
生成溯源树,研究论文发展脉络
Chat Paper
正在生成论文摘要