Curriculum Meta-Learning for Few-shot Classification
arxiv(2021)
摘要
We propose an adaptation of the curriculum training framework, applicable to state-of-the-art meta learning techniques for few-shot classification. Curriculum-based training popularly attempts to mimic human learning by progressively increasing the training complexity to enable incremental concept learning. As the meta-learner's goal is learning how to learn from as few samples as possible, the exact number of those samples (i.e. the size of the support set) arises as a natural proxy of a given task's difficulty. We define a simple yet novel curriculum schedule that begins with a larger support size and progressively reduces it throughout training to eventually match the desired shot-size of the test setup. This proposed method boosts the learning efficiency as well as the generalization capability. Our experiments with the MAML algorithm on two few-shot image classification tasks show significant gains with the curriculum training framework. Ablation studies corroborate the independence of our proposed method from the model architecture as well as the meta-learning hyperparameters
更多查看译文
关键词
curriculum,classification,meta-learning,few-shot
AI 理解论文
溯源树
样例
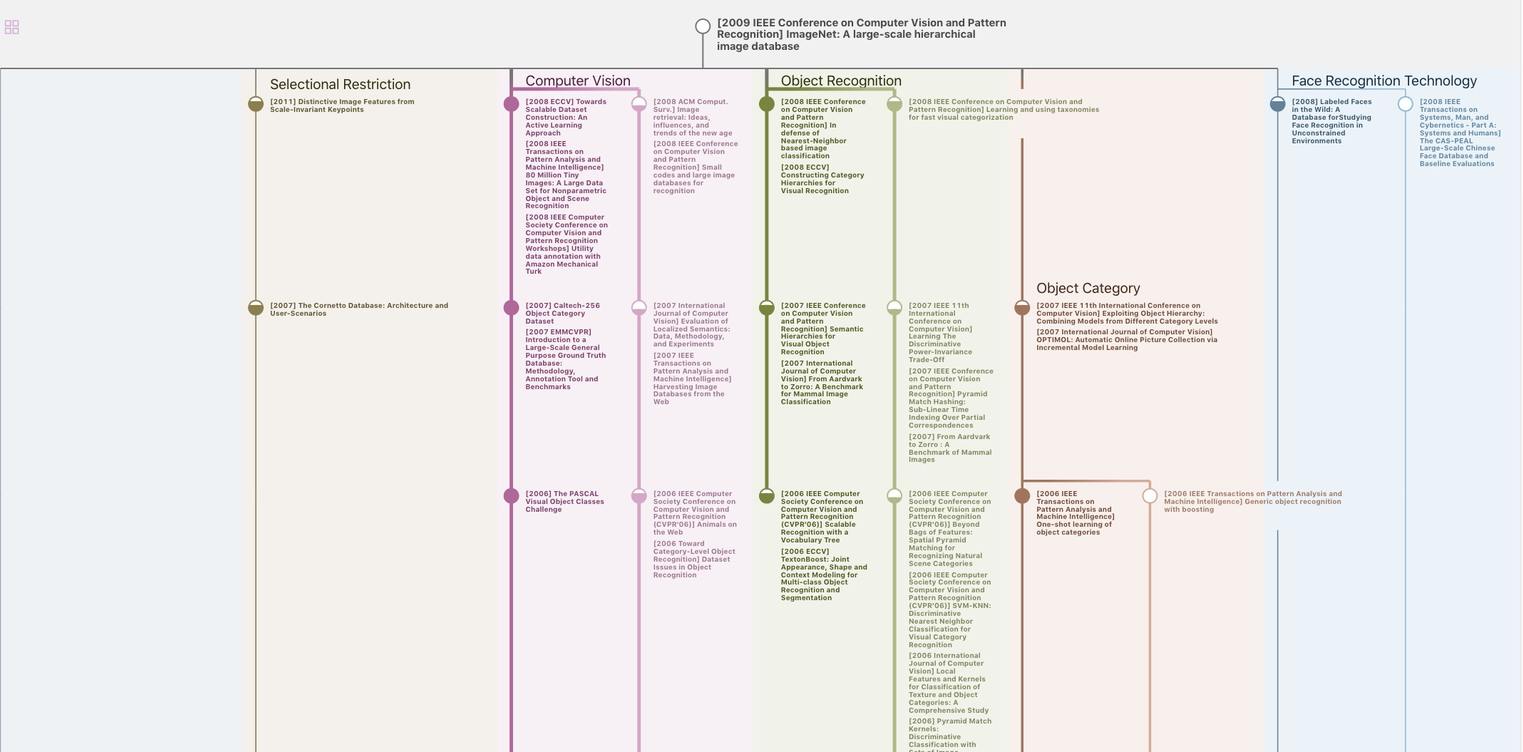
生成溯源树,研究论文发展脉络
Chat Paper
正在生成论文摘要